MGBM-YOLO: a Faster Light-Weight Object Detection Model for Robotic Grasping of Bolster Spring Based on Image-Based Visual Servoing
JOURNAL OF INTELLIGENT & ROBOTIC SYSTEMS(2022)
摘要
The rapid detection and accurate positioning of bolster spring with complex geometric features in cluttered background is highly important for the grasping task of bolster spring in overhauling workshop. To achieve a better trade-off among positioning accuracy, running time and model size, two MobileNetv3 and GhostNet-based modified YOLOv3 (MGBM-YOLO) models are proposed in this paper: MGBM-YOLO-Large and MGBM-YOLO-Small, which are applied to the robotic grasping system of bolster spring based on image-based visual servoing (IBVS). The proposed MGBM-YOLO models are trained and evaluated on Pascal VOC2007 and Pascal VOC2012 datasets. The results show that compared with the original YOLOv3 model based on Darknet53, the sizes of the proposed Large and Small models are reduced by 59% and 66% respectively, and the model parameters are reduced by 63% and 66% respectively. The detection speed is 4x and 5.5x faster respectively when the difference of mAP is small. To improve the convergence speed of IBVS system, an online depth estimation method based on the area feature of detection bounding box of bolster spring is proposed. The comparative experiments of visual servoing grasping are conducted on the robotic grasping platform of bolster spring. The experimental results show that the two MGBM-YOLO detection models proposed in this paper can meet the requirements of fast detection and positioning of bolster spring robotic grasping system based on IBVS. The proposed method of feature depth online estimation is of great significance to accelerate the convergence speed of visual servoing system.
更多查看译文
关键词
Modified YOLOv3 model,Light-weight neural network,Depth online estimation,Visual servoing,Bolster spring grasping
AI 理解论文
溯源树
样例
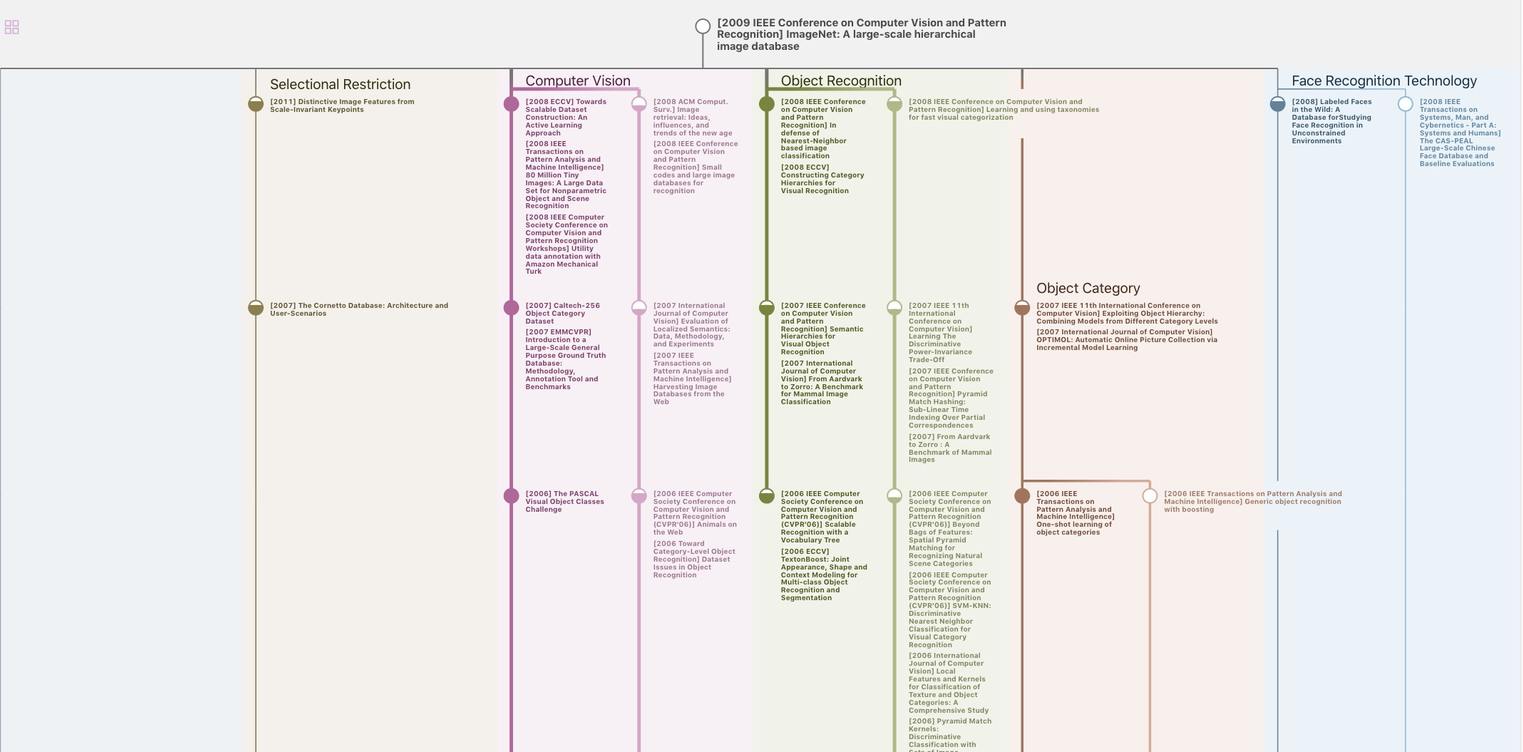
生成溯源树,研究论文发展脉络
Chat Paper
正在生成论文摘要