Individual Emotion Recognition Approach Combined Gated Recurrent Unit With Emoticon Distribution Model
IEEE ACCESS(2021)
Abstract
Mining individual emotions from the data is extremely challenging work in many fields, such as opinion monitoring, business decisions, and information prediction. In previous studies, many studies recognized texts containing positive, negative and neutral sentiments, but few studies focus on emotions according to multiple categories, such as happiness, sadness, anger, fear, disgust and surprise. In this paper, we propose an individual emotion-identifying model called semantic emoticon emotion recognition (SEER). First, we divide the input short text into four categories by using the emotion dictionary and emoticons. Second, we combine a bidirectional gated recurrent unit (Bi-GRU) network with an attention mechanism to capture the emotion vectors of input text from the word aspect. Third, we construct an emoticon distribution model to obtain the emotion vectors in a large quantity of social network data. Fourth, according to different types of input short texts, we select different fusion weights to fuse the emoticon emotion features in text and the semantic emotion features of the texts. Finally, we classify the short text emotions into six categories depending on the final emotion vector. The experimental results show that the SEER is an effective method for improving the accuracy of emotion recognition. In addition, our proposed approach achieves the highest accuracy of 86.35% in emotion recognition, which indicates that the accuracy of our proposed approach achieves improvements of 12.66%, 14.56%, 5.38%, 4.48%, 4.9%, 2.68%, and 3.17% compared to SVM, WL, LSTM, BiLSTM, GRU, Bi-GRU, and CNN+BiLSTM, respectively.
MoreTranslated text
Key words
Emotion recognition,Social networking (online),Semantics,Logic gates,Dictionaries,Deep learning,Text recognition,Emotion recognition,bidirectional gated recurrent unit,attention mechanism,emotion distribution model
AI Read Science
Must-Reading Tree
Example
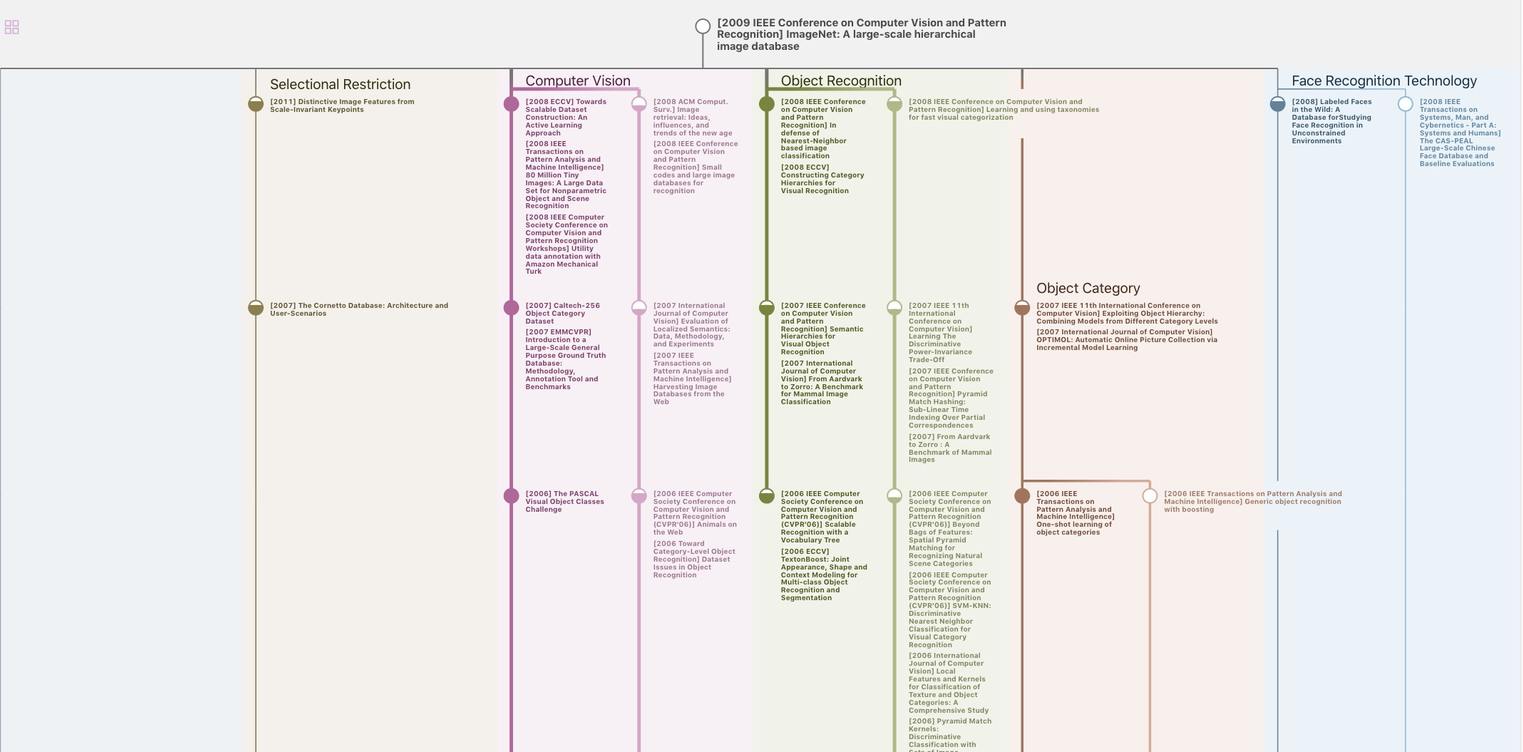
Generate MRT to find the research sequence of this paper
Chat Paper
Summary is being generated by the instructions you defined