Label-free and interpretable hyperspectral imaging for intraoperative clinical applications
TWELFTH INTERNATIONAL CONFERENCE ON INFORMATION OPTICS AND PHOTONICS (CIOP 2021)(2021)
摘要
Liver cancer is the fourth leading cause of cancer death in the world, and it is even more serious in China, occupying over 50% of new cases and deaths worldwide. One of the major challenges of cancer surgery remains the complete removal of the tumor. Therefore, numerous studies have proposed to design automatic systems aimed at supporting physicians during the surgery. As an optical imaging modality, hyperspectral imaging (HSI) simultaneously captures spatial and spectral features to bring additional insight to surgeons in a label-free manner. However, to the best of our knowledge, previous effort on medical HSI lacks attention to the interpretability of decisions, which greatly hinders the application of HSI in safety-critical scenarios. Through the intrinsic correlation between tissue composition and its spectrum, hyperspectral images can be interpreted according to different spectral characteristics. Generally, interpretability of spectral images often employs a physical model of light interact with tissues. Such an approach has been long practiced in tissue optics, e.g., diffuse reflectance spectroscopy (DRS). Recent advances in deep learning showed state-of-the-art performance on both detection and segmentation of malignant tissues, breaking through the bottleneck of traditional methods in real-time applications. However, due to the lack of interpretability of the deep learning model, the reliability of the results becomes an issue. In this work, we develop and evaluate a diffusion model-based and a deep learning-based interpretable spectral imaging model, respectively, in the context of liver tumor delineation from clinical hyperspectral image dataset. Notably, both methods point to a key sign of abnormality is the difference in bile volume fraction, which could lay the foundation for the interpretability and system design of the spectral imaging system in practical use.
更多查看译文
关键词
Liver, Cancer, Hyperspectral imaging, Interpretable, Deep learning, Diffusion model
AI 理解论文
溯源树
样例
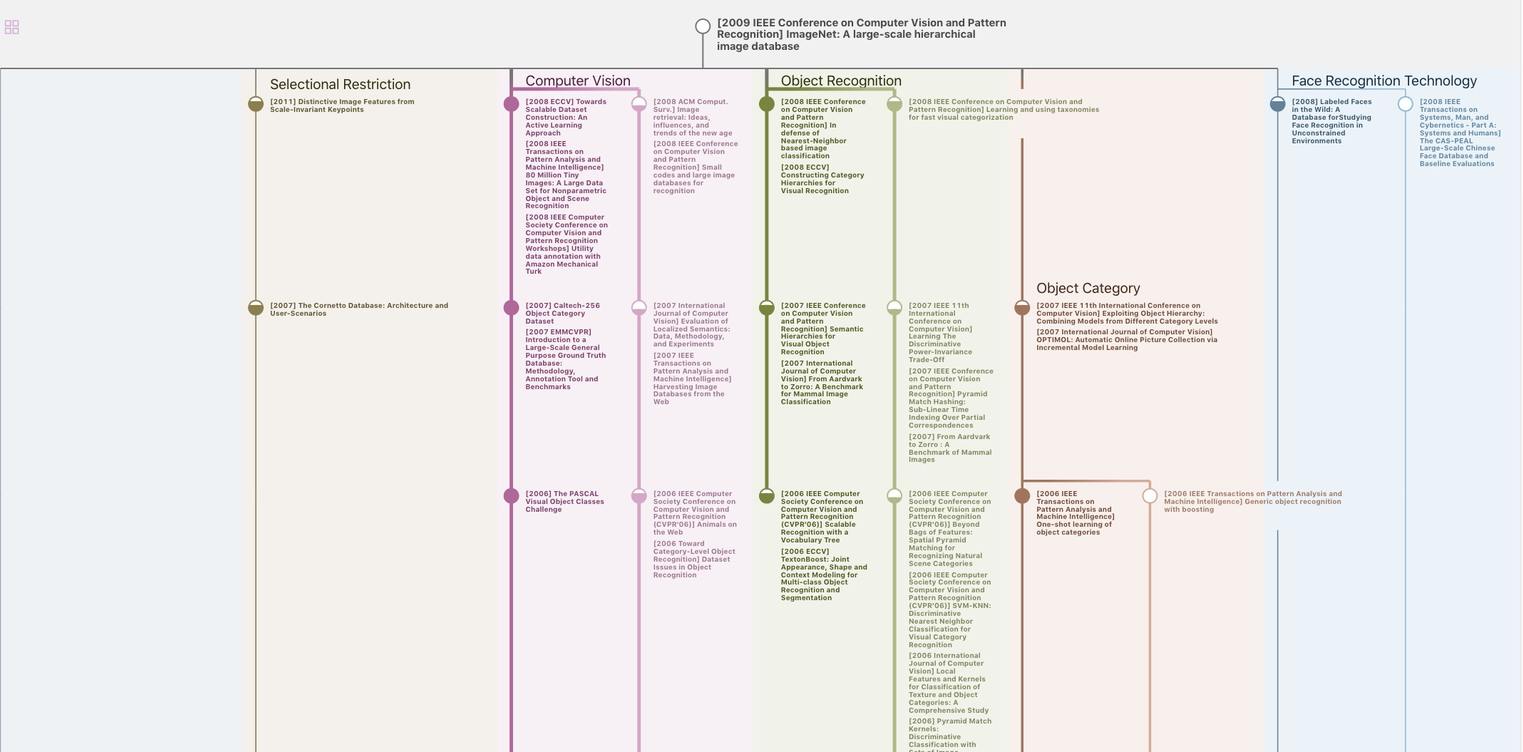
生成溯源树,研究论文发展脉络
Chat Paper
正在生成论文摘要