In-process acoustic pore detection in milling using deep learning
CIRP JOURNAL OF MANUFACTURING SCIENCE AND TECHNOLOGY(2022)
摘要
Cast parts tend to show quality relevant pores and cracks on the inside. During machining operations those defects are exposed, but often not detected. This paper presents an in-process pore detection method for machining operations using a structure-borne acoustic sensor. By detecting the defects in-process, the machining operation can be stopped immediately if those defects are detected. A test case using additive manufactured workpieces with repeatable cavities was implemented, demonstrating the in-process pore detection and localization. The acoustic signals are analyzed both in the time domain and in the frequency domain, using deep learning methods. On experimental AlSi10Mg parts, pores could be detected with a quantified uncertainty using the applied methodology during a milling process.(c) 2022 CIRP.
更多查看译文
关键词
In-process measurement, Acoustic emission, Failure, Milling, Artificial intelligence, Machine learning, In-process measurement, Acoustic emission, Failure, Milling, Artificial intelligence, Machine learning
AI 理解论文
溯源树
样例
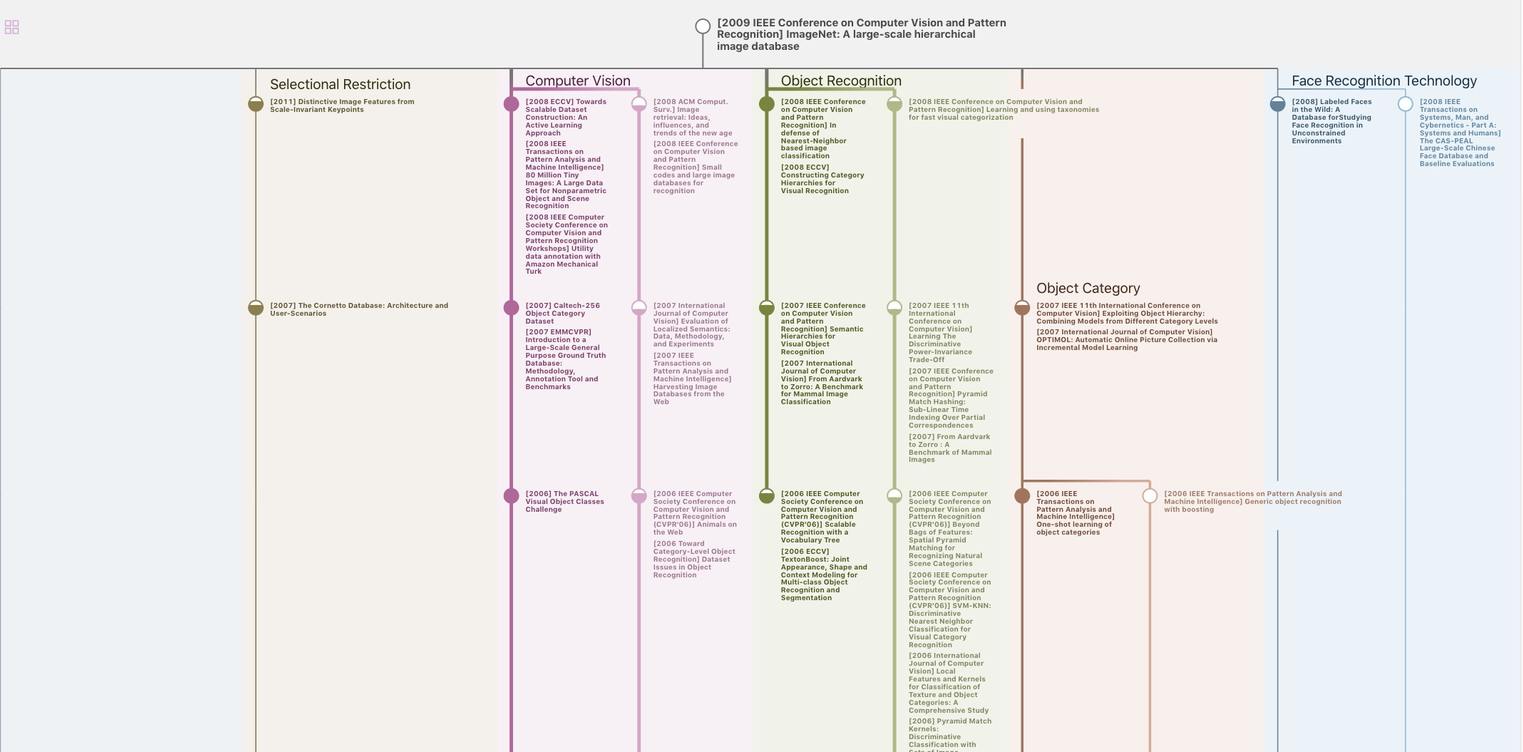
生成溯源树,研究论文发展脉络
Chat Paper
正在生成论文摘要