Using Deep Perceptual Embeddings as a Quality Metric for Synthetic Imagery
GEOSPATIAL INFORMATICS XI(2021)
摘要
The potential of synthetic image generation in machine learning applications has a myriad of both benefits and drawbacks. Synthetic datasets are valuable when real datasets are costly to acquire or difficult to share. Although synthetic imagery has improved in quality over the years, automated evaluation of the synthetic data remains challenging. For example, the Frechet inception distance does not always correlate with perceptual quality and the perceptual path length operates at the model level rather than the data level. In this work, we propose a new evaluation metric that both correlates with perceptual quality and operates at the data level so that it can function on datasets from any domain. We do this by mapping high dimensional images into a lower dimensional, perceptually disentangled embedding space and computing the distance between distributions of embeddings within this space. We utilize a convolutional autoencoder trained with a linear combination of pixelwise and perceptual losses to perform the mapping and use existing metrics to measure the distance between the distributions of embeddings. We demonstrate efficacy of this metric on the CIFAR-10 dataset.
更多查看译文
关键词
Image quality, autoencoder, perceptual loss, embedding, deep learning, quality metric
AI 理解论文
溯源树
样例
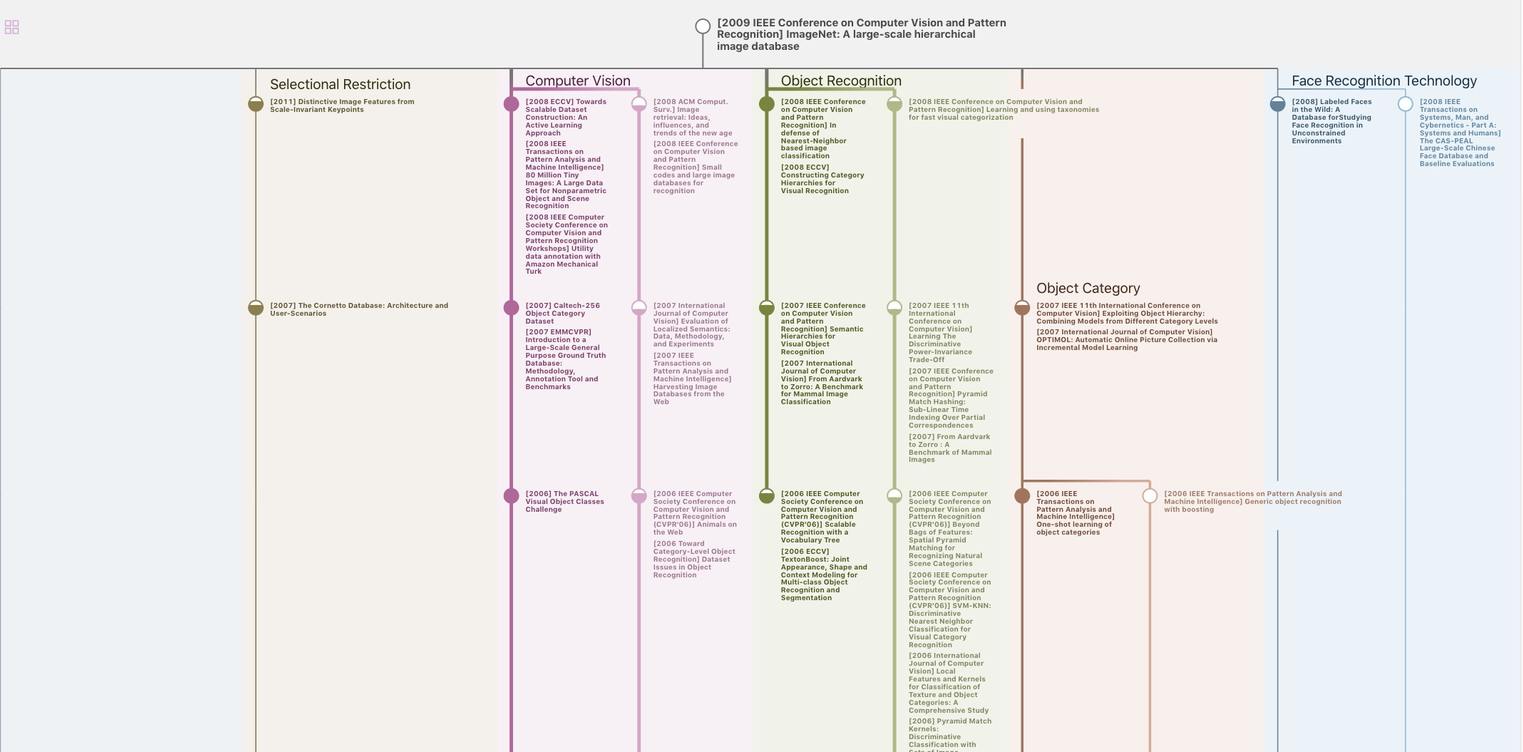
生成溯源树,研究论文发展脉络
Chat Paper
正在生成论文摘要