Visual Tracking With Siamese Network Based on Fast Attention Network
IEEE ACCESS(2022)
摘要
Visual tracking remains an open challenge, as it requires real-time and long-term accurate target prediction. Siamese network has been widely studied due to its excellent accuracy and speed. Since long-term tracking may lead to model degradation and drift, most existing algorithms cannot well solve this problem. This article proposes a new Siamese Network based on Fast Attention Network named SiamFA. This method designs an attention model, which can enhance the key and global information of the target, to obtain a more robust target model and achieve long-term tracking. At the same time, the attention model is used to obtain the potential position information of the target when calculating the similarity between the template and the search area. In addition, the attention network we design reduces many redundant operations and effectively improves computational efficiency. We utilize a multi-layer perceptron to forecast the bounding box to avoid excessive hyper-parameters. In order to verify the effectiveness of our network, we conduct tests on many commonly used datasets, such as OTB100, GOT-10k, LaSOT, TrackingNet, UAV123. Our method can achieve a success rate of 62.7% and the precision rate of 64.3% on LaSOT. At the same time, it can run at about 100fps, which exceeds the comparison network, proving that our network can run in real-time.
更多查看译文
关键词
Target tracking,Licenses,Residual neural networks,Convolution,Adaptation models,Training,Task analysis,Visual tracking,deep learning,Siamese network,attention mechanism,multi-layer perceptron
AI 理解论文
溯源树
样例
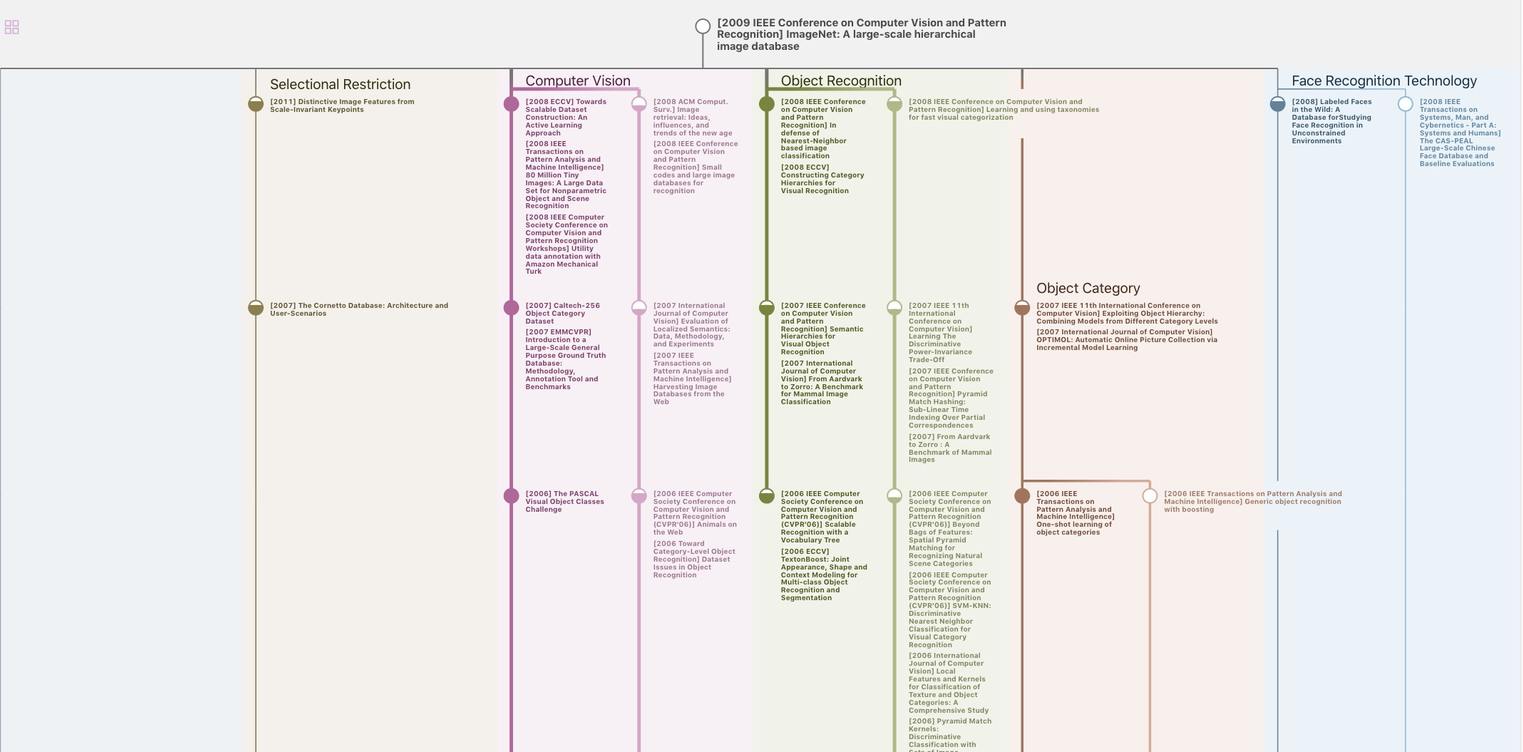
生成溯源树,研究论文发展脉络
Chat Paper
正在生成论文摘要