Item-level Forecasting for E-commerce Demand with High-dimensional Data Using a Two-stage Feature Selection Algorithm
Journal of Systems Science and Systems Engineering(2022)
Abstract
With the rapid development of information technology and fast growth of Internet users, e-commerce nowadays is facing complex business environment and accumulating large-volume and high-dimensional data. This brings two challenges for demand forecasting. First, e-merchants need to find appropriate approaches to leverage the large amount of data and extract forecast features to capture various factors affecting the demand. Second, they need to efficiently identify the most important features to improve the forecast accuracy and better understand the key drivers for demand changes. To solve these challenges, this study conducts a multi-dimensional feature engineering by constructing five feature categories including historical demand, price, page view, reviews, and competition for e-commerce demand forecasting on item-level. We then propose a two-stage random forest-based feature selection algorithm to effectively identify the important features from the high-dimensional feature set and avoid overfitting. We test our proposed algorithm with a large-scale dataset from the largest e-commerce platform in China. The numerical results from 21,111 items and 109 million sales observations show that our proposed random forest-based forecasting framework with a two-stage feature selection algorithm delivers 11.58%, 5.81% and 3.68% forecast accuracy improvement, compared with the Autoregressive Integrated Moving Average (ARIMA), Random Forecast, and Random Forecast with one-stage feature selection approach, respectively, which are widely used in literature and industry. This study provides a useful tool for the practitioners to forecast demands and sheds lights on the B2C e-commerce operations management.
MoreTranslated text
Key words
Forecasting,e-commerce,high-dimensional feature,feature selection
AI Read Science
Must-Reading Tree
Example
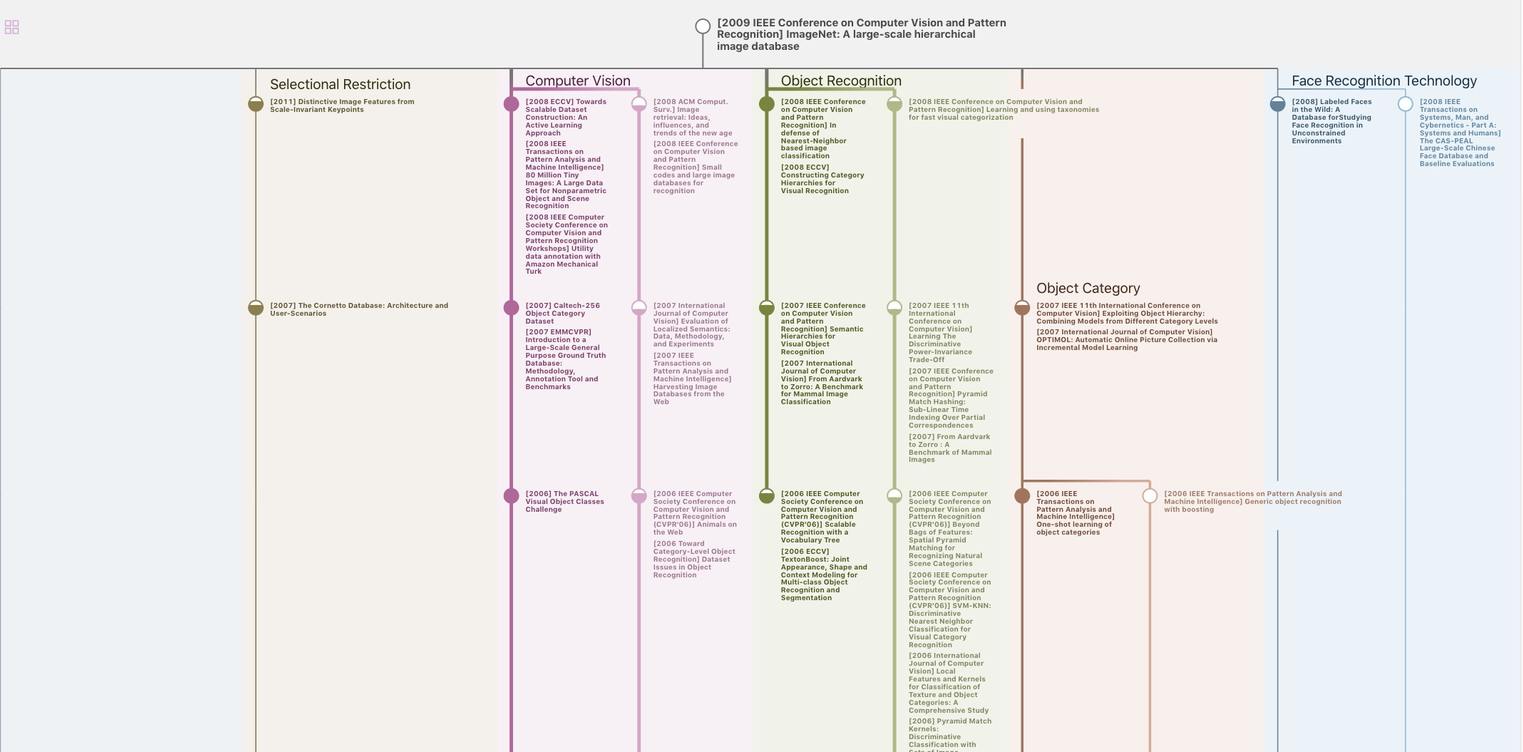
Generate MRT to find the research sequence of this paper
Chat Paper
Summary is being generated by the instructions you defined