A Novel Feature Set Extraction Based on Accelerometer Sensor Data for Improving the Fall Detection System
ELECTRONICS(2022)
摘要
Because falls are the second leading cause of injury deaths, especially in the elderly according to WHO statistics, there have been a lot of studies on developing a fall detection and warning system. Many approaches based on wearable sensors, cameras, Infrared sensors, radar, etc., have been proposed to detect falls efficiently. However, it still faces many challenges due to noise and no clear definition of fall activities. This paper proposes a new way to extract 44 features based on the time domain, frequency domain, and Hjorth parameters to deal with this. The effect of the proposed feature set has been evaluated on several classification algorithms, such as SVM, k-NN, ANN, J48, and RF. Our method achieves a relative high performance (F1-Score metric) in detecting fall and non-fall activities, i.e., 95.23% (falls), 99.11% (non-falls), and 96.16% (falls), 99.90% (non-falls) for the MobileAct 2.0 and UP-Fall datasets, respectively.
更多查看译文
关键词
accelerometer, fall detection system (FDS), human activity recognition (HAR), multi-classification, unbalanced data
AI 理解论文
溯源树
样例
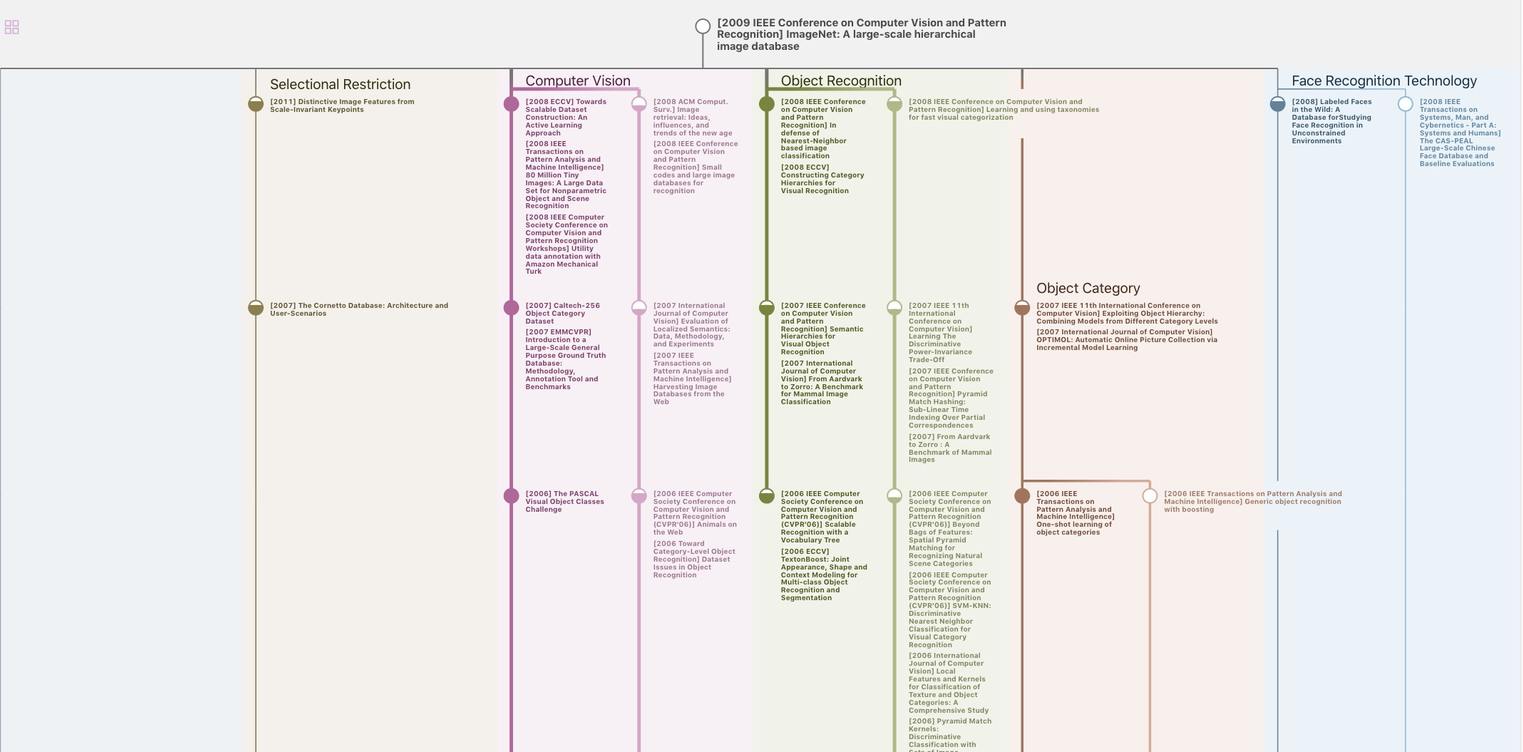
生成溯源树,研究论文发展脉络
Chat Paper
正在生成论文摘要