An Overview of Deeply Optimized Convolutional Neural Networks and Research in Surface Defect Classification of Workpieces
IEEE ACCESS(2022)
摘要
Currently, the development of industry is becoming increasingly rapid. Technicalization, informatization and industrialization give the fundamental impetus for industrial development and progress. Nevertheless, there are numerous problems that are hindering industrial progress and threatening human security in the industrial field. The surface defects of the workpieces are one of the primary problems. Moreover, defects of multi-type, mixed and unapparent characteristics presented by workpieces make the detection and classification of workpiece more difficult. Deep convolutional neural networks (DCNN) show strong ability of feature extraction and mines deeper essential features of data because of its features of unique receptive field structure and weights of shared. It can represent original data information well and obtain results more accurately than the traditional methods. But there also remains a problem that conventional DCNN has a huge number of parameters and computation, which brings great pressure to the equipment in terms of computing power, memory, speed and so on. Based on this situation, the optimization methods of CNNs model in the aspects of data, structure, algorithm are summarized. Related lightweight structures and networks are also summarized in this paper. The purpose of these work is to reduce the number of parameters and computation and improve the training performance. At the same time, the research on defect classification of workpieces based on traditional machine learning and deep learning model is reviewed, and the research on defect classification of workpieces based on deeply optimized CNNs is referred and prospected.
更多查看译文
关键词
Feature extraction, Support vector machines, Surface cracks, Deep learning, Classification algorithms, Machine learning, Task analysis, Deeply optimized CNN, workpieces detection, lightweight, surface defect classification
AI 理解论文
溯源树
样例
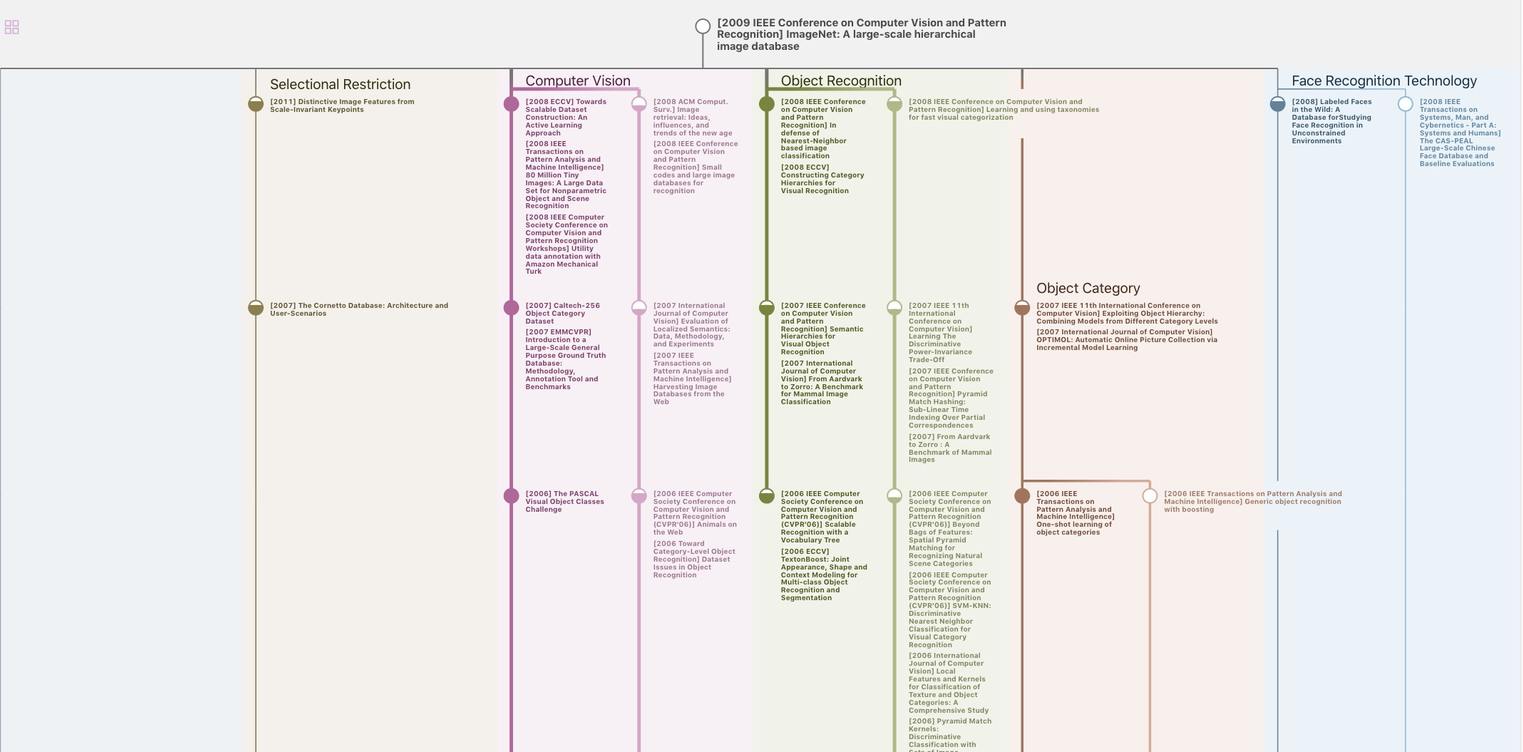
生成溯源树,研究论文发展脉络
Chat Paper
正在生成论文摘要