Deep reinforcement learning based QoE-aware actor-learner architectures for video streaming in IoT environments
Computing(2022)
摘要
The number of connected smart devices enabling multimedia applications has expanded tremendously in Internet-of-Things (IoT) environments. Specifically, the requirement for a high quality of experience (QoE) for video streaming services is a crucial prerequisite for a range of use cases, including smart surveillance, smart healthcare, smart agriculture and many more. However, providing a high QoE for video streaming is challenging due to underlying dynamic network conditions. To address this issue, several adaptive bit rate (ABR) algorithms based on predetermined rules have been developed. However, they do not generalize well to a wide variety of network conditions. ABR algorithms, based on reinforcement learning (RL), have been proven to be more effective at generalizing to varying network conditions but they still have limitations, specifically, constrained exploration and high variance in value estimates. In this paper, we propose asynchronous advantage actor-critic (A3C) based actor-learner architectures for generating the adaptive bit rates for video streaming in IoT environments. To address the existing issues, we propose integrating two advanced A3C algorithms: Follow then Forage Exploration (FFE) and Averaged A3C. We demonstrate their efficacy in improving the QoE over vanilla A3C. Additionally, we also demonstrate the benefits of the proposed architecture for video streaming under different network conditions and for different variants of the QoE metric. We show that advanced A3C methods provide up to 30.70% improvement in QoE over vanilla A3C and a considerably higher QoE over other fixed-rule-based ABR algorithms.
更多查看译文
关键词
Deep reinforcement learning, Internet of things (IoT), Quality of experience (QoE), Adaptive bit rates (ABR), Video streaming, 68, 60, 93
AI 理解论文
溯源树
样例
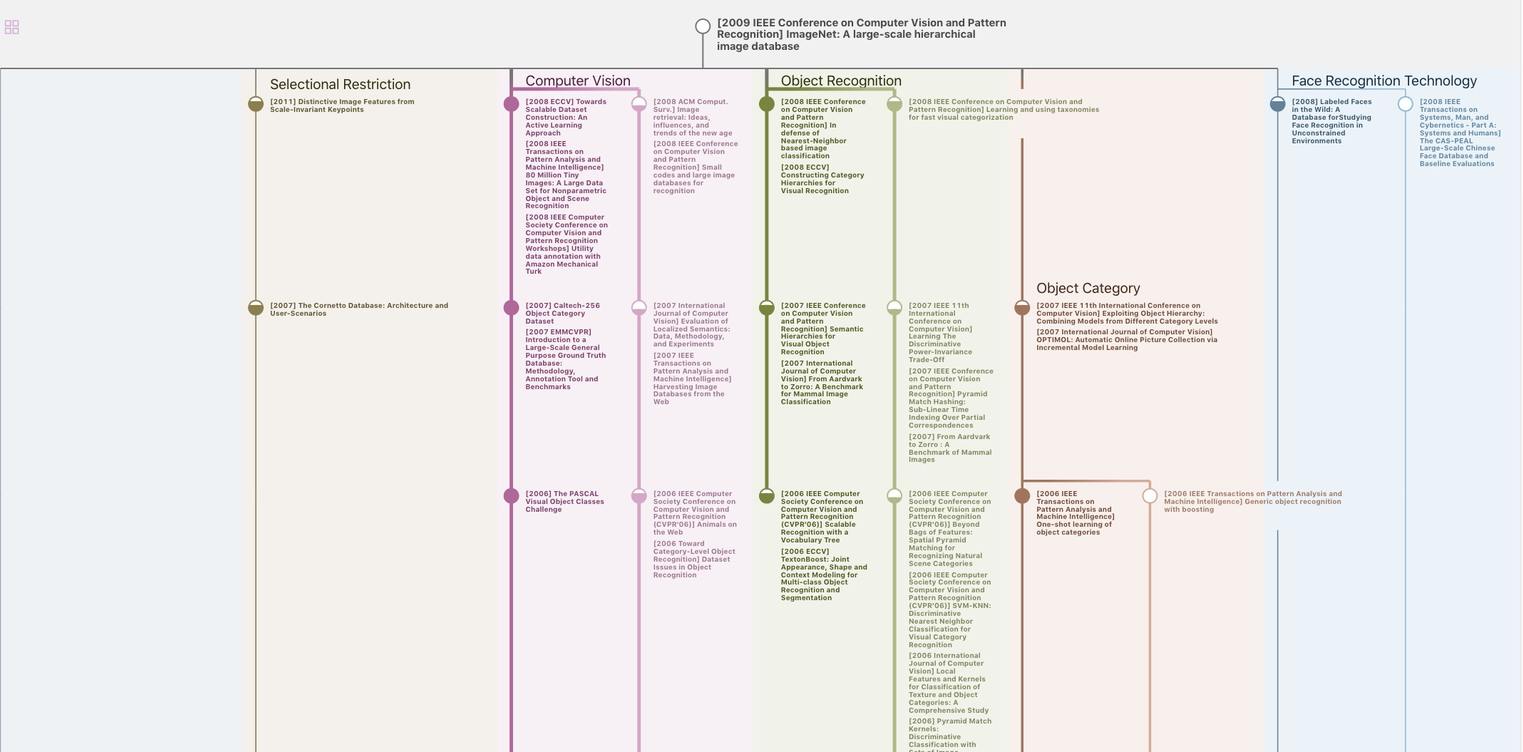
生成溯源树,研究论文发展脉络
Chat Paper
正在生成论文摘要