Adaptive aggregation with self-attention network for gastrointestinal image classification
IET IMAGE PROCESSING(2022)
摘要
Automatic classification of diseases in endoscopic images is essential to the improvement of diagnostic performance and the reduction of colorectal cancer mortality. However, due to the ambiguous boundary between background and foreground, abnormal classification in endoscopic images is still challenging. To tackle such a situation, an adaptive aggregation with self-attention network (AASAN), including a global branch, a local branch, and a fusion branch, is proposed imitating the diagnosis process of endoscopists. On this basis, the self-attention with relative position encoding (SA-RPE) module is designed to capture long-range dependencies and gather lesion neighborhood information. Furthermore, an adaptive aggregation feature (AAF) module is proposed and embedded into the fusion branch for final image label prediction, which is helpful to capture more discriminant features. Extensive experiments show that the classification accuracy of the authors' method on Kvasir public dataset reaches 96.37% in a fivefold cross-validation, higher than the state-of-the-art deep learning algorithms.
更多查看译文
AI 理解论文
溯源树
样例
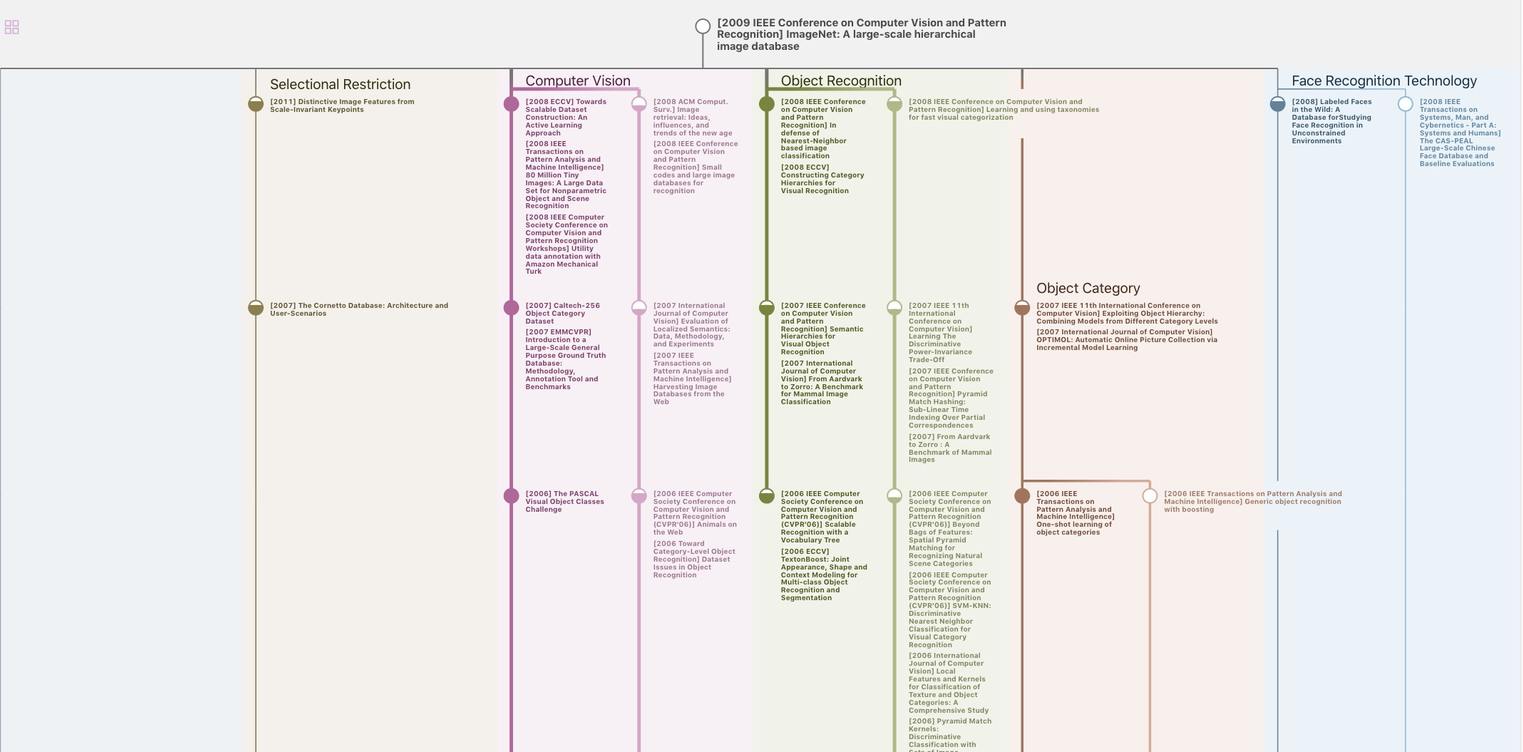
生成溯源树,研究论文发展脉络
Chat Paper
正在生成论文摘要