Adaptive and Global Approaches Based Feature Selection for Large-Scale Hierarchical Text Classification
ADVANCES ON INTELLIGENT INFORMATICS AND COMPUTING: HEALTH INFORMATICS, INTELLIGENT SYSTEMS, DATA SCIENCE AND SMART COMPUTING(2022)
摘要
High-feature dimensions is one of the obvious challenges facing largescale hierarchical classification problem. One promising way to overcome such problem is reducing the features size by removing irrelevant, noise and redundant features. Filter-based feature selection methods are commonly used to construct smaller subset of most relevant features in either adaptive or global approach at each non leaf node within the hierarchy. Both the Feature selection approaches do not only reduce time and space complexity but also improve classification accuracy of the learning models. In this study, the effectiveness of the main approaches for constructing relevant features subset, that is adaptive and global approaches are analyzed using the two filter-based methods suitable for text classification. Experiment evaluation conducted on 20NG, IPC, and DMOZ-small datasets shows that implementing feature selection adaptively has more capability of selecting most relevant features, thus, achieving the best classification accuracy. Moreover, further evaluation shown integrating feature selection with LSHC records improve performance when compared to without feature selection.
更多查看译文
关键词
Feature selection, Filter-based method, Adaptive, Global, Large-scale
AI 理解论文
溯源树
样例
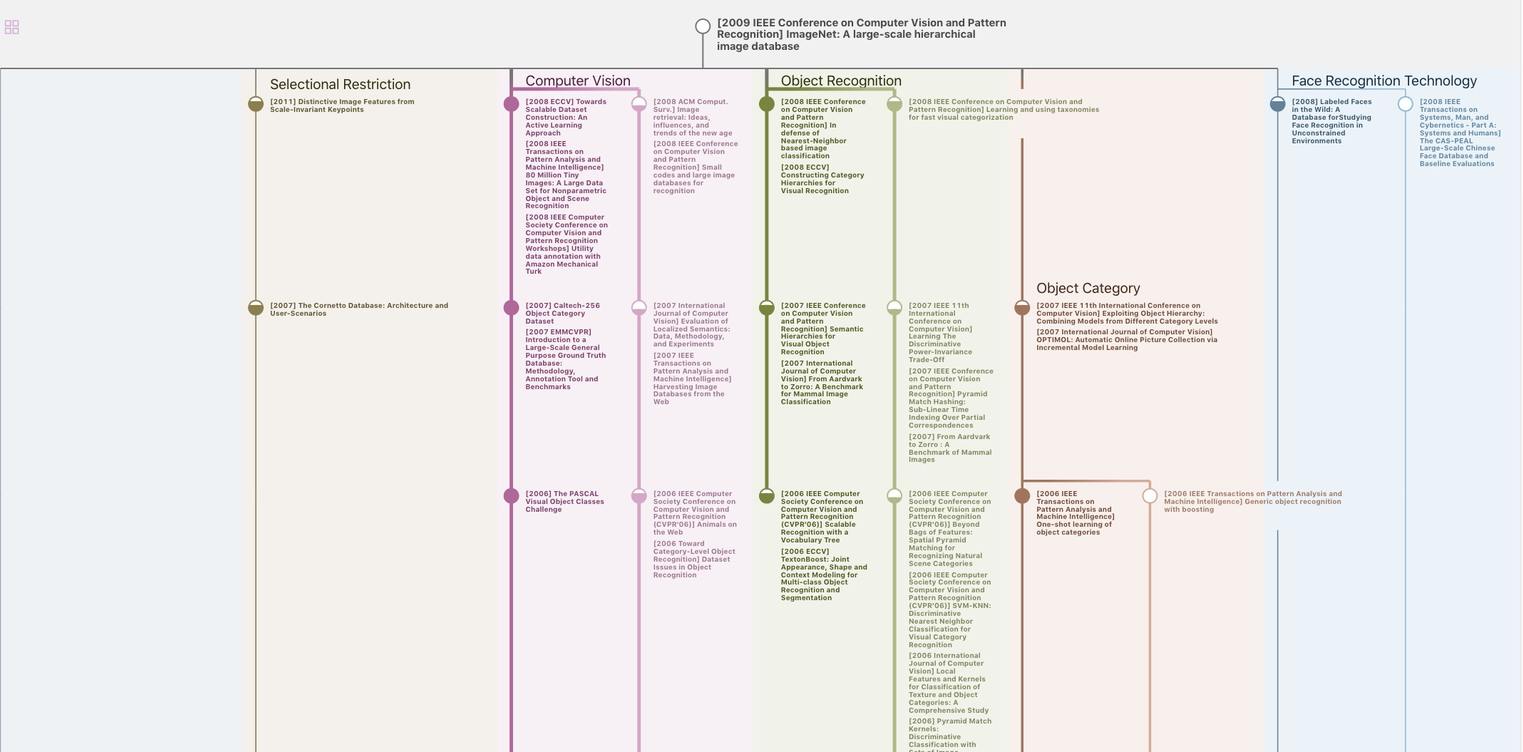
生成溯源树,研究论文发展脉络
Chat Paper
正在生成论文摘要