Attention Network Forecasts Time-to-Failure in Laboratory Shear Experiments
JOURNAL OF GEOPHYSICAL RESEARCH-SOLID EARTH(2021)
摘要
Rocks under stress deform by creep mechanisms that include formation and slip on small-scale internal cracks. Intragranular cracks and slip along grain contacts release energy as elastic waves termed acoustic emissions (AE). AEs are thought to contain predictive information that can be used for fault failure forecasting. Here, we present a method using unsupervised classification and an attention network to forecast labquakes using AE waveform features. Our data were generated in a laboratory setting using a biaxial shearing device with granular fault gouge intended to mimic the conditions of tectonic faults. Here, we analyzed the temporal evolution of AEs generated throughout several hundred laboratory earthquake cycles. We used a Conscience Self-Organizing Map (CSOM) to perform topologically ordered vector quantization based on waveform properties. The resulting map was used to interactively cluster AEs. We examined the clusters over time to identify those with predictive ability. Finally, we used a variety of LSTM and attention-based networks to test the predictive power of the AE clusters. By tracking cumulative waveform features over the seismic cycle, the network is able to forecast the time-to-failure (TTF) of lab earthquakes. Our results show that analyzing the data to isolate predictive signals and using a more sophisticated network architecture are key to robustly forecasting labquakes. In the future, this method could be applied on tectonic faults to monitor earthquakes and augment early warning systems. Plain Language Summary Earthquake forecasting is a daunting task, but advances in machine learning can help us toward this goal. In this study, we combine machine learning with expert knowledge about earthquake formation to forecast synthetic earthquakes made in a laboratory. Prior to an earthquake, rocks emit small bursts of energy as they are stressed, which are called acoustic emissions (AE). We identified all the AEs in the raw seismic data from our lab earthquakes and then used a machine-learning algorithm called a Conscience Self- Organizing Map to divide them into groups based on their characteristics. We examined the groups to determine which ones would be useful for earthquake forecasting. Finally, we used the data from the chosen groups along with another machine learning approach (LSTM and attention networks) to forecast the time of future labquakes. Our results show that earthquake forecasting is improved by processing seismic data to find predictive signals and by using machine-learning networks that are specifically made for time series data.
更多查看译文
AI 理解论文
溯源树
样例
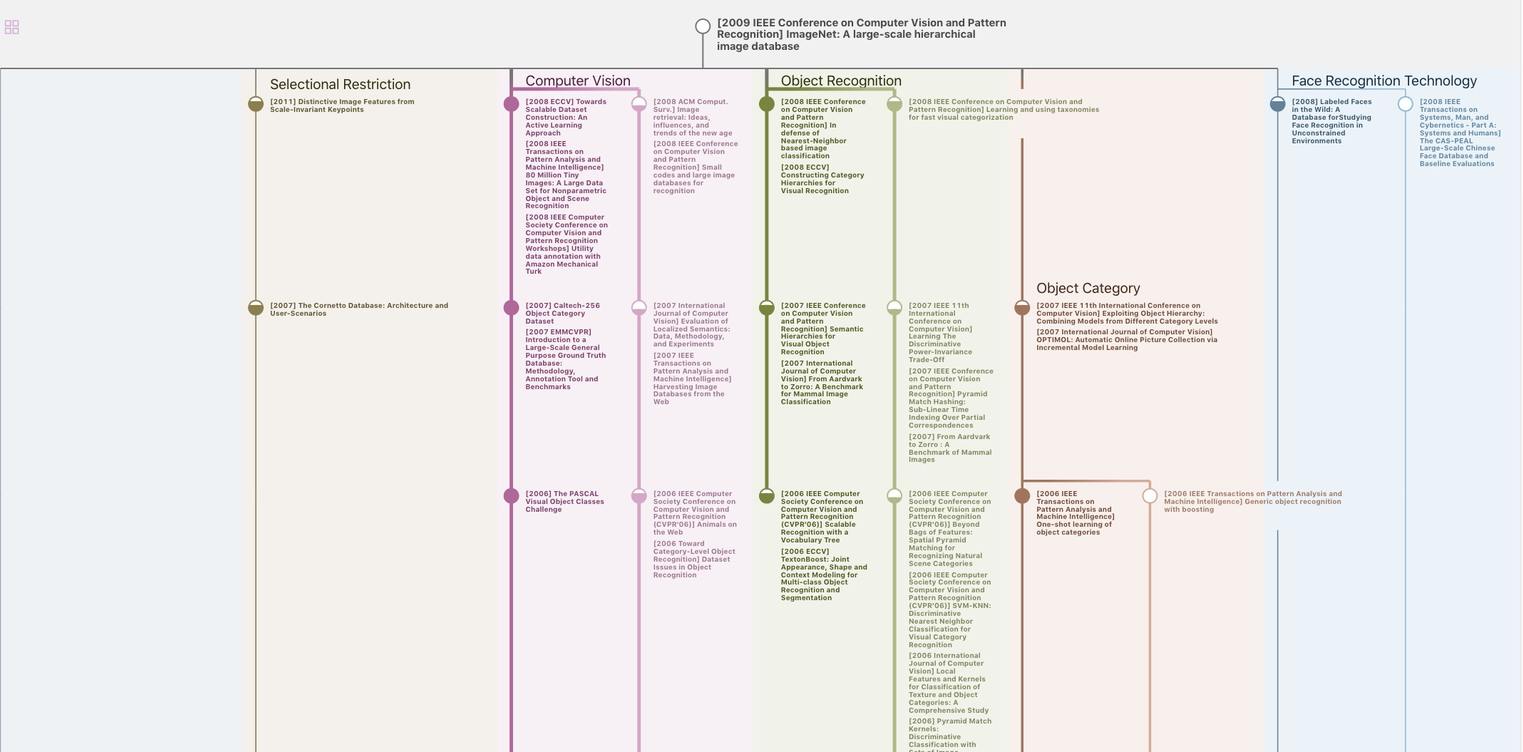
生成溯源树,研究论文发展脉络
Chat Paper
正在生成论文摘要