ADS-B-Based Spatiotemporal Alignment Network for Airport Video Object Segmentation
IEEE Transactions on Intelligent Transportation Systems(2022)
摘要
Video object segmentation (VOS) is the fundamental problem of vision-based intelligent transportation, and many VOS algorithms relying on inference from reference masks have been proposed. Due to the inherent defects of the inference strategy and the complex changes of targets, VOS methods that perform well on public datasets are usually ineffective in airport scenarios. We propose a spatiotemporal alignment network (STA-Net) that makes use of Automatic Dependent Surveillance-Broadcast (ADS-B) data as prior information to guide the long-term segmentation of aircraft. ADS-B is an airport-specific signal, which indicates the location of aircraft in real time. Based on ADS-B, we continuously generate new reference masks instead of using previous masks for inference, which greatly reduces the accumulation of inference errors. To achieve this, previous masks of each aircraft are aligned on the temporal domain based on the position information in ADS-B. All temporally-aligned masks are compared, and the one most similar to the current instant is reserved. This mask is both temporally and spatially aligned; hence it is a better reference mask for inference. Aligned masks are updated every time new ADS-B data arrive, so that they can support long-term inference. With the selected mask as a reference, aircraft of interest are segmented within a unified encoder-decoder framework over the long term. Experiments on a benchmark dataset and in a real airport scenario verify the effectiveness of the presented method.
更多查看译文
关键词
ADS-B,video object segmentation,mask propagation,mask matching,airport ground surveillance
AI 理解论文
溯源树
样例
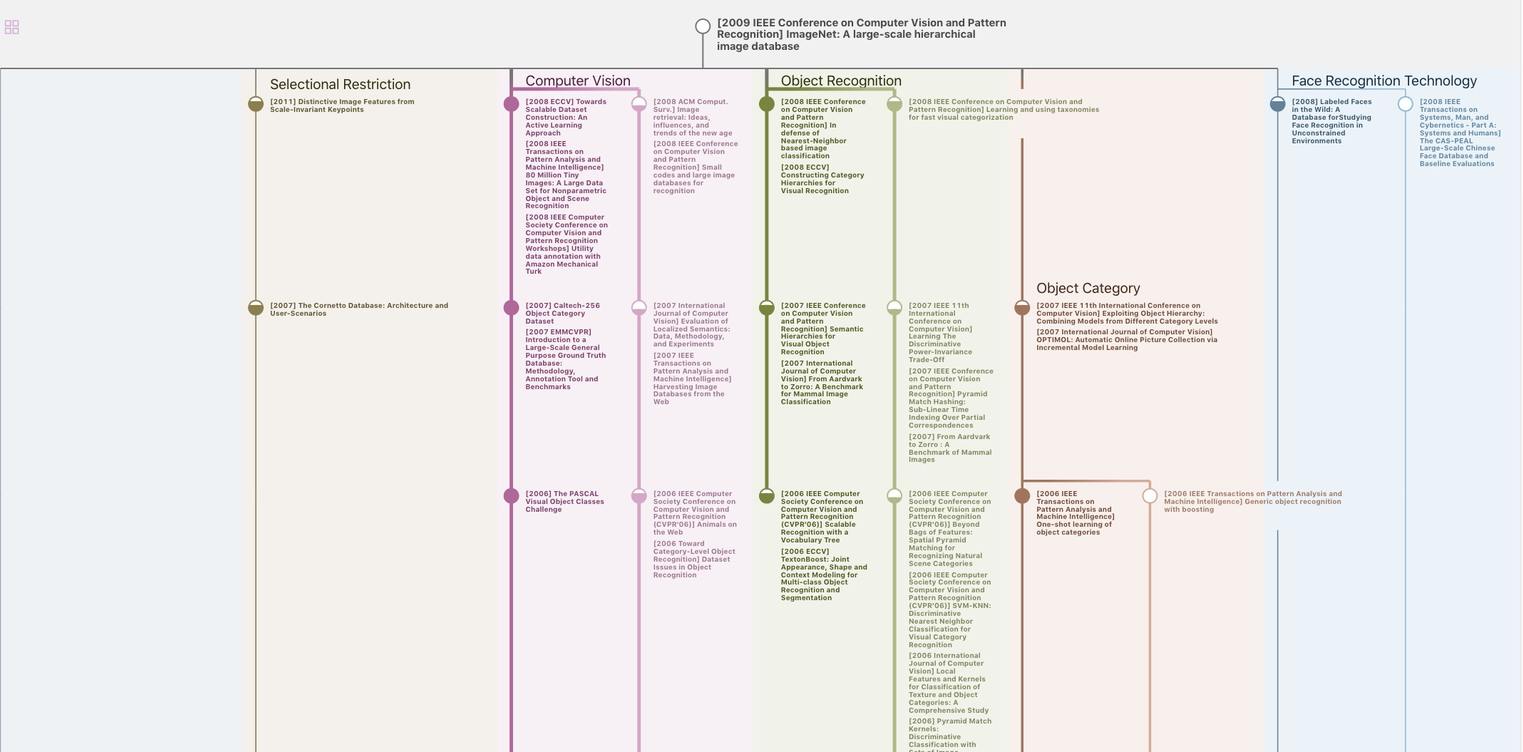
生成溯源树,研究论文发展脉络
Chat Paper
正在生成论文摘要