GRHAM: Towards Group Recommendation Using Hierarchical Attention Mechanism
WEB AND BIG DATA, APWEB-WAIM 2021, PT II(2021)
摘要
Group recommendations extend individual user recommendations to groups, which have become one of the most prevalent topics in the recommender system and widely applied in catering, tourism, movies, and many other fields. The key to group recommendation is how to aggregate the preferences of different group members and calculate the group's preferences. However, the existing aggregation strategy is static and simple. First, they ignore that the preferences of members will change over time. Second, they fail to consider that member's influence is different when the group makes decisions on different activities. To this end, this paper proposes a new novel model for Group Recommendation using Hierarchical Attention Mechanism (GRHAM), which can dynamically adjust the weight of members in group decision-making. Our model consists of two layers of attention neural networks, the first attention layer learns the influence weights of members when the group makes the decision, and the second attention layer learns the influence weights between group members. Besides aggregating the preference of group members, we further learn group topic preferences from the historical data. We conduct experiments on two real datasets, and the experimental results show that our model outperforms other group recommendation models.
更多查看译文
关键词
Group recommendation, Recommender system, Attention mechanism, Preferences aggregation
AI 理解论文
溯源树
样例
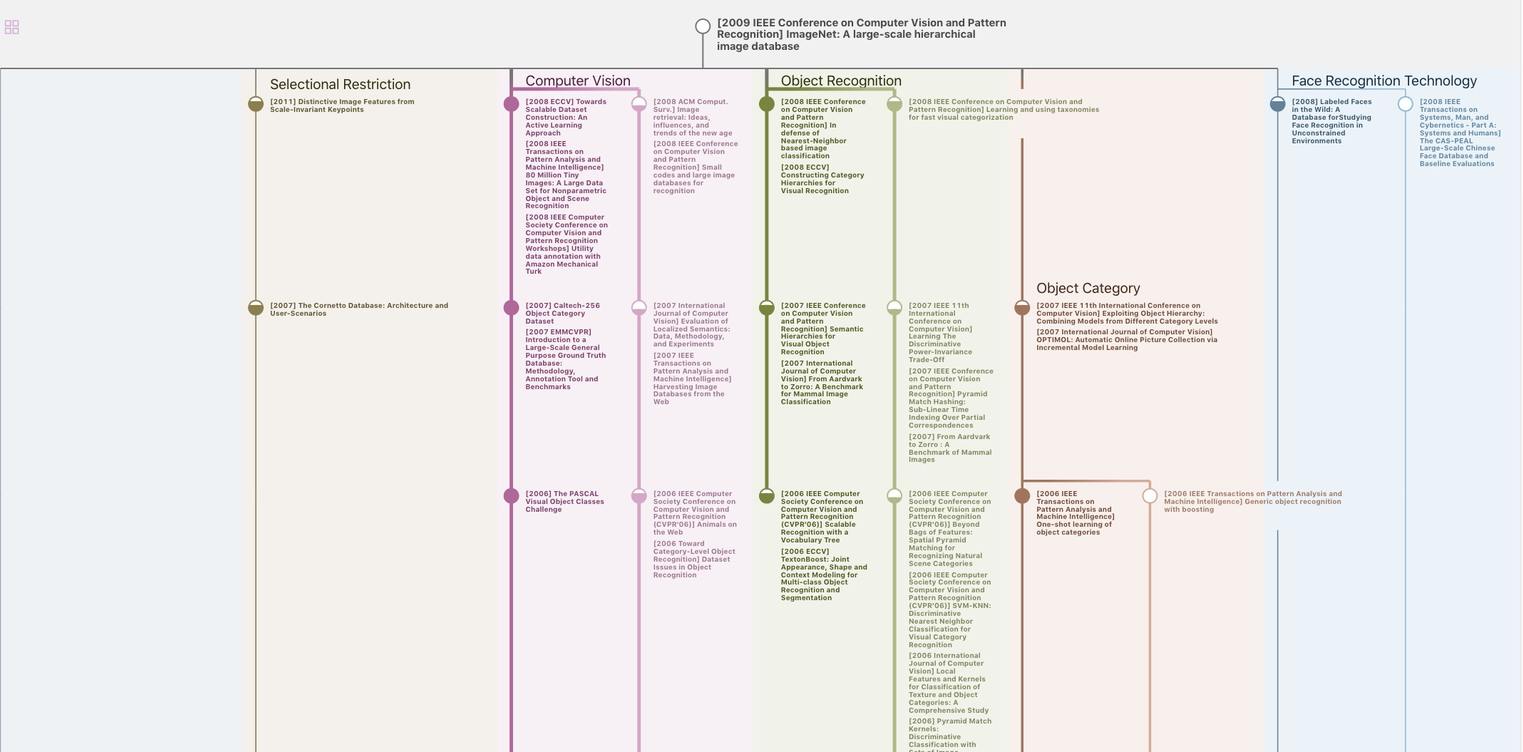
生成溯源树,研究论文发展脉络
Chat Paper
正在生成论文摘要