Temporal Multiresolution Graph Learning
IEEE ACCESS(2021)
摘要
Estimating time-varying graphs, i.e., a set of graphs in which one graph represents the relationship among nodes in a certain time slot, from observed data is a crucial problem in signal processing, machine learning, and data mining. Although many existing methods only estimate graphs with a single temporal resolution, the actual graphs often demonstrate different relationships in different temporal resolutions. In this study, we propose an approach for time-varying graph learning by leveraging a multiresolution property. The proposed method assumes that time-varying graphs can be decomposed by a linear combination of graphs localized at different temporal resolutions. We formulate a convex optimization problem for temporal multiresolution graph learning. In experiments using synthetic and real data, the proposed method demonstrates the promising objective performances for synthetic data, and obtains reasonable temporal multiresolution graphs from real data.
更多查看译文
关键词
Signal resolution, Laplace equations, Spatial resolution, Optimization, Brain modeling, Mathematical models, Licenses, Graph inference, graph learning, time-varying graph, temporal multiresolution graph
AI 理解论文
溯源树
样例
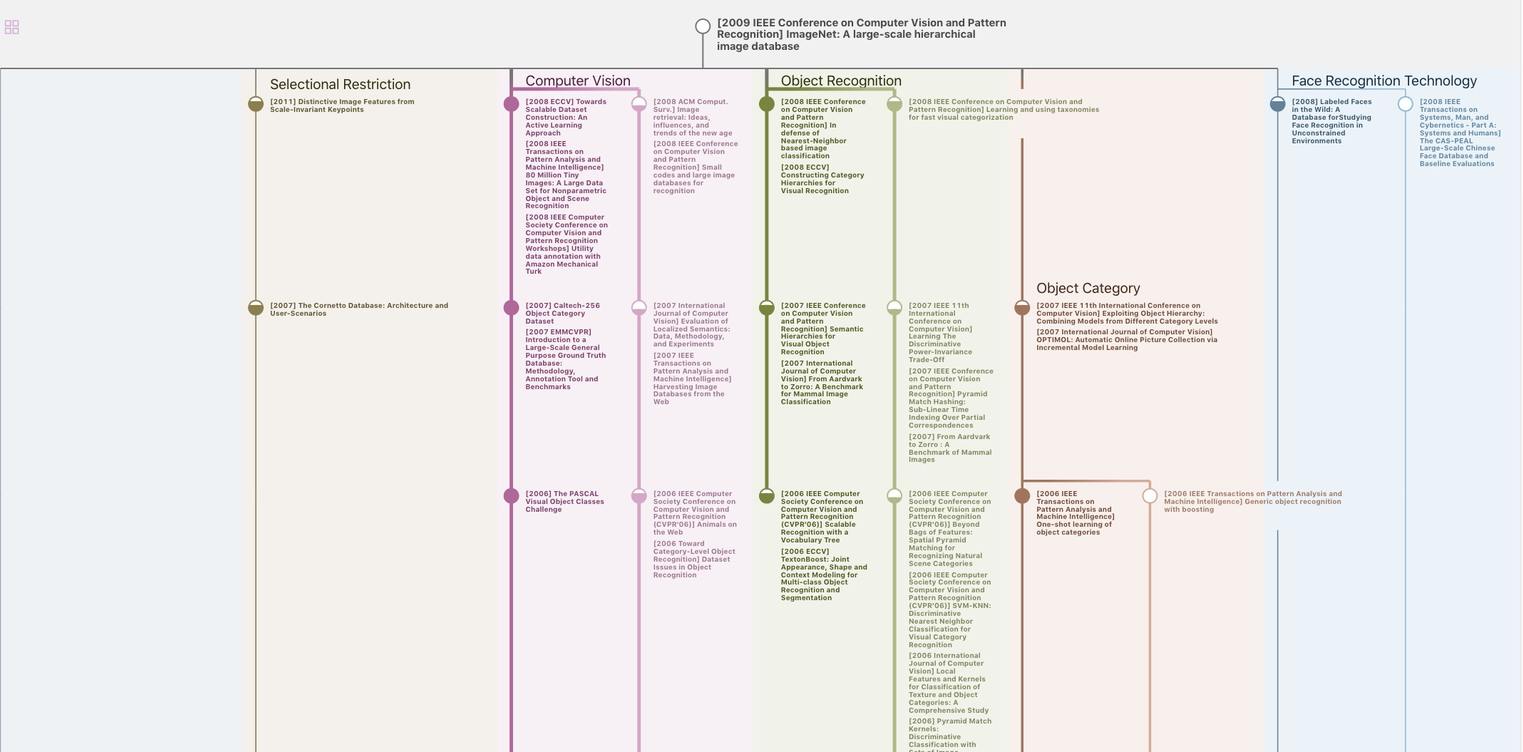
生成溯源树,研究论文发展脉络
Chat Paper
正在生成论文摘要