Pivotal discrepancy measures for Bayesian modelling of spatio-temporal data
Environmental and Ecological Statistics(2022)
摘要
Within the field of geostatistics, Gaussian processes are a staple for modelling spatial and spatio-temporal data. Statistical literature is rich with estimation methods for the mean and covariance of such processes (in both frequentist and Bayesian contexts). Considerably less attention has been paid to developing goodness-of-fit tests for assessment of model adequacy. Jun et al. (Environmetrics 25(8):584–595, 2014) introduced a statistical test that uses pivotal discrepancy measures to assess goodness-of-fit in the Bayesian context. We present a modification and generalization of their statistical test. The initial method involves spatial partitioning of the data, followed by evaluation of a pivotal discrepancy measure at each posterior draw to obtain a posterior distribution of pivotal statistics. Order statistics from this distribution are used to obtain approximate p-values. Jun et al. (Environmetrics 25(8):584–595, 2014) use arbitrary partitions based on pre-existing spatial boundaries. The partitions are made to be of equal size. Our contribution is two-fold. We use K-means clustering to create the spatial partitions and we generalise Jun et al.’s approach to incorporate unequal partition sizes. Observations from a spatial or spatio-temporal process are partitioned using an appropriate feature vector that incorporates the geographic location of the observations into subsets (not necessarily of the same size). The method’s viability is illustrated in a simulation study, and in an application to hoki ( Macruronus novaezelandiae ) catch data from a survey of the sub-Antarctic region.
更多查看译文
关键词
Bayesian models, Goodness-of-fit, Pivotal discrepancy measure, Spatio-temporal models
AI 理解论文
溯源树
样例
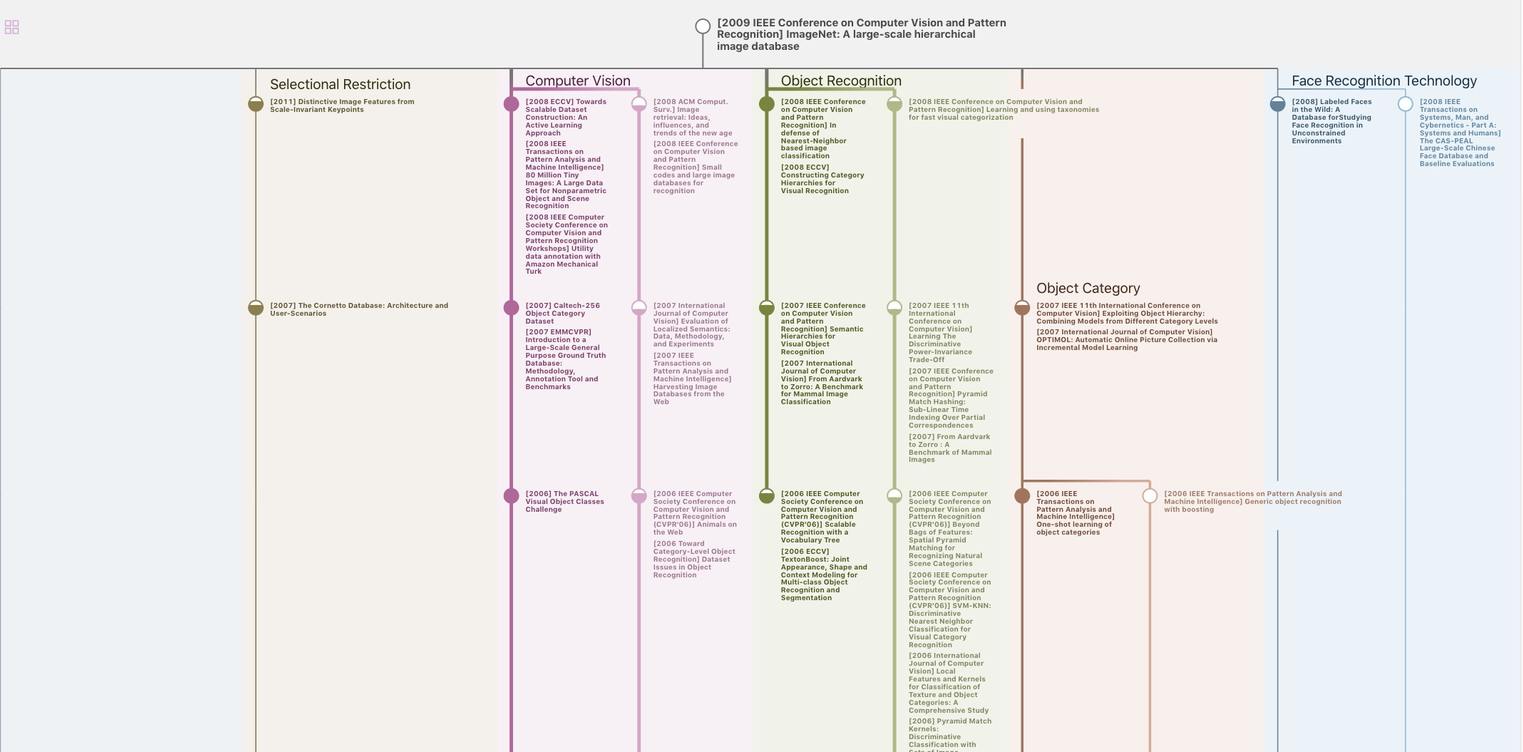
生成溯源树,研究论文发展脉络
Chat Paper
正在生成论文摘要