Linear shrinkage estimation of high-dimensional means
COMMUNICATIONS IN STATISTICS-THEORY AND METHODS(2023)
摘要
In estimation of a mean vector, consider the case that the mean vector is suspected to be in one or two general linear subspaces. Then it is reasonable to shrink a sample mean vector toward the restricted estimators on the linear subspaces. Motivated from a standard Bayesian argument, we propose single and double shrinkage estimators in which their optimal weights are estimated consistently in high dimension without assuming any specific distributions. Asymptotic relative improvement in risk of shrinkage estimators over the sample mean vector is derived in high dimension, and it is shown that the gain in improvement by shrinkage depends on the linear subspace. Finally, the performance of the linear shrinkage estimators is numerically investigated by simulation.
更多查看译文
关键词
High dimension, mean vector, non-normal distribution, quadratic loss function, risk function, shrinkage, Stein estimator
AI 理解论文
溯源树
样例
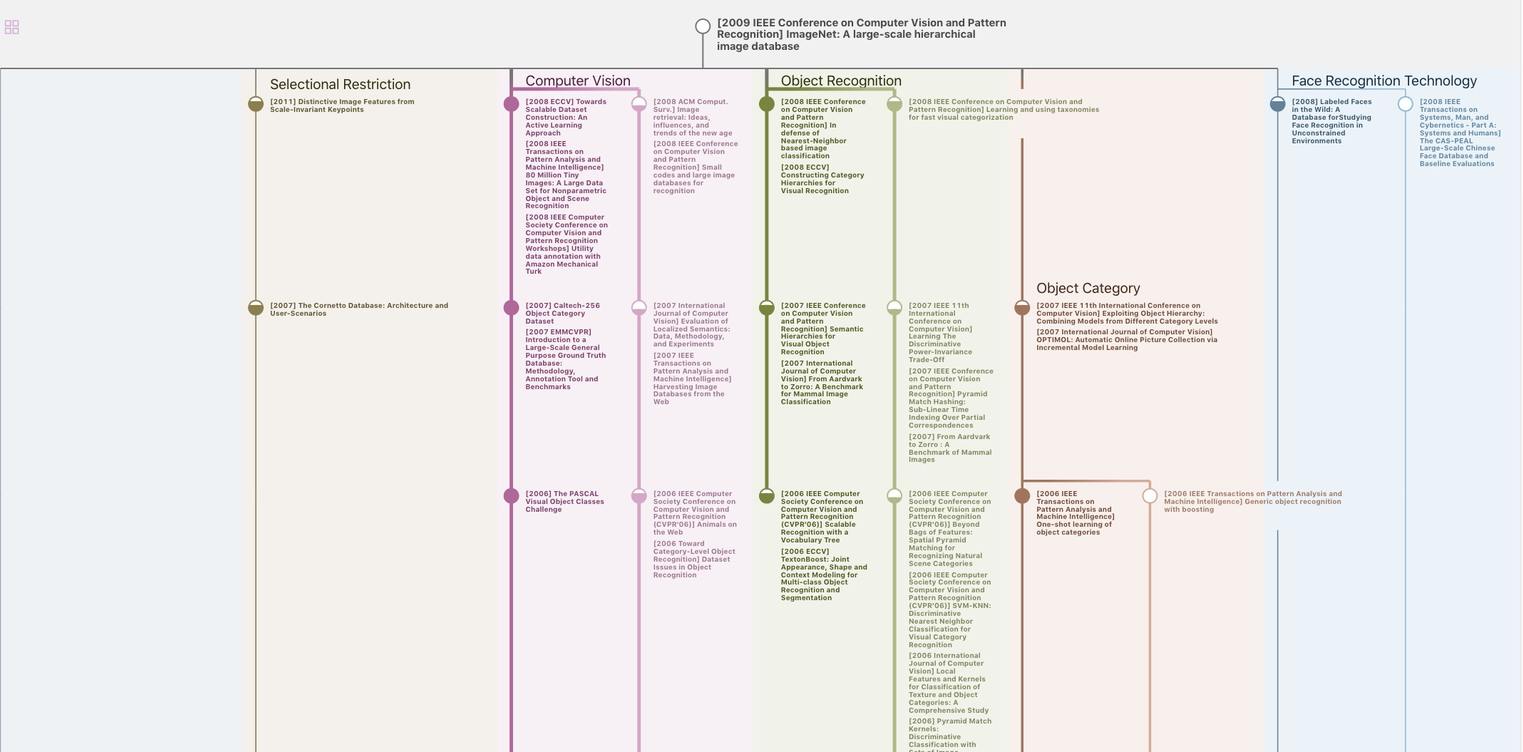
生成溯源树,研究论文发展脉络
Chat Paper
正在生成论文摘要