An investigation of the relationship between numerical precision and performance of Q-learning for hardware implementation
IEICE NONLINEAR THEORY AND ITS APPLICATIONS(2022)
摘要
Reinforcement learning is promising as a machine learning paradigm in edge computing. However, its high computational cost poses a challenge when implementing in devices with limited circuit resources and power consumption. In this study, we investigated the relationship between the bit-length of floating-point operations and the learning performance of the reinforcement learning algorithm. In the case of the FrozenLake maze problem, we found that the learning performance of 8-bit floating-point arithmetic decreased, while that of 16-bit floating-point arithmetic was comparable to that of 64-bit CPU arithmetic. Our results provide a practical guideline for designing a dedicated reinforcement learning hardware with minimum circuit resources and power consumption.
更多查看译文
关键词
reinforcement learning, FPGA, Q-learning, edge computing
AI 理解论文
溯源树
样例
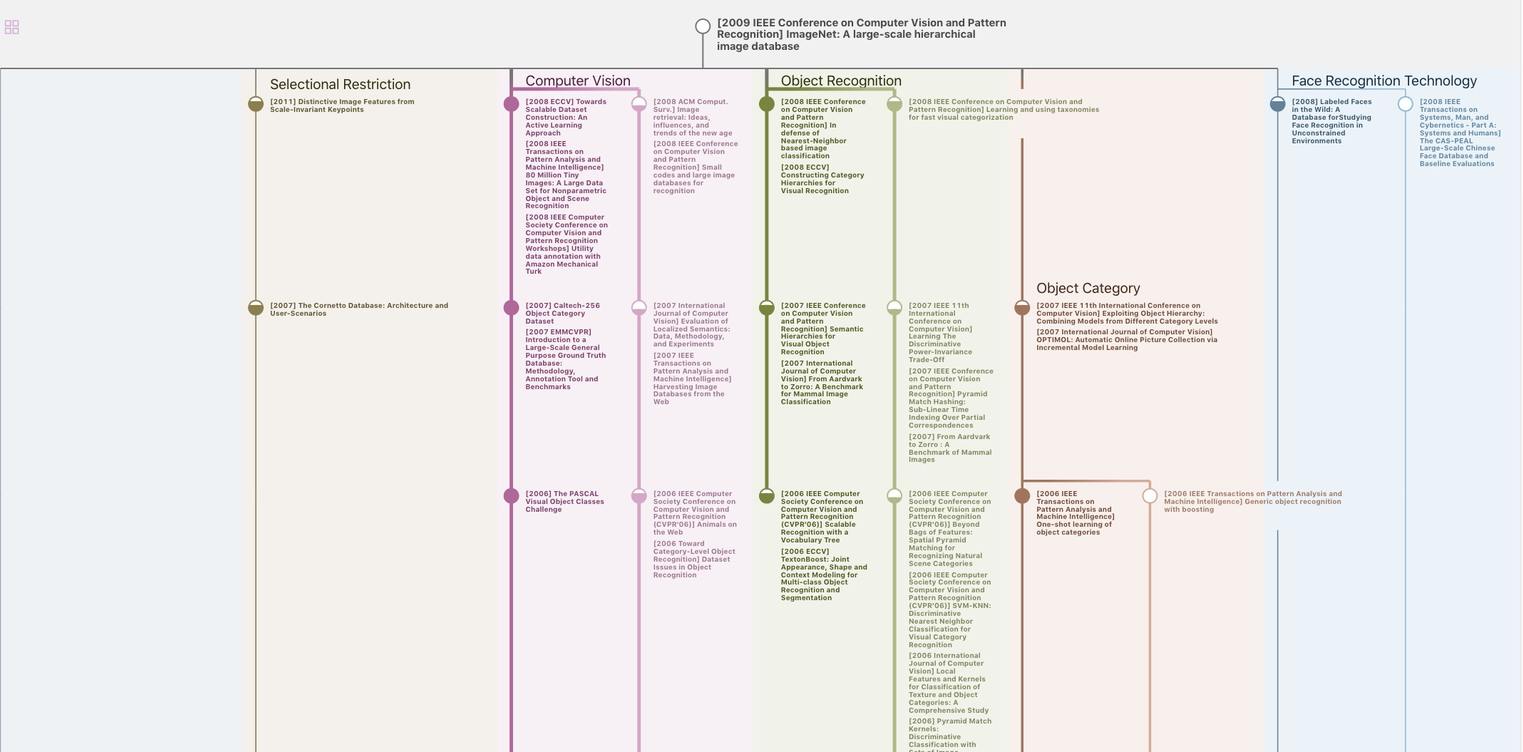
生成溯源树,研究论文发展脉络
Chat Paper
正在生成论文摘要