Modeling and Compensation of IR Drop in Crosspoint Accelerators of Neural Networks
IEEE TRANSACTIONS ON ELECTRON DEVICES(2022)
摘要
In-memory computing (IMC) has the potential of accelerating data-intensive computing tasks, such as inference and training of neural networks, by the matrix vector multiplication (MVM) in the crosspoint array. As the array size increases, however, a significant problem arises due to the parasitic IR drop at row and column lines. Understanding and compensating IR drop is essential to enable high-density accelerators of MVM. This work presents an analytical model for the IR drop and a numerical algorithm capable of accelerating the nodal analysis of crosspoint arrays up to five orders of magnitude with respect to SPICE analysis. The numerical algorithm is used to study the impact of array size, data density, device/wire resistance, and nonlinearity on the IR drop and the related loss of accuracy in IMC. We also derive two simple compensation schemes and an architectural solution for the mitigation of IR drop that are able to increase the accuracy of neural networks from 59% up to 96.6%, compared to an ideal accuracy of 97%.
更多查看译文
关键词
Voltage, Neural networks, Wires, Analytical models, SPICE, Resistance, Estimation, Hardware accelerator, in-memory computing (IMC), neural network, nonvolatile memory, resistive switching memory
AI 理解论文
溯源树
样例
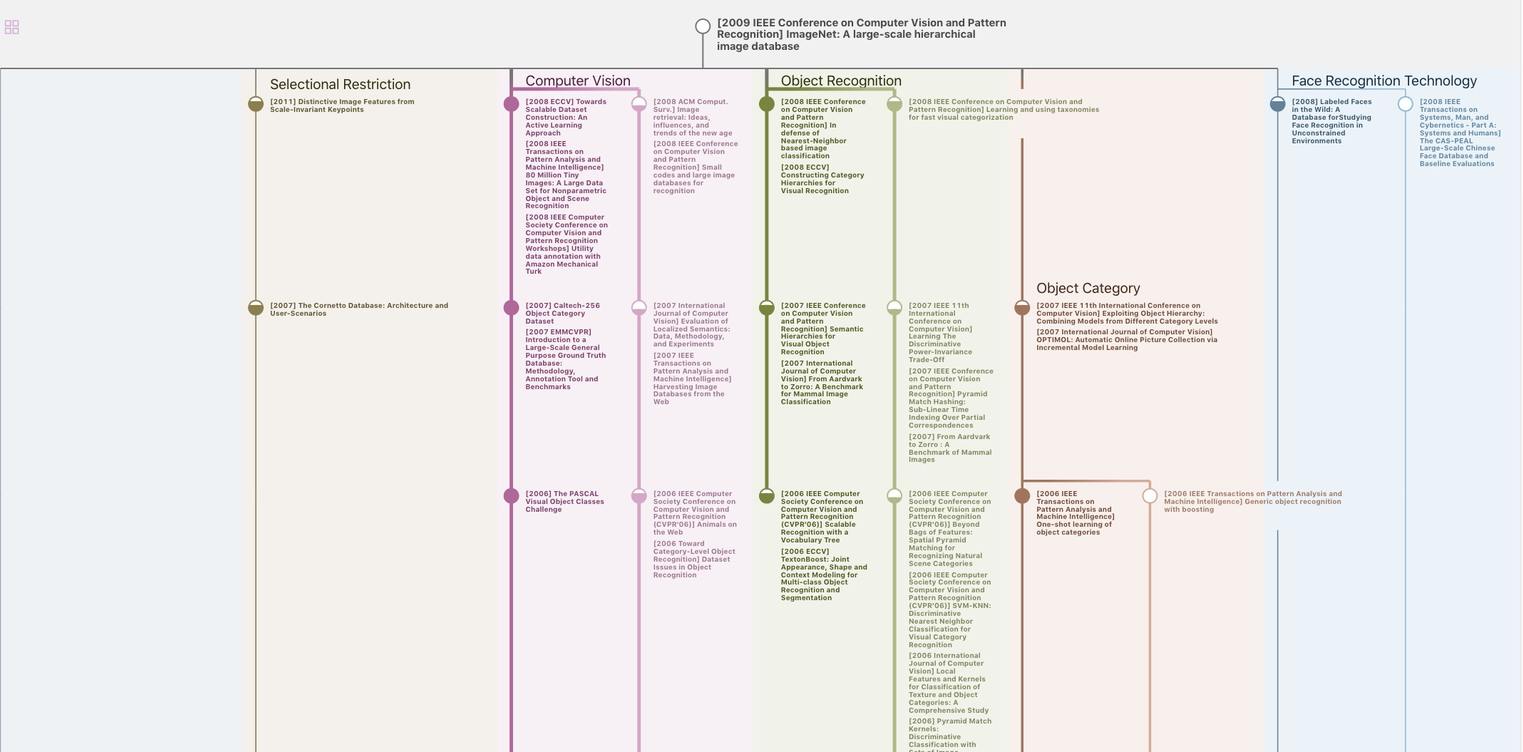
生成溯源树,研究论文发展脉络
Chat Paper
正在生成论文摘要