An ensembling model for early identification of at-risk students in higher education
COMPUTER APPLICATIONS IN ENGINEERING EDUCATION(2022)
摘要
Audience Response Systems like clickers are gaining much attention for early identification of at-risk students as quality education, student success rate and retention are major concerning areas, as evidenced in this COVID scenario. Usage of this active learning strategy across the varying strength of classrooms are found to be much effective in retaining the attention, retention and learning power of the students. However, implementing clickers for large classrooms incur overhead costs on instructor's part. As a result, educational researchers are experimenting with various lightweight alternatives. This paper discusses one such alternative: lightweight formative assessments for blended learning environments. It discusses their implementation and effectiveness in early identification of at-risk students. This study validates the usage of lightweight assessments for three core pedagogically different courses of large computer science engineering classrooms. It uses voting ensemble classifier for effective predictions. With the usage of lightweight assessments in early identification of at-risk students, accuracy range of 87%-94.7% have been achieved along-with high ROC-AUC values. The study also proposes the generalized pedagogical architecture for fitting in these lightweight assessments within the course curriculum of pedagogically different courses. With the constructive outcomes, the light-weight assessments seem to be promising for efficient handling of scaling technical classrooms.
更多查看译文
关键词
at-risk students, blended learning environments, computer science engineering, ensemble classifier, lightweight formative assessments, pedagogical architecture
AI 理解论文
溯源树
样例
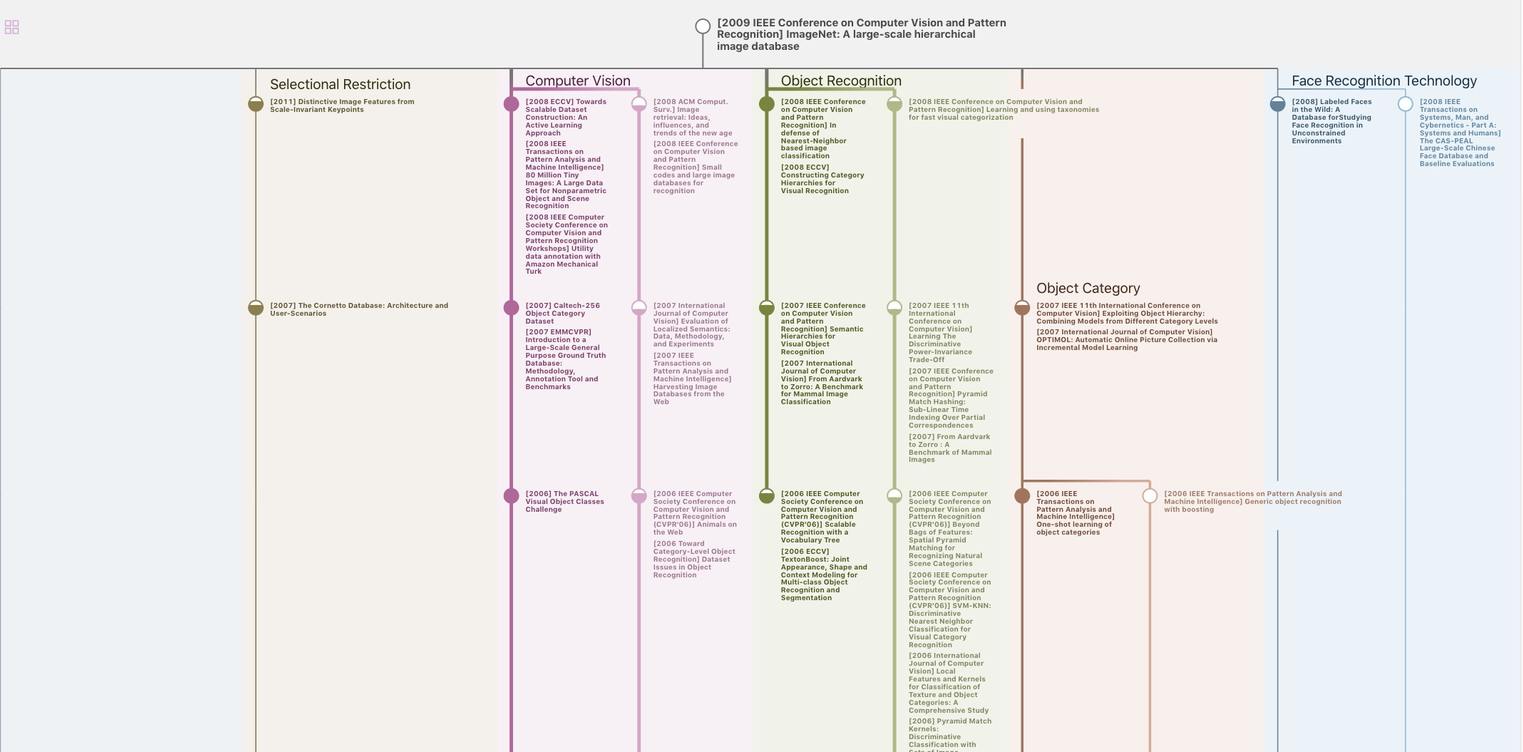
生成溯源树,研究论文发展脉络
Chat Paper
正在生成论文摘要