Detection of Adulterations in Fruit Juices Using Machine Learning Methods over FT-IR Spectroscopic Data
AGRONOMY-BASEL(2022)
Abstract
Fruit juices are one of the most adulterated beverages, usually because of the addition of water, sugars, or less expensive fruit juices. This study presents a method based on Fourier transform infrared spectroscopy (FT-IR), in combination with machine learning methods, for the correct identification and quantification of adulterants in juices. Thus, three types of 100% squeezed juices (pineapple, orange, and apple) were evaluated and adulterated with grape juice at different percentages (5%, 10%, 15%, 20%, 30%, 40%, and 50%). The results of the exploratory data analysis revealed a clear clustering trend of the samples according to the type of juice analyzed. The supervised learning analysis, based on the development of models for the detection of adulteration, obtained significant results for all tested methods (i.e., support-vector machines or SVM), random forest or RF, and linear discriminant analysis or LDA) with an accuracy above 97% on the test set. Regarding quantification, the best results are obtained with the support vector regression and with partial least square regression showing an R-2 greater than 0.99 and a root mean square error (RMSE) less than 1.4 for the test set.
MoreTranslated text
Key words
FT-IR,fruit juices,food control,machine learning,spectroscopy,regression,authentication,classification
AI Read Science
Must-Reading Tree
Example
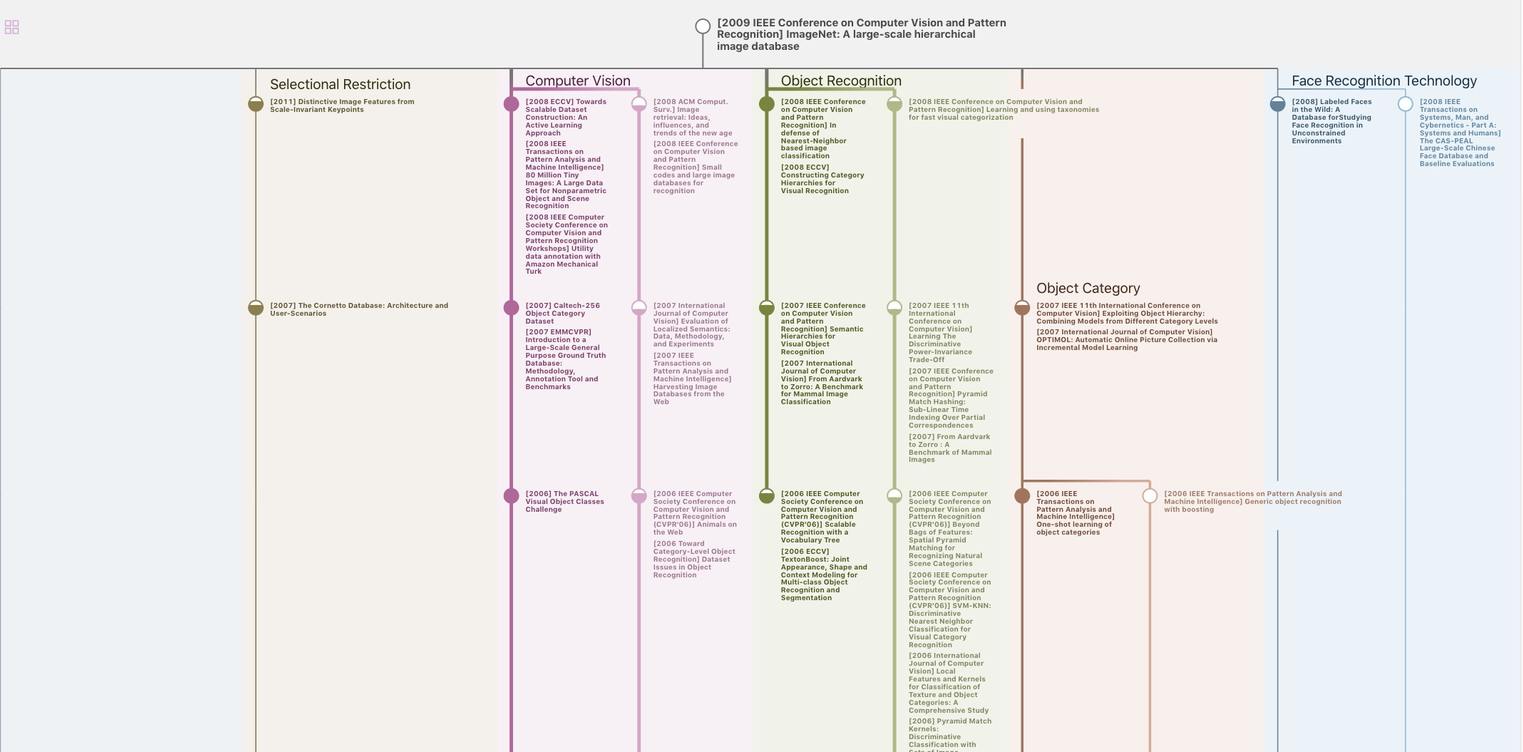
Generate MRT to find the research sequence of this paper
Chat Paper
Summary is being generated by the instructions you defined