Deep graph feature learning-based diagnosis approach for rotating machinery using multi-sensor data
JOURNAL OF INTELLIGENT MANUFACTURING(2022)
摘要
It is necessary to monitor and evaluate health state of rotating machinery, which directly affects the quality and productivity of manufacturing processes. At present, most of the existing fault diagnosis methods focus on analyzing the single sensor data. There are several problems: (1) single sensor data which only contain partial fault information are used to construct the graph, limiting the diagnosis performance; (2) the extracted traditional features containing shallow fault information have limited application scenarios. Recently, graph feature learning-based diagnosis approach shows its powerful feature learning ability, which can overcome the limitations of shallow feature extraction. In this paper, a deep graph feature learning-based diagnosis approach for rotating machinery using multi-sensor data is proposed. Singular values extracted from samples consisting of multi-sensor vibration signals are regarded as the sample node representation to construct the graph data. On the basis, a graph convolutional network is used to extract high-level features from graph data and achieve feature fusion for fault classification. Effectiveness of the proposed approach is verified on a practical experimental platform considering different working conditions (motor loads), and the results shows that it can perform well even in small training dataset.
更多查看译文
关键词
Multi-sensor fusion, Rotating machinery, Fault diagnosis, Graph theory, Feature extraction
AI 理解论文
溯源树
样例
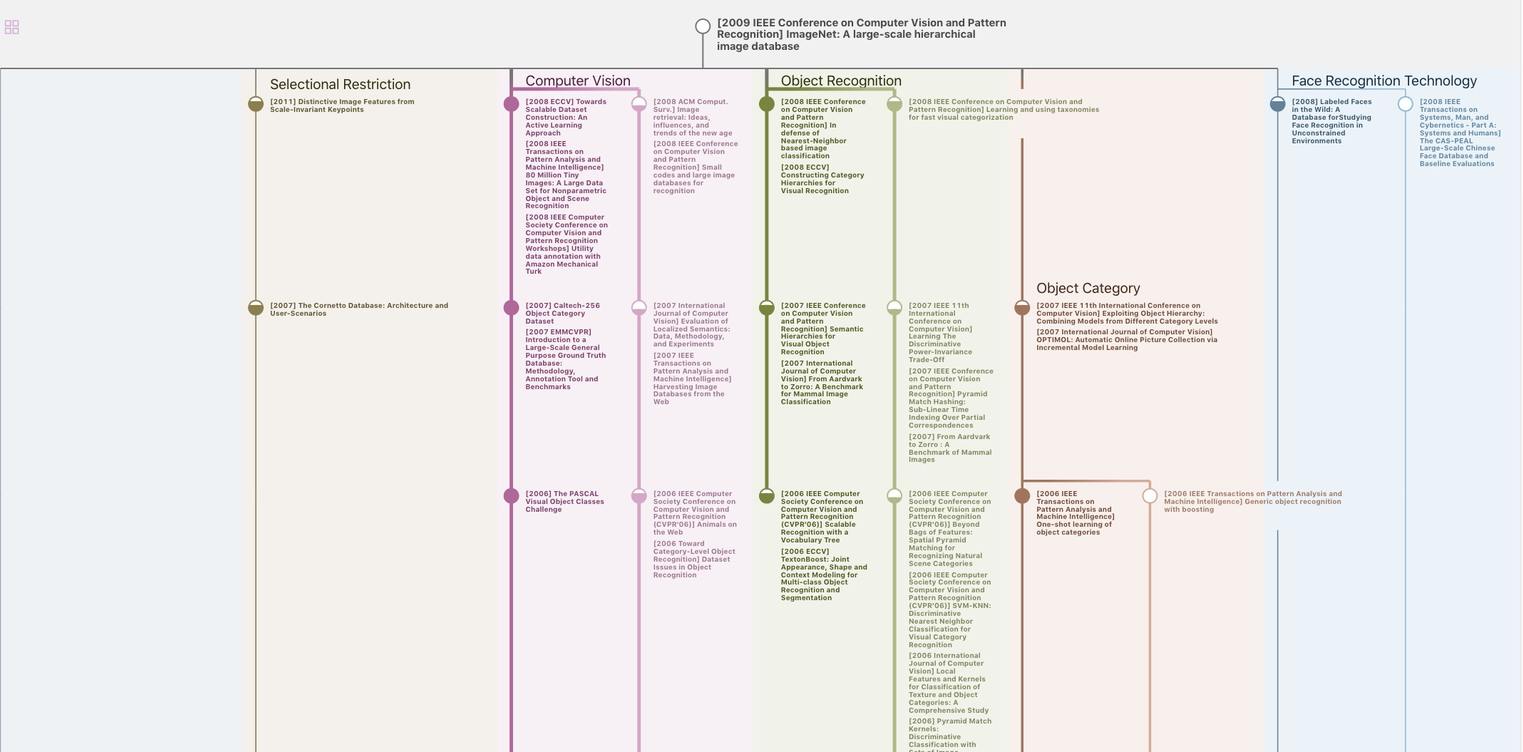
生成溯源树,研究论文发展脉络
Chat Paper
正在生成论文摘要