A Novel Learning Based Non-Lambertian Photometric Stereo Method for Pixel-Level Normal Reconstruction of Polished Surfaces
MACHINES(2022)
摘要
High-quality reconstruction of polished surfaces is a promising yet challenging task in the industrial field. Due to its extreme reflective properties, state-of-the-art methods have not achieved a satisfying trade-off between retaining texture and removing the effects of specular outliers. In this paper, we propose a learning based pixel-level photometric stereo method to estimate the surface normal. A feature fusion convolutional neural network is used to extract the features from the normal map solved by the least square method and from the original images respectively, and combine them to regress the normal map. The proposed network outperforms the state-of-the-art methods on the DiLiGenT benchmark dataset. Meanwhile, we use the polished rail welding surface to verify the generalization of our method. To fit the complex geometry of the rails, we design a flexible photometric stereo information collection hardware with multi-angle lights and multi-view cameras, which can collect the light and shade information of the rail surface for photometric stereo. The experimental results indicate that the proposed method is able to reconstruct the normal of the polished surface at the pixel level with abundant texture information.
更多查看译文
关键词
polished surface, specular reflection, photometric stereo, feature fusion, 3D reconstruction, modeling
AI 理解论文
溯源树
样例
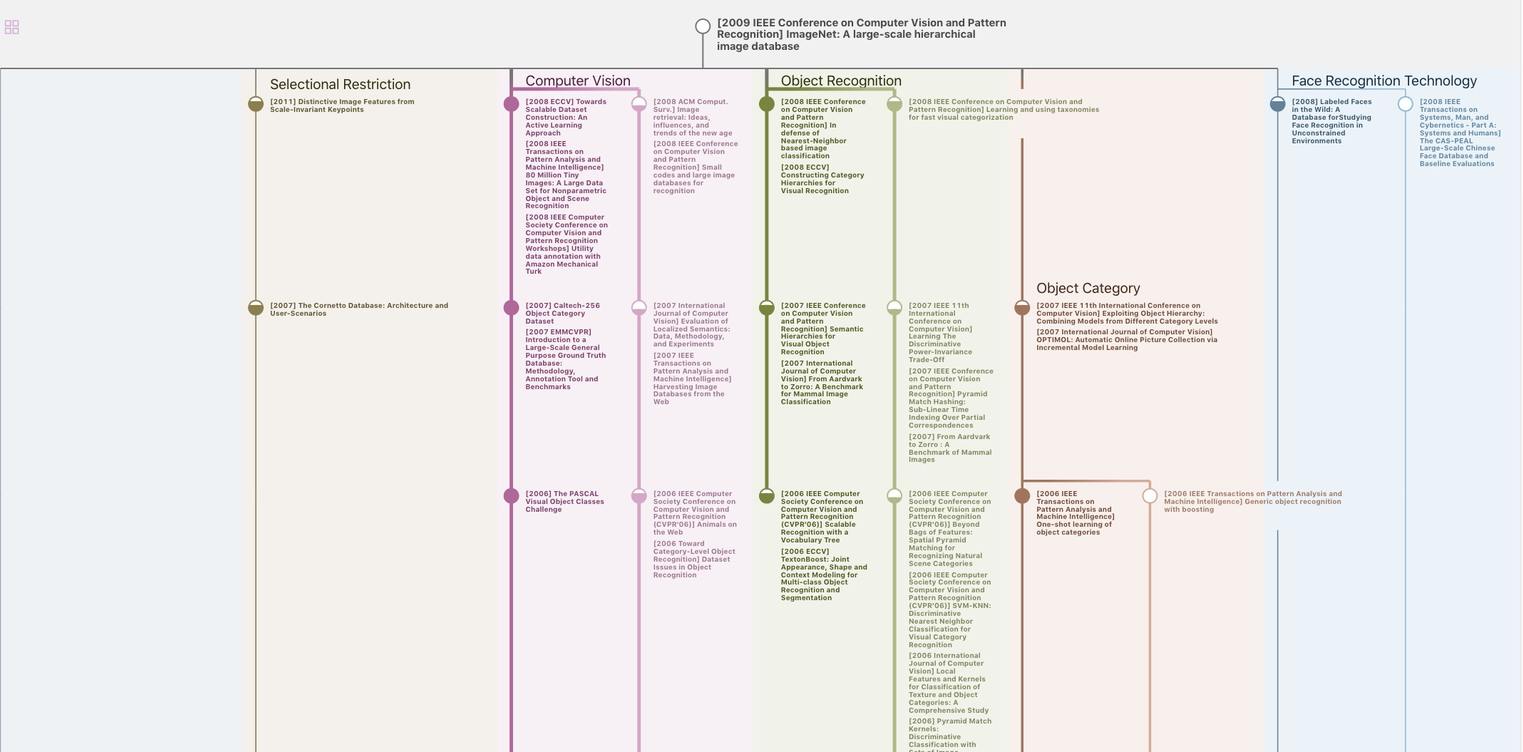
生成溯源树,研究论文发展脉络
Chat Paper
正在生成论文摘要