Remaining useful life prognosis for wind turbine using a neural network with a long-term prediction
WIND ENGINEERING
摘要
In this paper, an artificial neural network (ANN) is used to predict degradation phenomena occurring in high-speed shaft bearings wind turbine systems, and predict their remaining useful life (RUL). Two different prediction ways are possible. The first is known as short-term prediction, and it involves using measured three data from prior cycles to anticipate degradation in the present cycle. This is a future prediction. The difficulty with short-term prediction is that it is impossible to predict degradation in the future due to a lack of measurement data. Short-term prediction, on the other hand, is accurate because it is based on real measured data and the extrapolation distance is short. The second method is known as long-term prediction, where predicted degradations are used to predict the degradation at a further future time. This paper considers only the long-term prediction. The method was initially tested by using the experimental vibration data provided by the GPMS database, where the RUL was accurately predicted with a very small uncertainty.
更多查看译文
关键词
Artificial neural network, bearing, data acquisition, health indicator, prognostics and health management, prognostics approaches, remaining useful life prediction, wind turbine generators
AI 理解论文
溯源树
样例
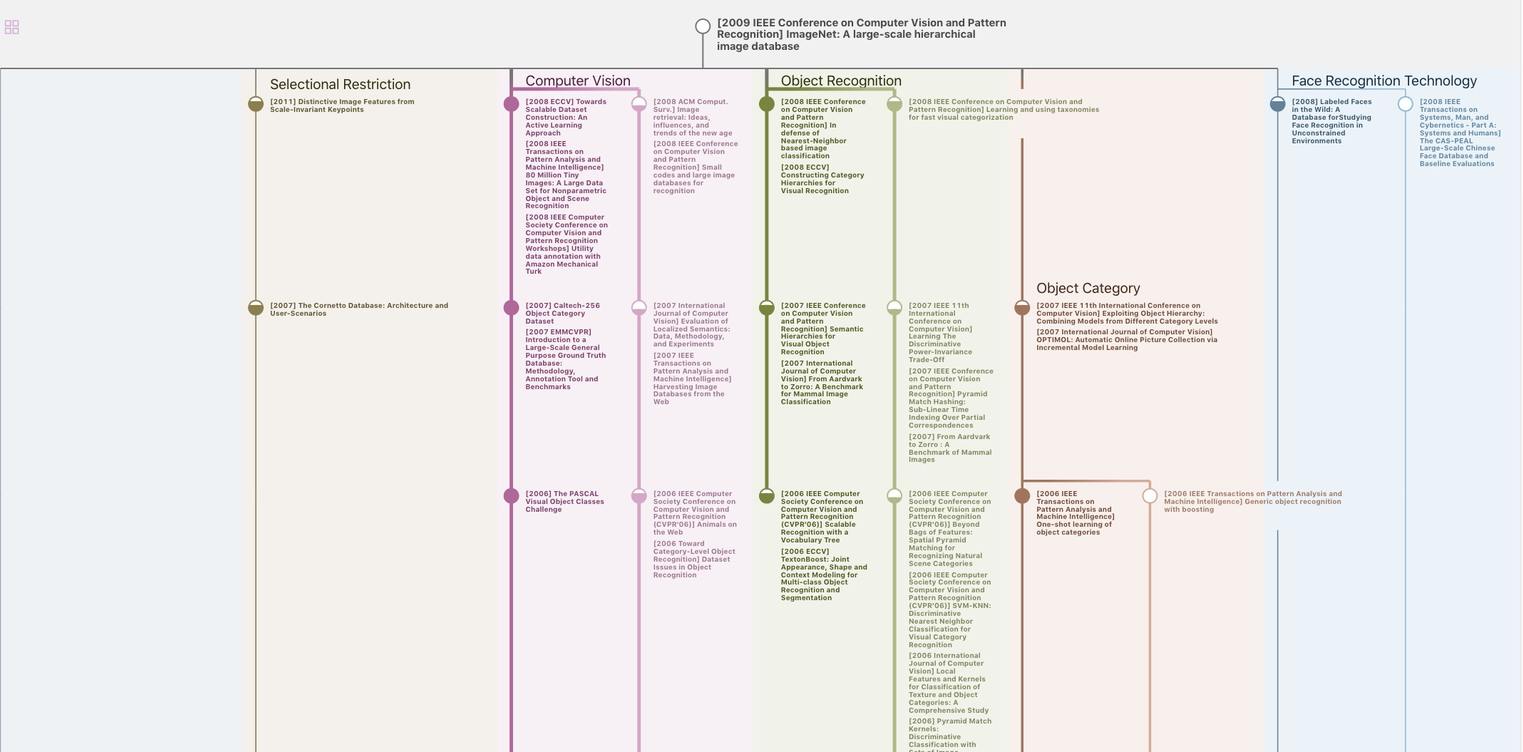
生成溯源树,研究论文发展脉络
Chat Paper
正在生成论文摘要