Lithology identification of logging data based on improved neighborhood rough set and AdaBoost
Earth Science Informatics(2022)
Abstract
Traditional lithology identification left the problems of low accuracy, recognition efficiency and generalization ability. Facing the logging data with outliers, unbalance and high complexity, we propose a lithology identification method based on an improved neighborhood rough set and AdaBoost. On the basis of the classical neighborhood rough set, the selection of the neighborhood radius and the running time are optimized. The redundant information in logging data is then effectively eliminated. Thus more sensitive logging curves are selected without changing the physical meaning of logging attributes. Then the selected data are input into the AdaBoost model to construct a lithology identification model. About 54,000 samples from 5 boreholes are tested in the study area. The accuracy of classification on the test set is about 98.42%. Compared with BP neural network and random forest algorithm, the proposed method owns advantages in recognition accuracy and generalization ability. It can provide help for complex lithology recognition in the study area.
MoreTranslated text
Key words
Neighborhood rough set, AdaBoost, Logging data, Lithology identification
AI Read Science
Must-Reading Tree
Example
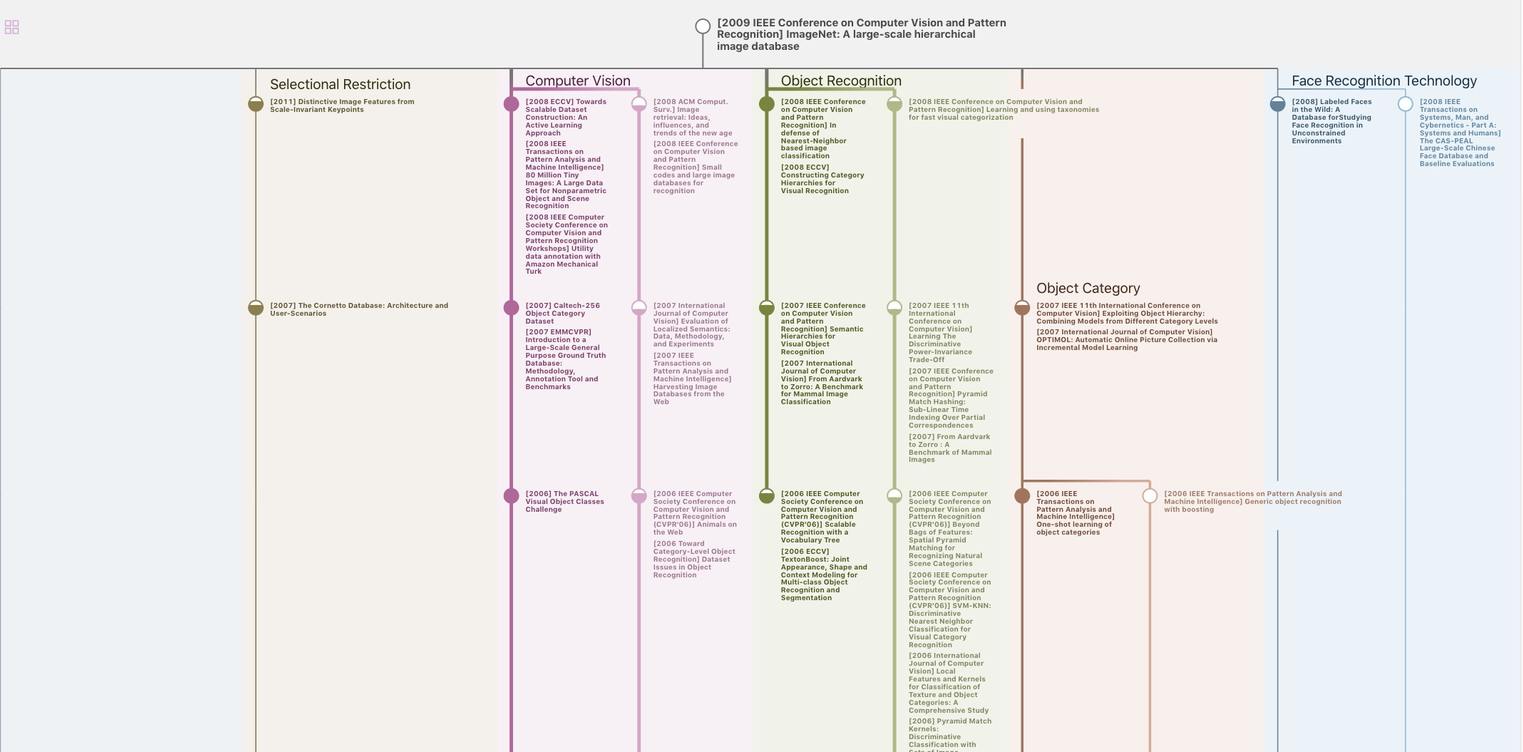
Generate MRT to find the research sequence of this paper
Chat Paper
Summary is being generated by the instructions you defined