Neural circuit policies-based temporal flexible soft-sensor modeling of subway PM2.5 with applications on indoor air quality management
BUILDING AND ENVIRONMENT(2022)
摘要
This study developed a data-based soft-sensor to predict indoor PM2.5 from easy-to-measure outdoor and indoor air variables. The method consists of neural circuit policies (NCP), nature-inspired liquid time-constant networks (LTC), a subclass of continuous recurrent neural networks (RNN) represented by an ordinary differential equation (ODE) system to be adapted to each instance. The performance metrics indicated that the NCP yielded the most accurate predictive performance accounting for an improvement compared to other neural methods accounting for 27%-30%. On the other hand, a health risk warning assessment was used to evaluate the NCP capability to detect whether the indoor PM2.5 concentration falls within an 'unhealthy for sensitive groups' health risk level. Finally, the NCP soft-sensor model is evaluated into the ventilation control system of the D subway station, making the comprehensive indoor air quality index (CIAI) stay in a moderate range without any violation of unhealthy breakpoints in contrast to the rule-based ventilation system.
更多查看译文
关键词
Indoor air quality management, Fine particulate matter, Neural circuit policies, Soft-sensor modeling, Subway ventilation system
AI 理解论文
溯源树
样例
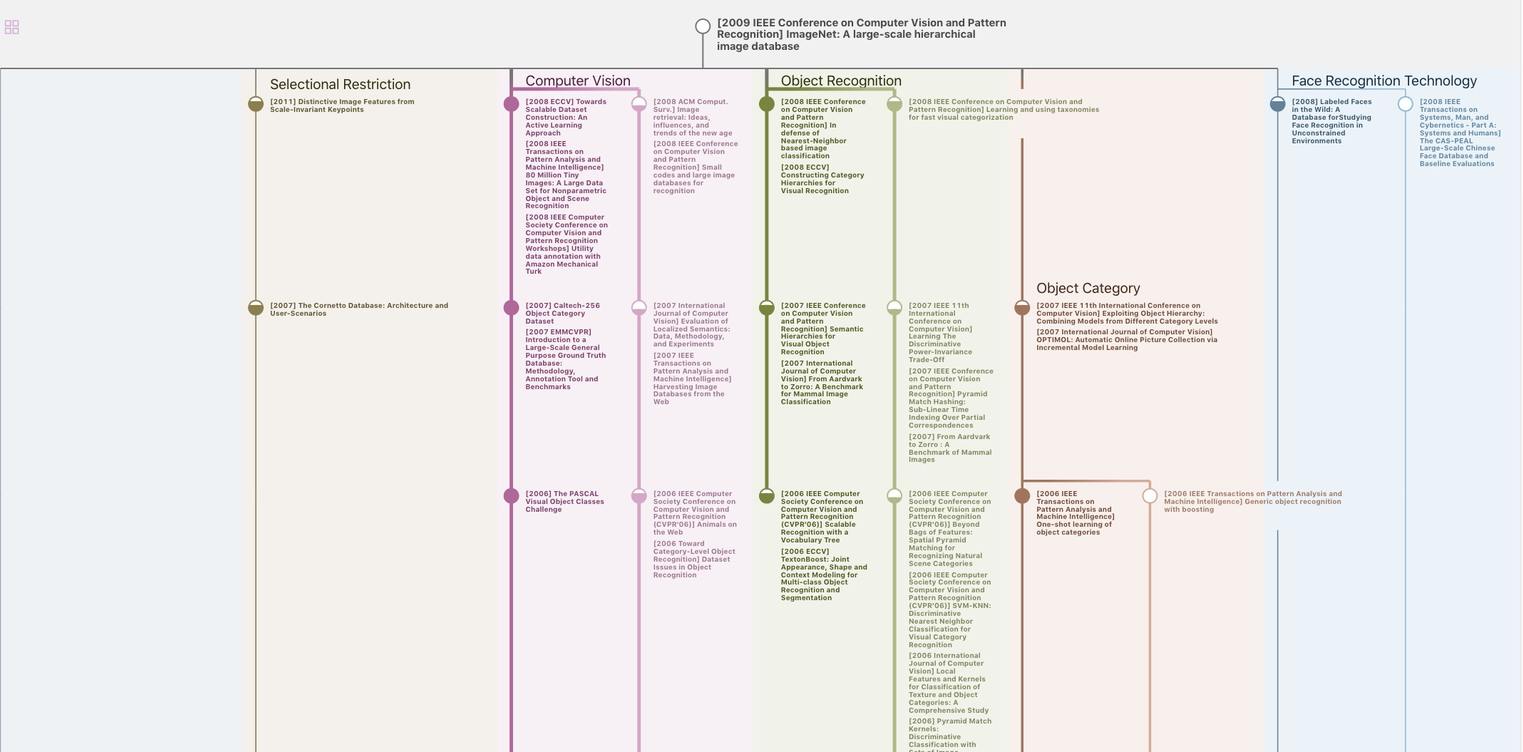
生成溯源树,研究论文发展脉络
Chat Paper
正在生成论文摘要