Double Q-learning-based Energy Management Strategy for Overall Energy Consumption Optimization of Fuel Cell/Battery Vehicle
2021 IEEE Transportation Electrification Conference & Expo (ITEC)(2021)
摘要
Nowadays, most energy management strategies (EMSs) of hybrid systems are developed based on optimization theories. However, the EMS with global optimization capabilities often relies on prior knowledge of operation condition, which is often difficult in practice. Furthermore, with the increasing promotion of machine learning technology, reinforcement learning has been introduced into the research field of hybrid system EMS. Algorithms such as Q-learning and Deep Q Network have been extensively studied. However, the above algorithms have inherent defects of overestimation problem, which will lead to the problems of instability and poor performance. In order to overcome the above problems and save the overall energy consumptions, this paper proposes to adopt Double Q-learning algorithm to design the EMS of a fuel cell hybrid system. Through simulation experiments, the effectiveness of the proposed strategy is verified.
更多查看译文
关键词
fuel cell,reinforcement learning,double Q-leaning,energy management strategy
AI 理解论文
溯源树
样例
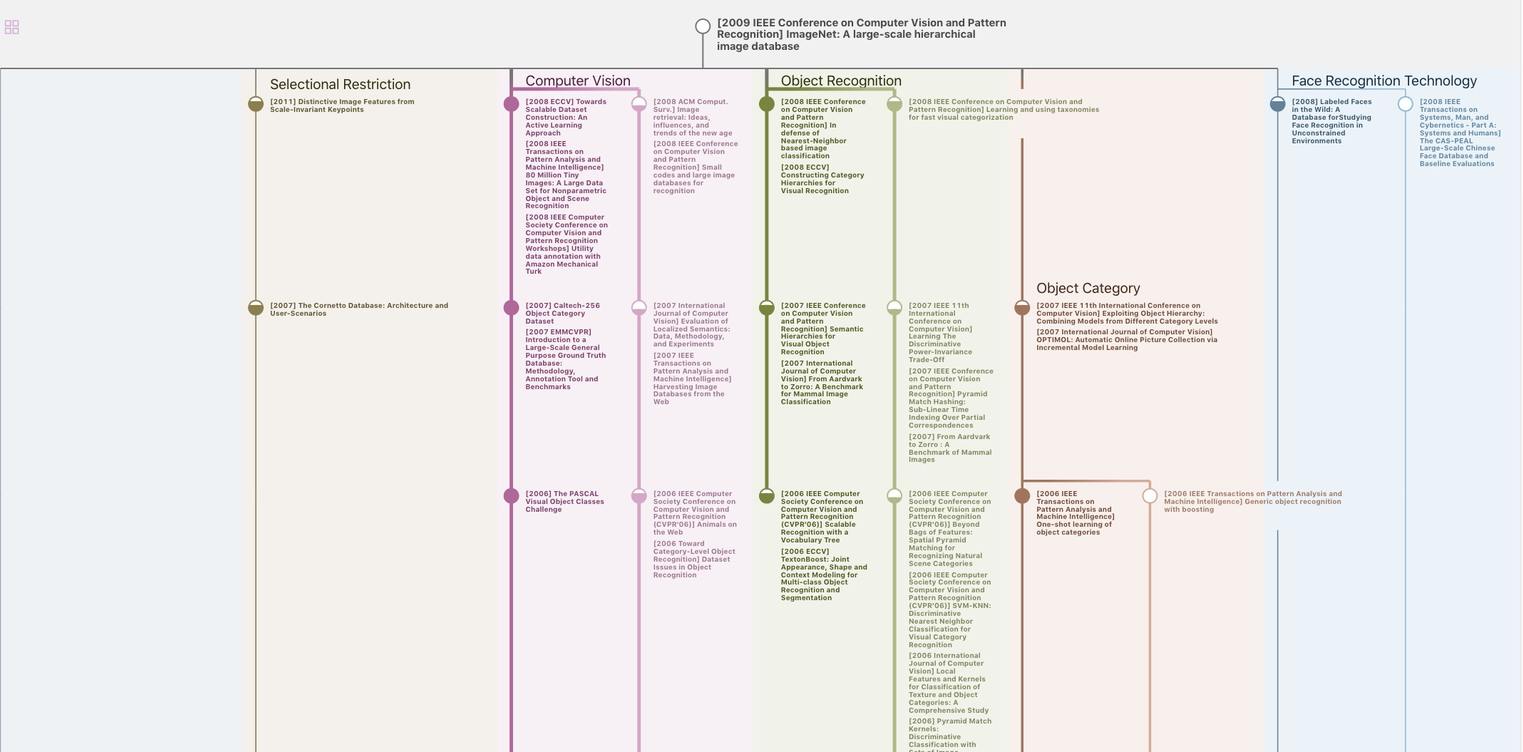
生成溯源树,研究论文发展脉络
Chat Paper
正在生成论文摘要