S-DFP: shifted dynamic fixed point for quantized deep neural network training
NEURAL COMPUTING & APPLICATIONS(2021)
摘要
Recent advances in deep neural networks have achieved higher accuracy with more complex models. Nevertheless, they require much longer training time. To reduce the training time, training methods using quantized weight, activation, and gradient have been proposed. Neural network calculation by integer format improves the energy efficiency of hardware for deep learning models. Therefore, training methods for deep neural networks with fixed point format have been proposed. However, the narrow data representation range of the fixed point format degrades neural network accuracy. In this work, we propose a new fixed point format named shifted dynamic fixed point (S-DFP) to prevent accuracy degradation in quantized neural networks training. S-DFP can change the data representation range of dynamic fixed point format by adding bias to the exponent. We evaluated the effectiveness of S-DFP for quantized neural network training on the ImageNet task using ResNet-34, ResNet-50, ResNet-101 and ResNet-152. For example, the accuracy of quantized ResNet-152 is improved from 76.6% with conventional 8-bit DFP to 77.6% with 8-bit S-DFP.
更多查看译文
关键词
Neural networks,Quantization,Training
AI 理解论文
溯源树
样例
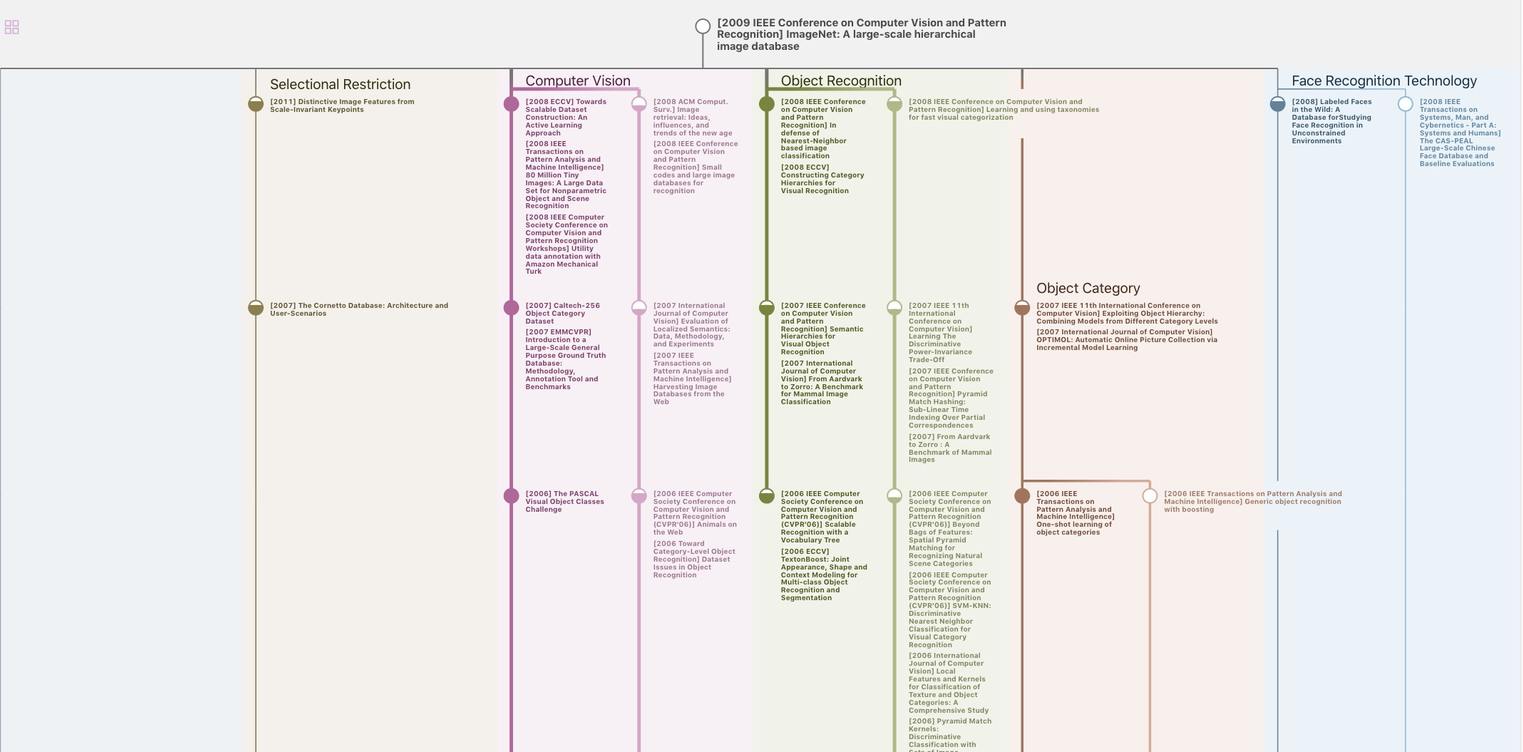
生成溯源树,研究论文发展脉络
Chat Paper
正在生成论文摘要