Video Visual Relation Detection via 3D Convolutional Neural Network
IEEE ACCESS(2022)
摘要
Video visual relation detection, which aims to detect the visual relations between objects in the form of relation triplet (e.g., "person-ride-bike", "dog-toward-car", etc.), is a significant and fundamental task in computer vision. However, most of the existing works about visual relation instances are focused on static images. Modeling the non-static relationships in videos has drawn little attention due to lacking large-scale video dataset support. In our work, we propose a video dataset named Video Predicate Detection and Reasoning (VidPDR) for dynamic video visual relation detection, which consists of 1,000 videos with dense manually dynamic labeled annotations on 21 object classes and 37 predicates classes. Moreover, we propose a novel spatio-temporal feature extraction framework with 3D Convolutional Neural Networks (ST3DCNN), which includes three modules 1) object trajectory, 2) short-term relation prediction, and 3) greedy relational association. We conducted appropriate experiments on public datasets and our own dataset (VidPDR). Results demonstrate that our proposed method has a great improvement in comparison to the state-of-the-art baselines.
更多查看译文
关键词
Visualization, Feature extraction, Trajectory, Three-dimensional displays, Convolutional neural networks, Task analysis, Object detection, Computer vision, 3D convolutional neural network, video visual relation detection
AI 理解论文
溯源树
样例
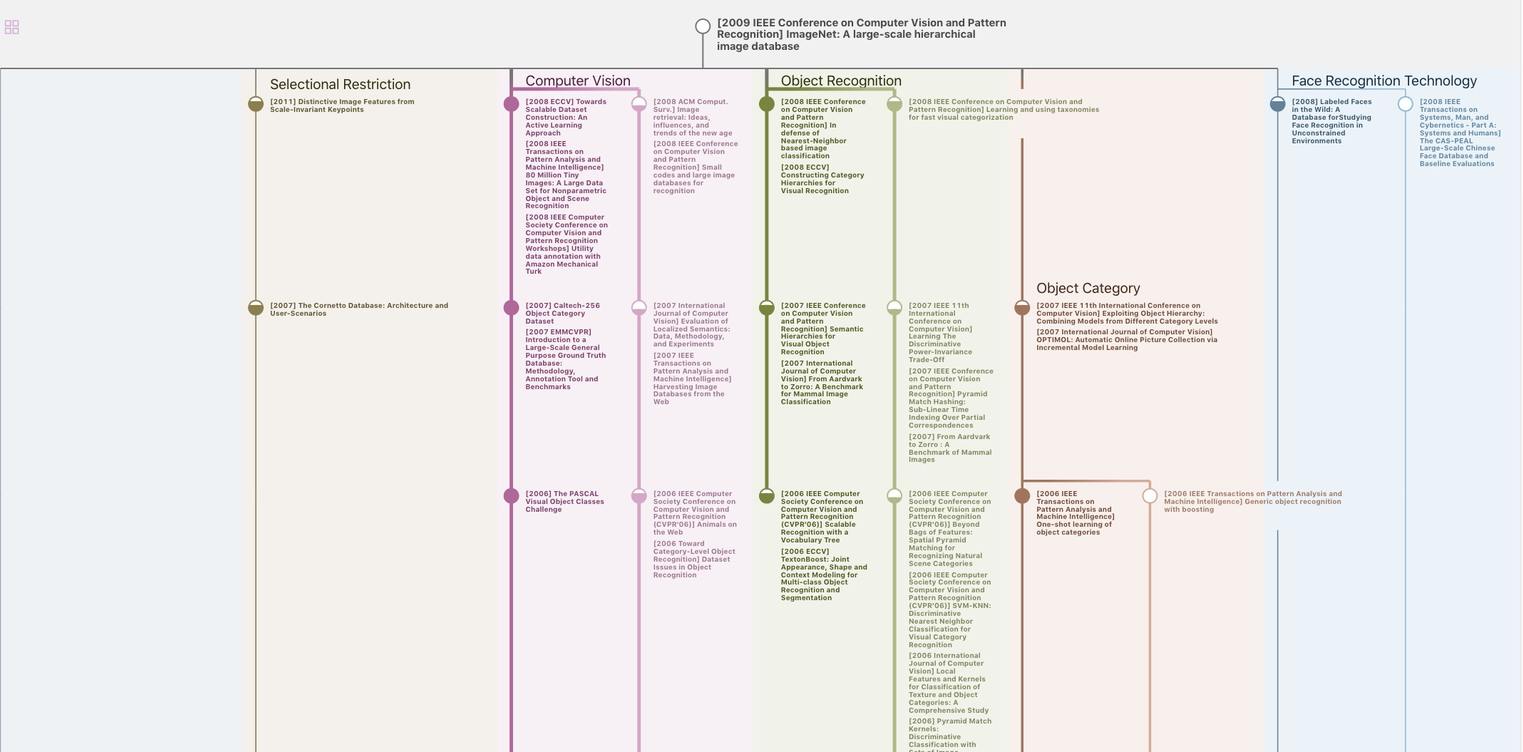
生成溯源树,研究论文发展脉络
Chat Paper
正在生成论文摘要