A spatial-temporal topic model with sparse prior and RNN prior for bursty topic discovering in social networks
JOURNAL OF INTELLIGENT & FUZZY SYSTEMS(2022)
摘要
With the rapid growth of social network users, the social network has accumulated massive social network topics. However, due to the randomness of content, it becomes sparse and noisy, accompanied by many daily chats and meaningless topics, which brings challenges to bursty topics discovery. To deal with these problems, this paper proposes the spatial-temporal topic model with sparse prior and recurrent neural networks (RNN) prior for bursty topic discovering (ST-SRTM). The semantic relationship of words is learned through RNN to alleviate the sparsity. The spatial-temporal areas information is introduced to focus on bursty topics for further weakening the semantic sparsity of social network context. Besides, we introduced the "Spike and Slab" prior to decouple the sparseness and smoothness. Simultaneously, we realized the automatic discovery of social network bursts by introducing the burstiness of words as the prior and binary switching variables. We constructed multiple sets of comparative experiments to verify the performance of ST-SRTM by leveraging different evaluation indicators on real Sina Weibo data sets. The experimental results confirm the superiority of our ST-SRTM.
更多查看译文
关键词
Social network, bursty topic, topic model, RNN, sparse prior
AI 理解论文
溯源树
样例
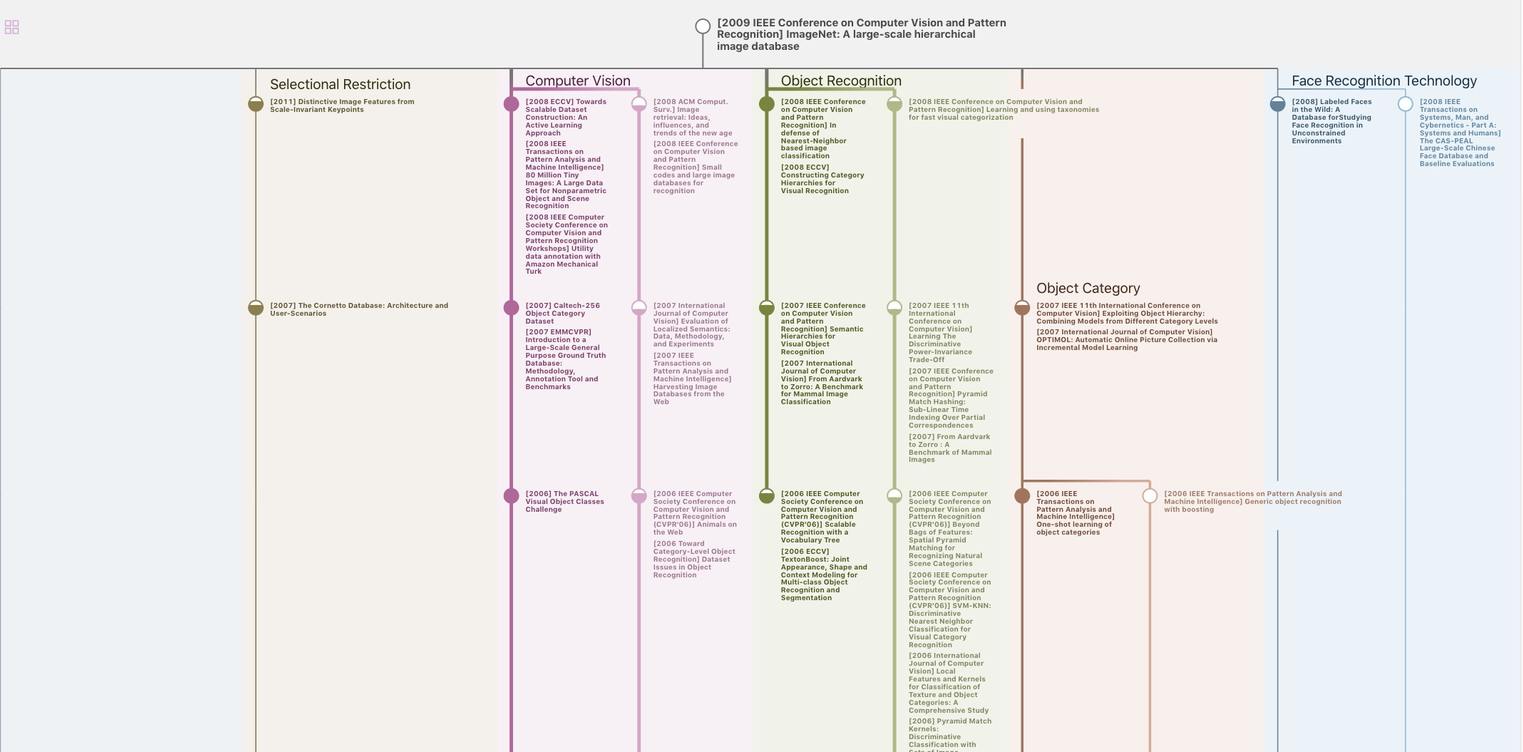
生成溯源树,研究论文发展脉络
Chat Paper
正在生成论文摘要