Active Exploration by Chance-Constrained Optimization for Voltage Regulation with Reinforcement Learning
ENERGIES(2022)
摘要
Voltage regulation in distribution networks encounters a challenge of handling uncertainties caused by the high penetration of photovoltaics (PV). This research proposes an active exploration (AE) method based on reinforcement learning (RL) to respond to the uncertainties by regulating the voltage of a distribution network with battery energy storage systems (BESS). The proposed method integrates engineering knowledge to accelerate the training process of RL. The engineering knowledge is the chance-constrained optimization. We formulate the problem in a chance-constrained optimization with a linear load flow approximation. The optimization results are used to guide the action selection of the exploration for improving training efficiency and reducing the conserveness characteristic. The comparison of methods focuses on how BESSs are used, training efficiency, and robustness under varying uncertainties and BESS sizes. We implement the proposed algorithm, a chance-constrained optimization, and a traditional Q-learning in the IEEE 13 Node Test Feeder. Our evaluation shows that the proposed AE method has a better response to the training efficiency compared to traditional Q-learning. Meanwhile, the proposed method has advantages in BESS usage in conserveness compared to the chance-constrained optimization.
更多查看译文
关键词
voltage regulation, chance-constrained optimization, reinforcement learning, uncertainties, battery, active exploration
AI 理解论文
溯源树
样例
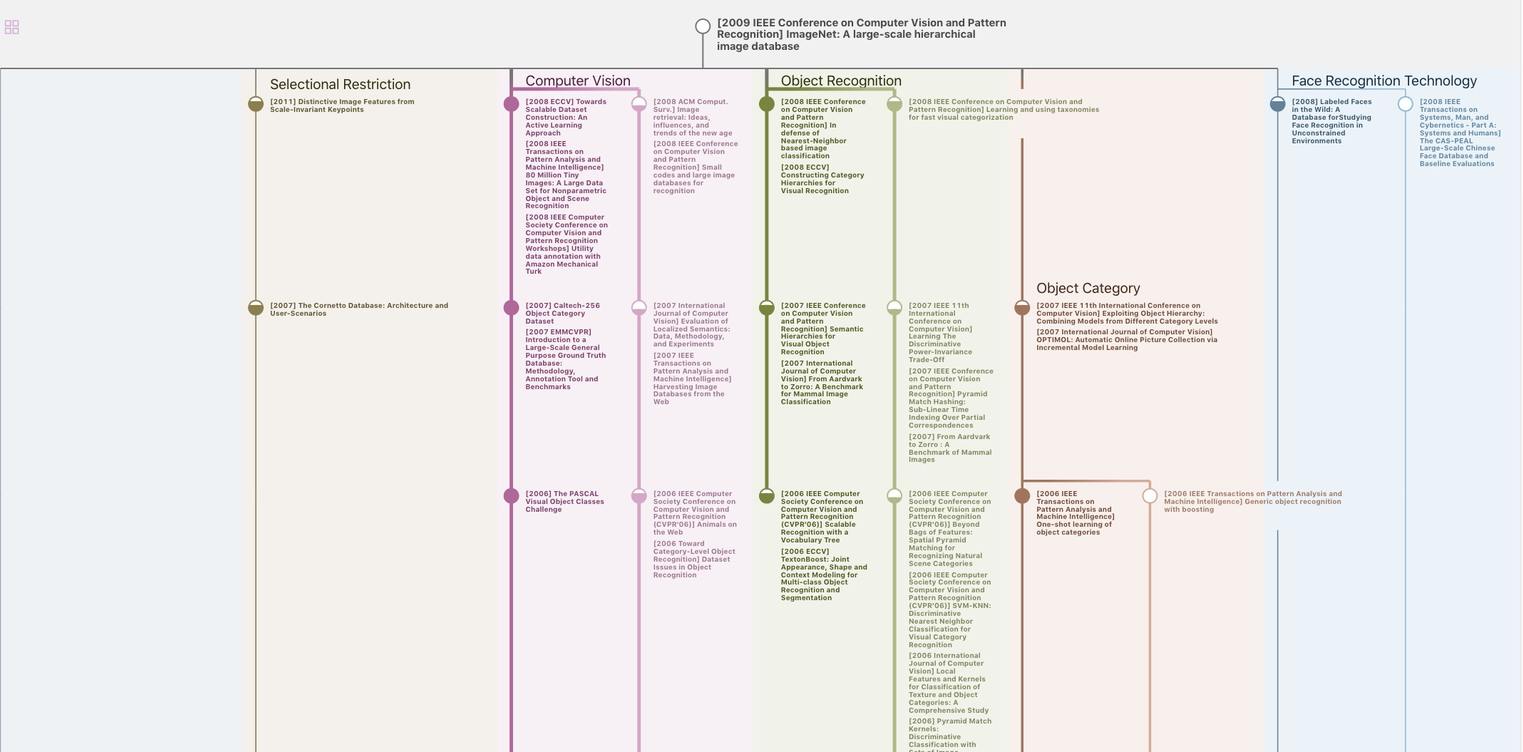
生成溯源树,研究论文发展脉络
Chat Paper
正在生成论文摘要