Robust Discriminant Subspace Clustering With Adaptive Local Structure Embedding
IEEE TRANSACTIONS ON NEURAL NETWORKS AND LEARNING SYSTEMS(2023)
Abstract
Unsupervised dimension reduction and clustering are frequently used as two separate steps to conduct clustering tasks in subspace. However, the two-step clustering methods may not necessarily reflect the cluster structure in the subspace. In addition, the existing subspace clustering methods do not consider the relationship between the low-dimensional representation and local structure in the input space. To address the above issues, we propose a robust discriminant subspace (RDS) clustering model with adaptive local structure embedding. Specifically, unlike the existing methods which incorporate dimension reduction and clustering via regularizer, thereby introducing extra parameters, RDS first integrates them into a unified matrix factorization (MF) model through theoretical proof. Furthermore, a similarity graph is constructed to learn the local structure. A constraint is imposed on the graph to guarantee that it has the same connected components with low-dimensional representation. In this spirit, the similarity graph serves as a tradeoff that adaptively balances the learning process between the low-dimensional space and the original space. Finally, RDS adopts the l $_{2,1}$ -norm to measure the residual error, which enhances the robustness to noise. Using the property of the l $_{2,1}$ -norm, RDS can be optimized efficiently without introducing more penalty terms. Experimental results on real-world benchmark datasets show that RDS can provide more interpretable clustering results and also outperform other state-of-the-art alternatives.
MoreTranslated text
Key words
Principal component analysis, Telecommunications, Clustering methods, Adaptation models, Symmetric matrices, Sparse matrices, Matrix decomposition, Clustering, dimension reduction, discriminant structure, matrix factorization (MF)
AI Read Science
Must-Reading Tree
Example
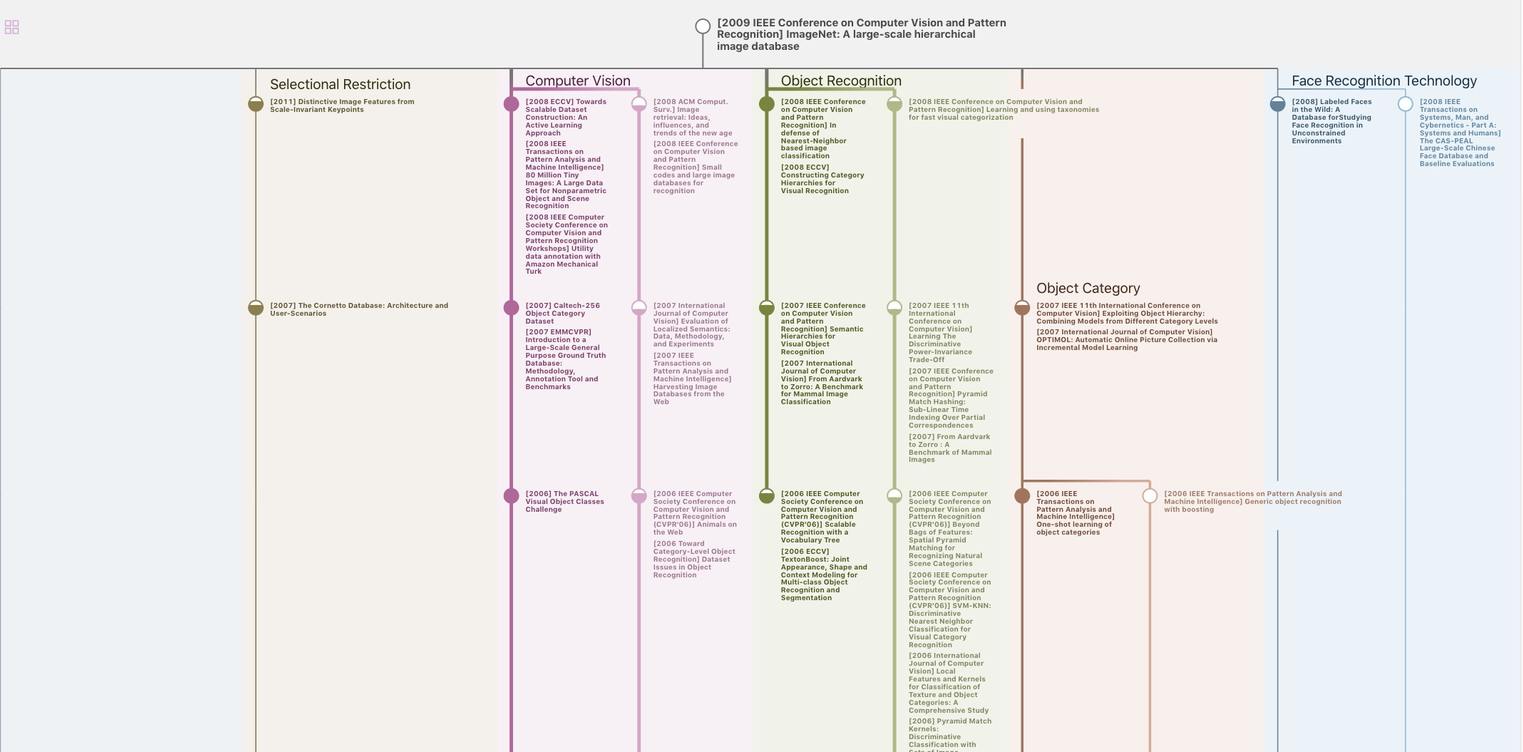
Generate MRT to find the research sequence of this paper
Chat Paper
Summary is being generated by the instructions you defined