MCCS-LSTM: Extracting Full-Image Contextual Information and Multi-Scale Spatiotemporal Feature for Radar Echo Extrapolation
ATMOSPHERE(2022)
摘要
Precipitation nowcasting has been gaining importance in the operational weather forecast, being essential for economic and social development. Conventional methods of precipitation nowcasting are mainly focused on the task of radar echo extrapolation. In recent years, deep learning methods have been used in this task. Nevertheless, raising the accuracy and extending the lead time of prediction remains as a challenging problem. To address the problem, we proposed a Multi-Scale Criss-Cross Attention Context Sensing Long Short-Term Memory (MCCS-LSTM). In this model, Context Sensing framework (CS framework) focuses on contextual correlations, and Multi-Scale Spatiotemporal block (MS block) with criss-cross attention is designed to extract multi-scale spatiotemporal feature and full-image dependency. To validate the effectiveness of our model, we conduct experiments on CIKM AnalytiCup 2017 data sets and Guangdong Province of China radar data sets. By comparing with existing deep learning models, the results demonstrate that the MCCS-LSTM has the best prediction performance, especially for predicting accuracy with longer lead times.
更多查看译文
关键词
radar echo extrapolation,deep learning,multi-scale spatiotemporal feature
AI 理解论文
溯源树
样例
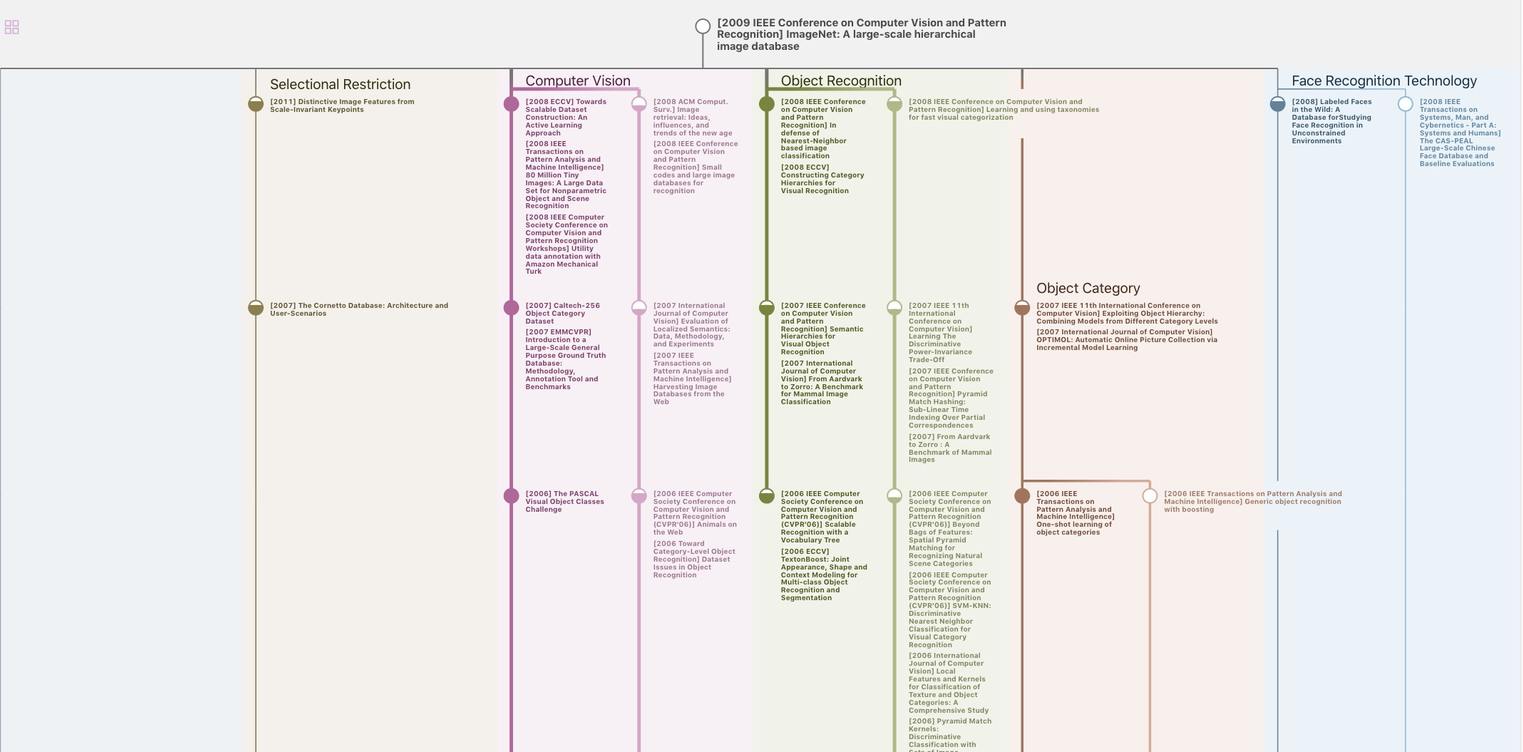
生成溯源树,研究论文发展脉络
Chat Paper
正在生成论文摘要