A Penalized Synthetic Control Estimator for Disaggregated Data
JOURNAL OF THE AMERICAN STATISTICAL ASSOCIATION(2021)
摘要
Synthetic control methods are commonly applied in empirical research to estimate the effects of treatments or interventions on aggregate outcomes. A synthetic control estimator compares the outcome of a treated unit to the outcome of a weighted average of untreated units that best resembles the characteristics of the treated unit before the intervention. When disaggregated data are available, constructing separate synthetic controls for each treated unit may help avoid interpolation biases. However, the problem of finding a synthetic control that best reproduces the characteristics of a treated unit may not have a unique solution. Multiplicity of solutions is a particularly daunting challenge when the data include many treated and untreated units. To address this challenge, we propose a synthetic control estimator that penalizes the pairwise discrepancies between the characteristics of the treated units and the characteristics of the units that contribute to their synthetic controls. The penalization parameter trades off pairwise matching discrepancies with respect to the characteristics of each unit in the synthetic control against matching discrepancies with respect to the characteristics of the synthetic control unit as a whole. We study the properties of this estimator and propose data-driven choices of the penalization parameter.
更多查看译文
关键词
Causal inference, Treatment effects, Observational studies
AI 理解论文
溯源树
样例
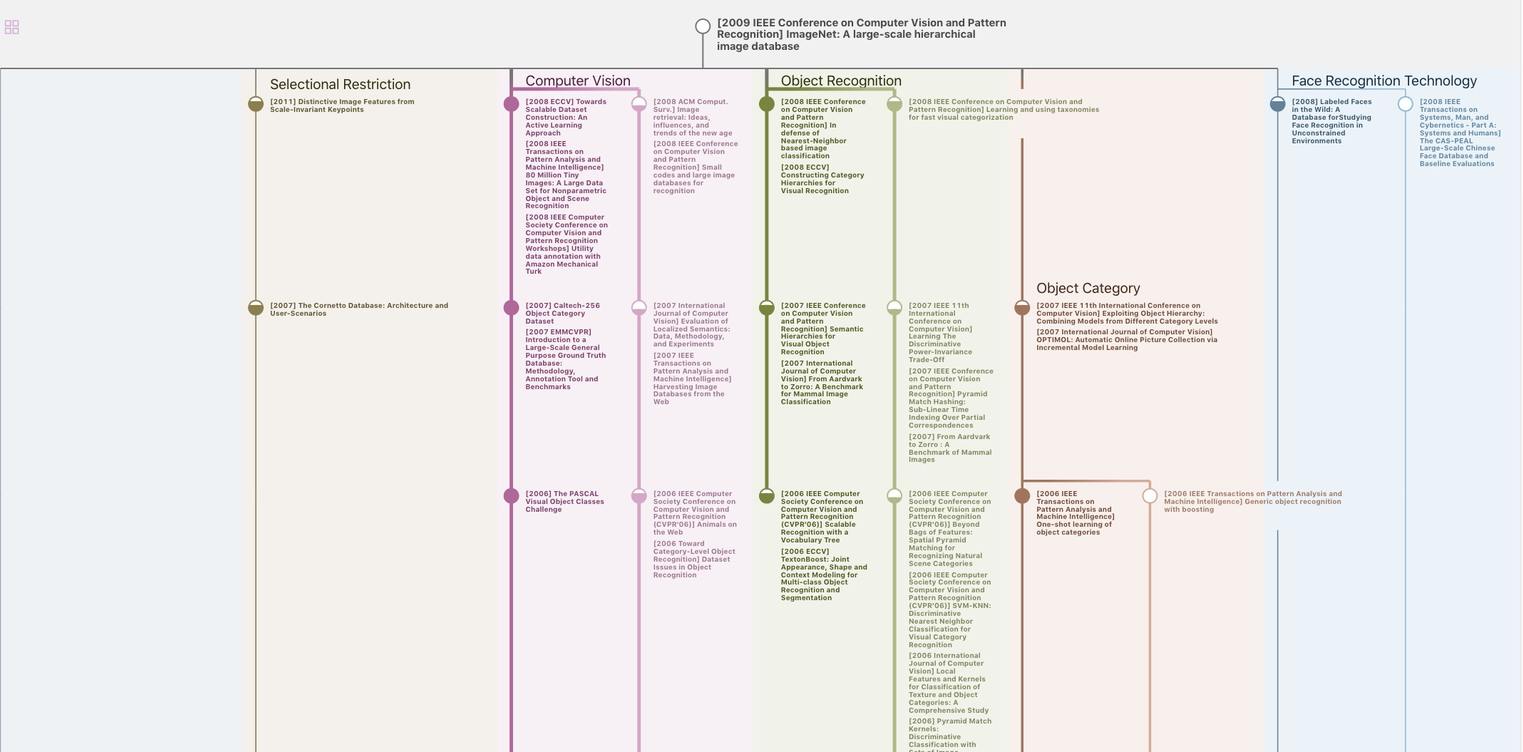
生成溯源树,研究论文发展脉络
Chat Paper
正在生成论文摘要