HCovBi-Caps: Hate Speech Detection Using Convolutional and Bi-Directional Gated Recurrent Unit With Capsule Network
IEEE ACCESS(2022)
摘要
Adversaries and anti-social elements have exploited the rapid proliferation of computing technology and online social media in the form of novel security threats, such as fake profiles, hate speech, social bots, and rumors. The hate speech problem on online social networks (OSNs) is also widespread. The existing literature has machine learning approaches for hate speech detection on OSNs. However, the effectiveness of contextual information at different orientations is understudied. This study presents a novel Convolutional, BiGRU, and Capsule network-based deep learning model, HCovBi-Caps, to classify the hate speech. The proposed model is evaluated over two Twitter-based benchmark datasets - DS1(balanced) and DS2(unbalanced) with the best performance of 0.90, 0.80, and 0.84 respectively considering precision, recall, and f-score over unbalanced dataset. In terms of training and validation accuracy, the proposed model shows the best performance of 0.93 and 0.90, respectively, over the unbalanced dataset. In comparative evaluation, HCovBi-Caps demonstrates a significantly better performance than state-of-the-art approaches. In addition, HCovBi-Caps shows comparatively better performance over the unbalanced dataset. We also investigate the impact of different hyperparameters on the efficacy of HCovBi-Caps to ascertain the selection of their values. We observed that a higher value of routing iterations adversely affects the model performance, whereas a higher value of capsule dimension improves the performance.
更多查看译文
关键词
Hate speech, Social networking (online), Deep learning, Blogs, Context modeling, Logic gates, Logistics, Hate speech detection, Twitter data analysis, convolutional layer, capsule network, BiGRU, deep learning
AI 理解论文
溯源树
样例
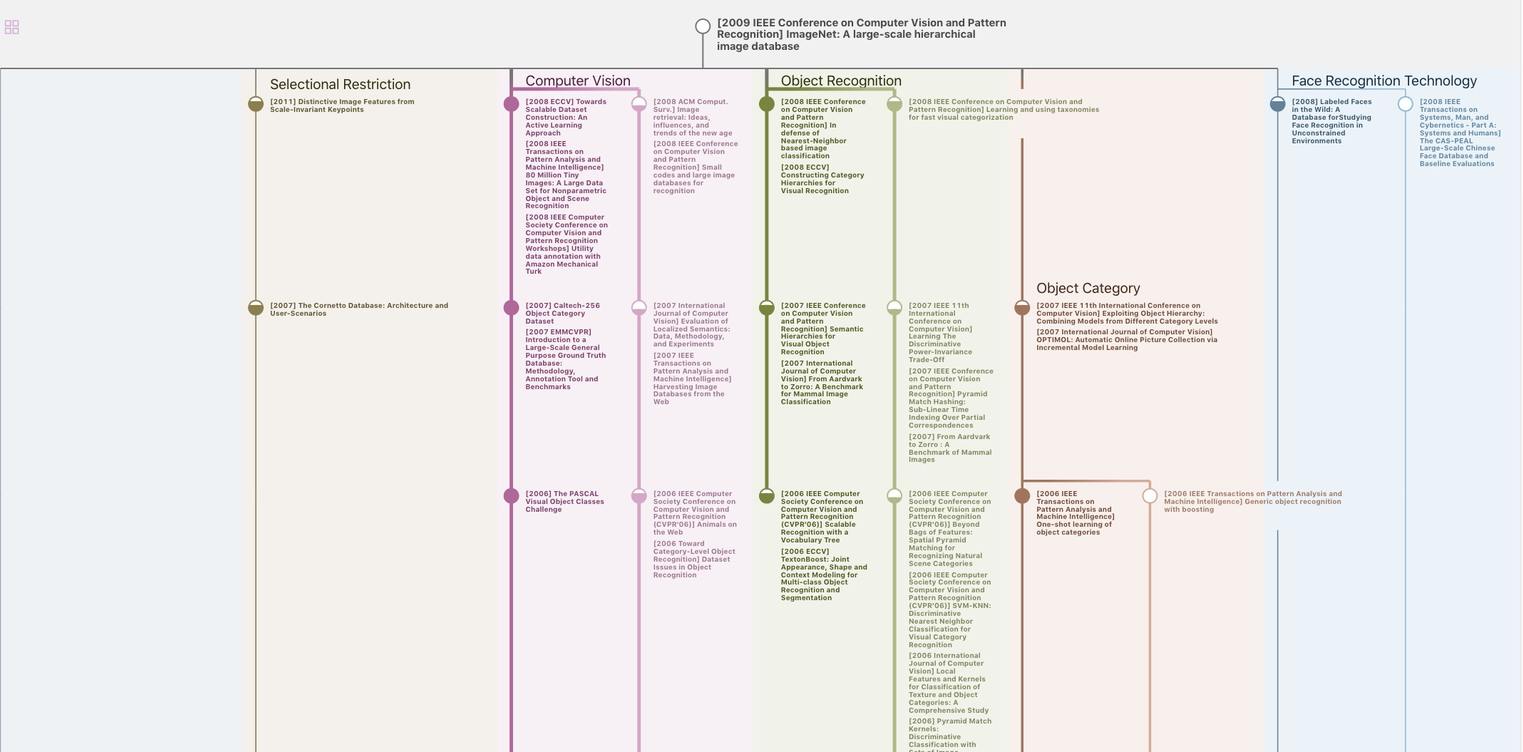
生成溯源树,研究论文发展脉络
Chat Paper
正在生成论文摘要