Hyperspectral Image Classification with Residual Learning Networks
AOPC 2021: OPTICAL SENSING AND IMAGING TECHNOLOGY(2021)
摘要
Hyperspectral imaging is particularly useful for per-pixel thematic classification by unique spectral signatures of landscape materials. Deep learning techniques such as convolutional neural networks have boosted the perfoimance of image classification. Recently, several composite learning-based convolutional networks, i.e., deep residual networks (ResNets) and dense convolutional networks (DenseNets), have been presented to learn deep feature representation for image classification, and achieve high classification accuracies. In this paper, we present a fairly comparable architecture, including two kinds of modified residual learning networks with a shallow depth using small training data. First, we perfoint the extraction of key components from deep residual networks and dense convolutional networks, which is a set of composite learning structures with skip connections. Second, the plain convolutional neural networks (PNets) have been constituted by a stack of plain blocks that also have been placed in the presented network architecture as the baseline networks. Third, we make them as comparable as possible with the plain convolutional network structures, so that the more profound exploration and improvement could be further done. Finally, we wrap them together and design a comparable architecture. Experiments demonstrate that the presented residual learning networks show special characteristics for hyperspectral image classification, which have not been revealed before.
更多查看译文
关键词
Hyperspectral image classification, deep learning, deep residual networks, dense convolutional networks, convolutional neural networks
AI 理解论文
溯源树
样例
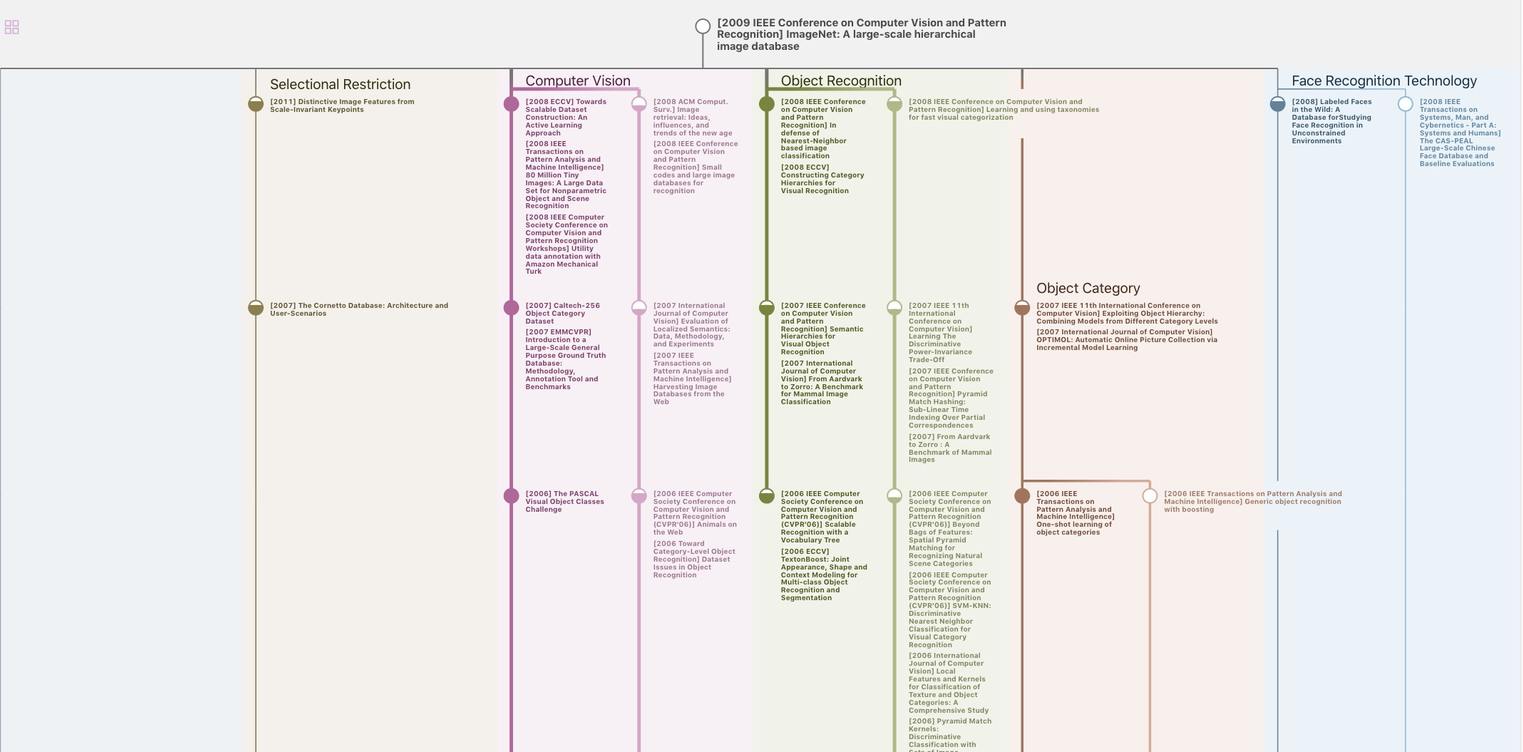
生成溯源树,研究论文发展脉络
Chat Paper
正在生成论文摘要