Chaotic diffusion-limited aggregation enhanced grey wolf optimizer: Insights, analysis, binarization, and feature selection
INTERNATIONAL JOURNAL OF INTELLIGENT SYSTEMS(2022)
摘要
Grey wolf optimization (GWO) is a widely used meta-heuristic method. It has limited searching potential when solving the majority of function optimization problems. This paper proposes a new variant of GWO, named SCGWO, which combines GWO with an improved spread strategy and a chaotic local search (CLS) mechanism to overcome these performance limitations. In detail, a spread strategy is introduced into the basic GWO to change the search agent's ability to avoid the local optima, the global exploration capability, and the individual movement's randomness. Then, a CLS mechanism is adopted to accelerate the convergence rate of the evolving agents. This method's effectiveness is illustrated by comparing the proposed SCGWO method with various algorithms, including seven GWO variants and eight well-known state-of-the-art algorithms on a comprehensive set of benchmark functions with the type of unimodal, multimodal, and composition functions. The experimental results confirmed that the established SCGWO algorithm has apparent advantages in processing unimodal, multimodal, and composition functions. Additionally, the proposed algorithm was utilized for finding the approximate optimal feature subset when applied to the feature selection problems on a set of 32 real-world data sets from the UCI machine learning repository. The results show that the binary variant also reveals a very competitive performance in dealing with feature selection. Our findings and analysis suggest that the proposed method can be a very suitable tool for realizing the optimal solutions to global optimization and wrapper-based feature selection tasks.
更多查看译文
关键词
chaotic intensification, feature selection, grey wolf optimizer, swarm intelligence
AI 理解论文
溯源树
样例
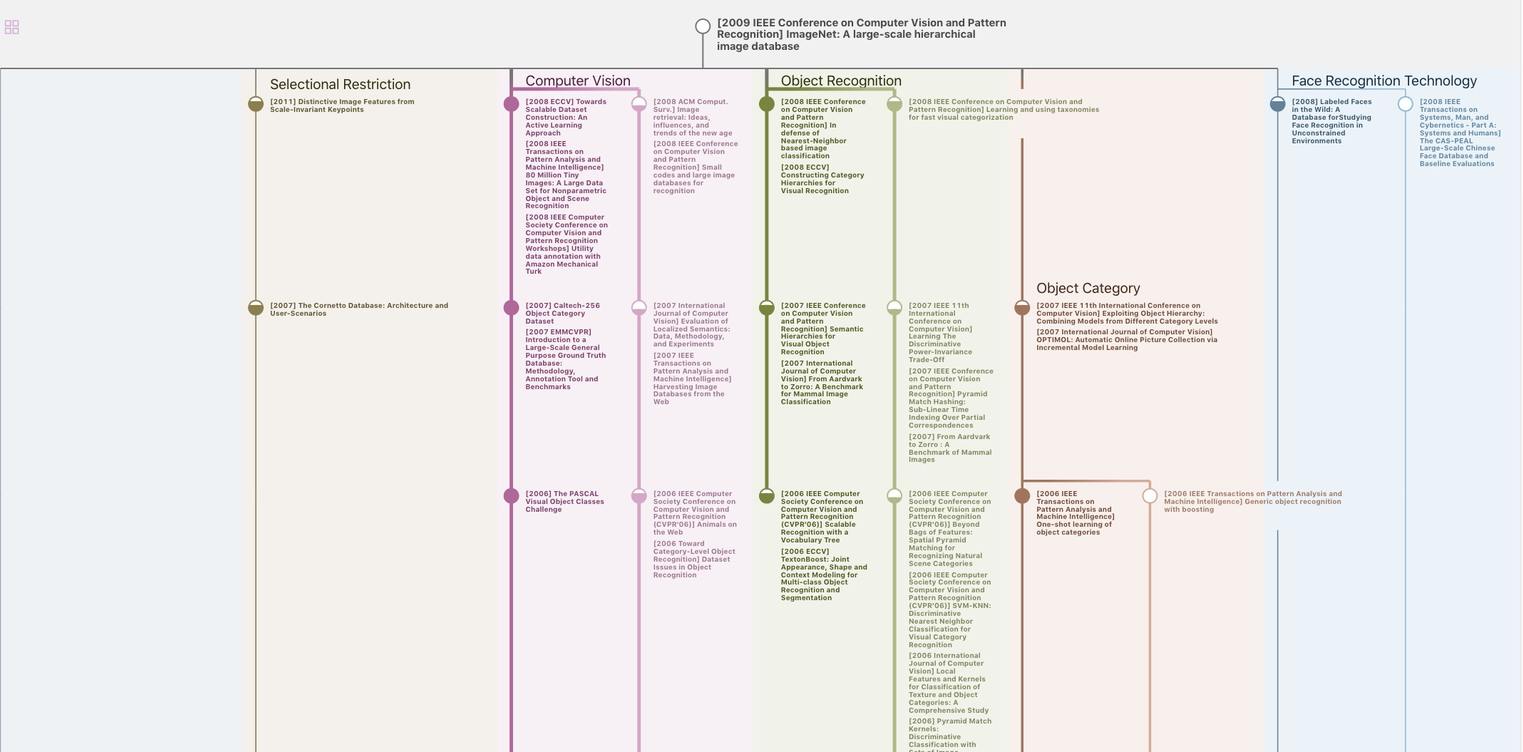
生成溯源树,研究论文发展脉络
Chat Paper
正在生成论文摘要