Optimally organized GRU-deep learning model with Chi(2) feature selection for heart disease prediction
JOURNAL OF INTELLIGENT & FUZZY SYSTEMS(2022)
摘要
In previous studies, various machine-driven decision support systems based on recurrent neural networks (RNN) were ordinarily projected for the detection of cardiovascular disease. However, the majority of these approaches are restricted to feature preprocessing. In this paper, we concentrate on both, including, feature refinement and the removal of the predictive model's problems, e.g., underfitting and overfitting. By evading overfitting and underfitting, the model will demonstrate good enactment on equally the training and testing datasets. Overfitting the training data is often triggered by inadequate network configuration and inappropriate features. We advocate using Chi(2) statistical model to remove irrelevant features when searching for the best-configured gated recurrent unit (GRU) using an exhaustive search strategy. The suggested hybrid technique, called Chi(2) GRU, is tested against traditional ANN and GRU models, as well as different progressive machine learning models and antecedently revealed strategies for cardiopathy prediction. The prediction accuracy of proposed model is 92.17%. In contrast to formerly stated approaches, the obtained outcomes are promising. The study's results indicate that medical practitioner will use the proposed diagnostic method to reliably predict heart disease.
更多查看译文
关键词
Gated recurrent unit, heart disease, overfitting, underfitting, feature selection
AI 理解论文
溯源树
样例
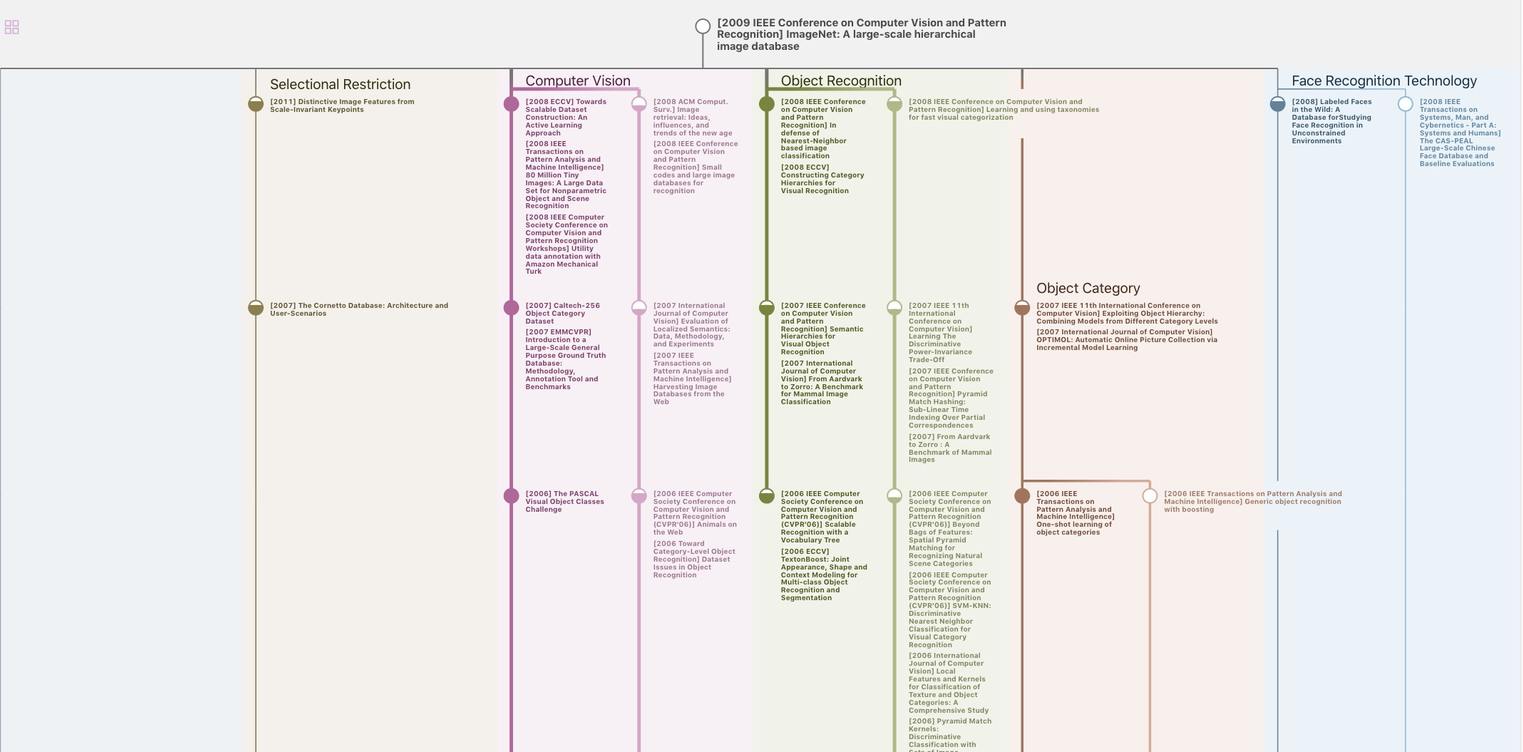
生成溯源树,研究论文发展脉络
Chat Paper
正在生成论文摘要