Automating Risk Assessment of Acute Kidney Injury for Patients Undergoing Percutaneous Coronary Intervention
CIRCULATION(2021)
摘要
Background: The risk of acute kidney injury (AKI) for percutaneous coronary intervention (PCI) can inform decision-making and mitigation. However, risk assessment is hampered by the burden of manually abstracting and inputting data required by the established model developed on registry data. Accordingly, there is a need to develop AKI models that use automatically extracted electronic health records (EHR) data and evaluate their performance in comparison to the contemporary registry model. Methods: We used EHR data for PCIs performed at Yale New Haven Hospital (YNHH) and the linked local National Cardiovascular Data Registry (NCDR) CathPCI data to develop and compare the EHR models with the NCDR model. AKI was defined as an increase of ≥0.3 mg/dL or 50% in serum creatinine. To maximize interoperability across systems and usability at the point of care, we only considered structured EHR readily extractable before PCI, and implemented simple but universal data preprocessing. Longitudinal data was aggregated into 24h, 7d and 14d prior to PCI. We used Lasso regression and gradient descent boosting (GDB) to train the models and tested their capacity to approximate the NCDR model predictions and predict AKI independently. We evaluated performance via cross-validation by c-statistic, calibration slope, and predictive range defined by event rate difference in the lowest and highest risk deciles. We used paired t tests for model comparison. Results: The cohort included 9,202 PCIs from December 2012 to November 2019 at YNHH for 8,445 patients and had a mean age of 67.0±12.0 years with 27.6% females and 798 (8.7%) AKIs. Evaluated on the same test sets, the EHR models approximating the NCDR model predictions achieved similar performance to that model (all p>0.05). Further, the EHR model employing GDB to independently predict AKI achieved slightly better performance than the NCDR model in c-statistic (0.84 vs 0.83, p=0.02) and predictive range (41.2% vs 39.5%, p=0.13) with similar calibration (slope 0.98 vs 0.99). Conclusion: The automatically extracted EHR data produced models with similar performance to the registry model. These models can automate AKI risk assessment for PCI, alleviating provider burden and increase uptake of risk assessment.
更多查看译文
AI 理解论文
溯源树
样例
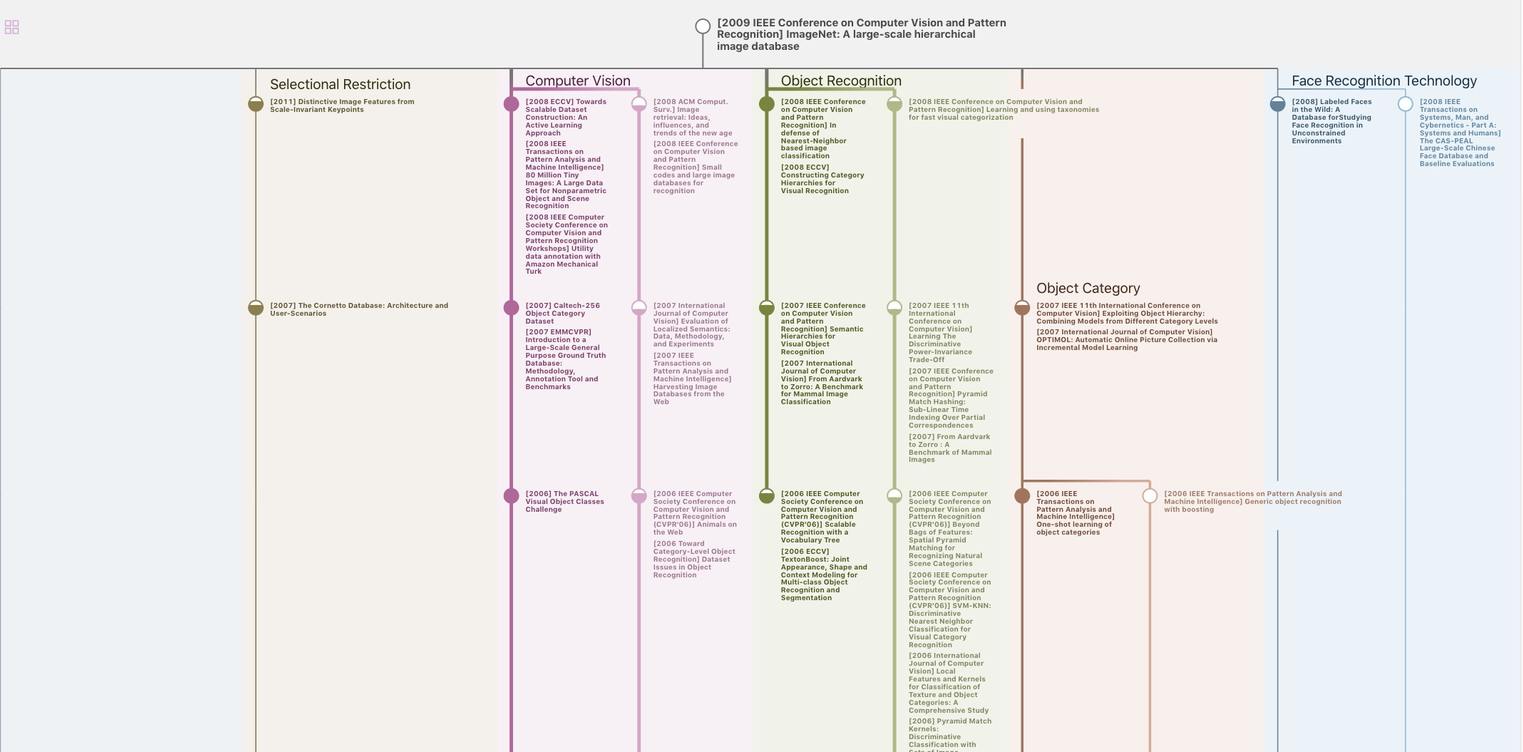
生成溯源树,研究论文发展脉络
Chat Paper
正在生成论文摘要