The Vulnerability of UAVs: An Adversarial Machine Learning Perspective
GEOSPATIAL INFORMATICS XI(2021)
摘要
The study of the vulnerabilities of unmanned aerial vehicles (UAVs) to a wide range of counter-UAV (C-UAV) attacks is well-known and established. While most C-UAV lies in the cyber, sensing, and kinetic domains, there is an emerging threat to these platforms from the perspective of adversarial machine learning (ML). Modern ML approaches are vulnerable to attacks that are largely imperceptible to humans and can be extremely successful in causing undesired false positives and false negatives in real-world scenarios. With the proliferation of ML algorithms throughout the software stack of modern UAVs, these new attacks could have real implications in the security of UAVs. A successful attack on a UAV has real-world consequences such as a collision or takeover of the platform itself. We describe a methodology for understanding the vulnerability of UAVs to these attacks by threat modeling each potential state and mode of the UAV, from powering-on, to various mission modes. In this threat modeling, we consider well-known attacks on deep learning approaches, such as state-of-the-art object detection, but also explore the possibility of novel attacks on traditional computer vision approaches, such as stereo algorithms. We examine one potential threat vector and evaluate the likelihood of success of such an attack given the current progress of adversarial ML.
更多查看译文
关键词
adversarial machine learning, counter UAV, cyber security, unmanned aerial vehicle (UAV), quadrotor
AI 理解论文
溯源树
样例
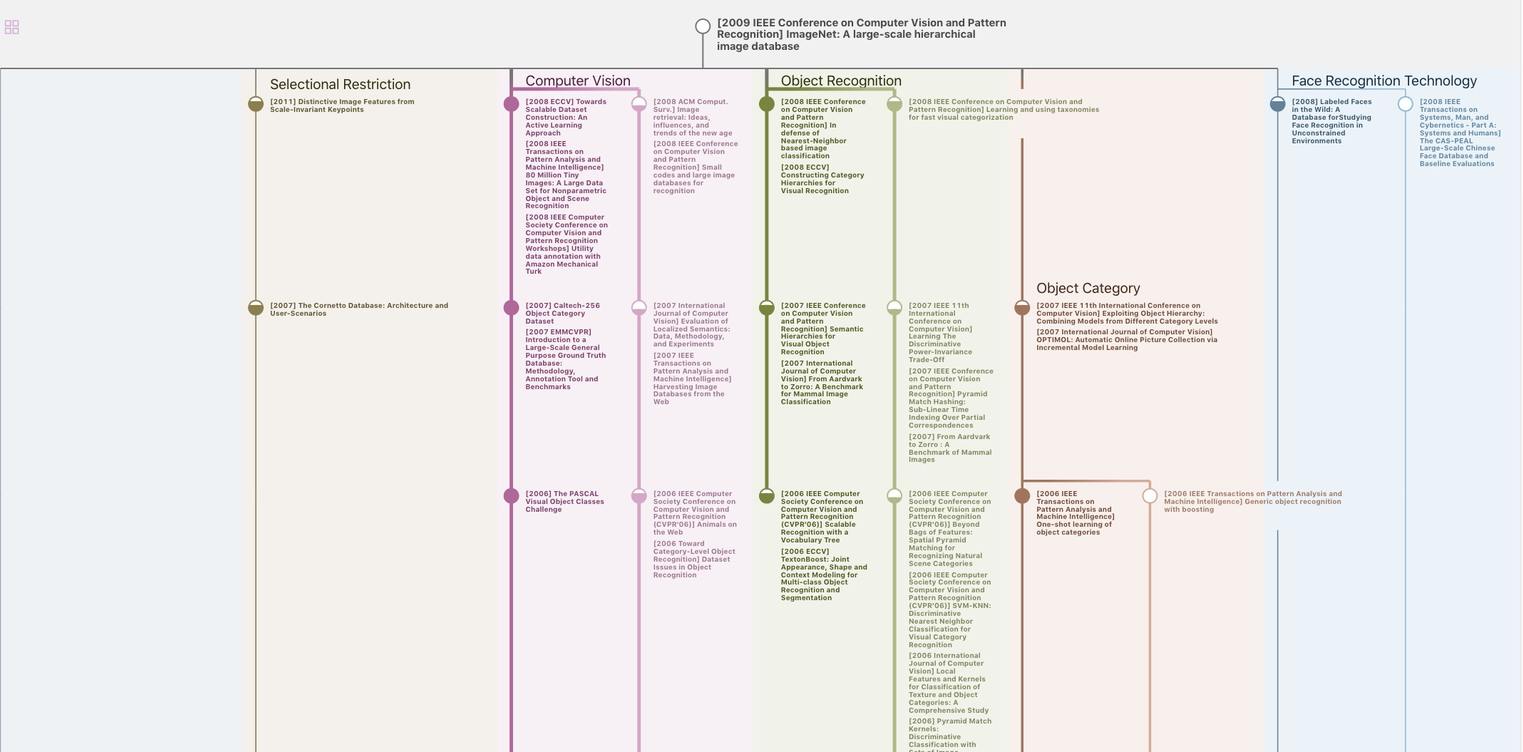
生成溯源树,研究论文发展脉络
Chat Paper
正在生成论文摘要