Time-Varying Univariate and Bivariate Frequency Analysis of Nonstationary Extreme Sea Level for New York City
ENVIRONMENTAL PROCESSES-AN INTERNATIONAL JOURNAL(2022)
摘要
Bivariate frequency analysis is an emerging method for the assessment of compound floods, especially in coastal regions. Changing climate, which usually leads to changes in characteristics of extreme hydrometeorological phenomena, makes the application of nonstationary methods more critical. In this research, a methodology is developed to apply frequency analysis on extreme sea level using physically-based hydroclimatic variables as covariates based on univariate Generalized Extreme Value (GEV) as the probability distribution function and copula methods. The results show that for extreme sea level, the location parameter of marginal distribution is directly related to the covariate variable of maximum temperature. For precipitation, the scale parameter is related to the covariate variable of minimum temperature, and the shape parameter is time-dependent. The univariate return periods of hurricanes Sandy and Irene are estimated at 85 and 12 years in nonstationary GEV distribution, respectively, while for stationary GEV distribution they are estimated at 1200 and 25 years, and in the bivariate frequency analysis of water level and precipitation, the normal copula function has more flexibility compared to other competitors. Using time-varying copula, the bivariate return periods of Hurricanes Sandy and Irene are 109 years and 136 years, respectively. The results confirm the importance of incorporating rainfall and extreme sea level in coastal flood frequency analysis. Although the proposed methodology can be applied to other hydro-climatological variables, the findings of this research suggest the necessity of considering nonstationarity in the analysis of extreme hydrologic events.
更多查看译文
关键词
Time-Varying Copula,Climate Extreme Event,Dynamic Return Period,Frequency Analysis
AI 理解论文
溯源树
样例
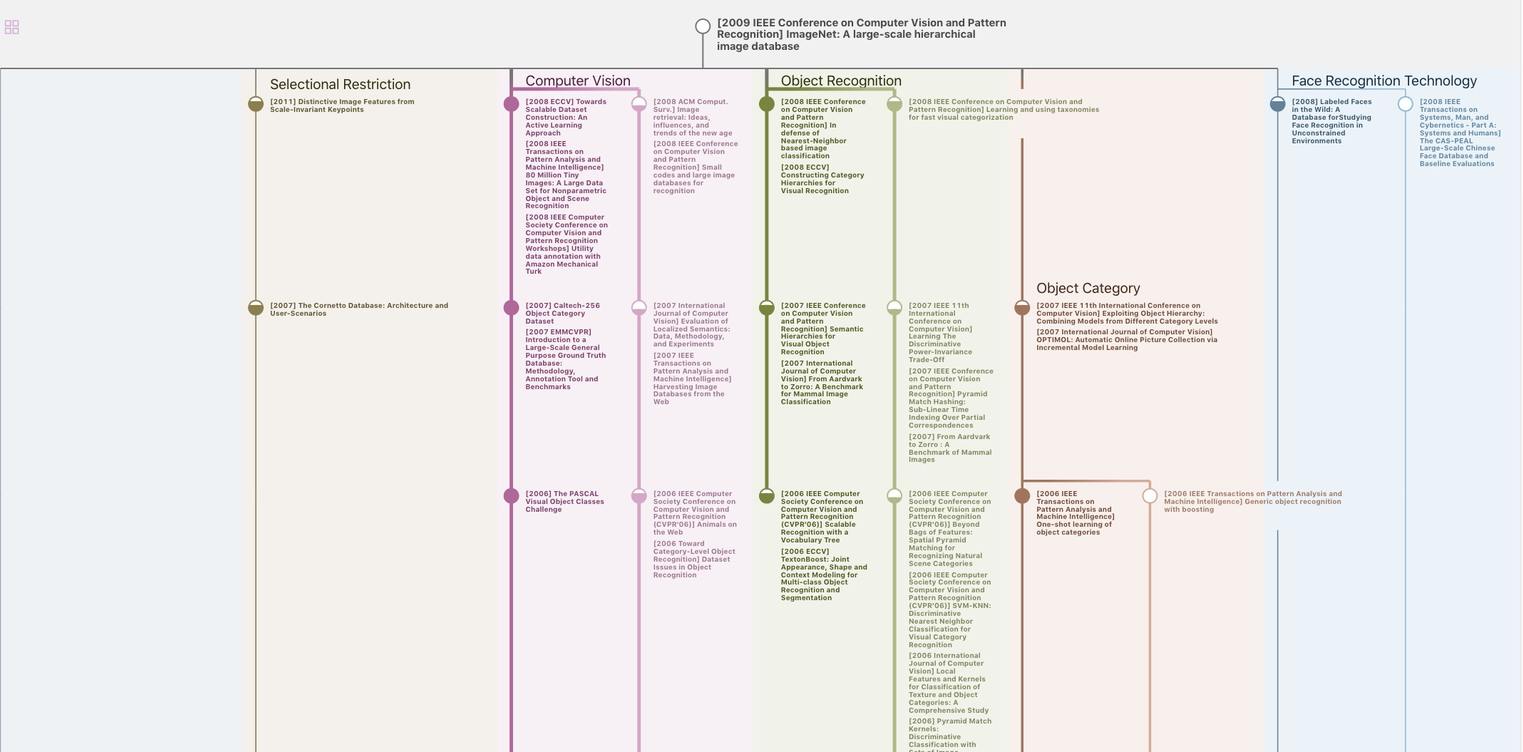
生成溯源树,研究论文发展脉络
Chat Paper
正在生成论文摘要