HMANet: Hyperbolic Manifold Aware Network for Skeleton-Based Action Recognition
IEEE Transactions on Cognitive and Developmental Systems(2023)
Abstract
Skeleton-based action recognition has attracted significant attentions in recent years. To model the skeleton data, most popular methods utilize graph convolutional networks to fuse nodes located in different parts of the graph to obtain rich geometric information. However, these methods cannot be generalized to different graph structures due to their dependencies on the input of the topological structure. In this article, we design a novel hyperbolic manifold aware network without introducing a dynamic graph. Instead, it leverages Riemannian geometry attributes of a hyperbolic manifold. Specifically, this method utilizes the Poincare model to embed the tree-like structure of the skeleton into a hyperbolic space to automatically capture hierarchical features, which may explore the underlying manifold of the data. To extract spatio-temporal features in the network, the features in manifold space are projected to a tangent space, and a tangent space features translation method based on the Levi-Civita connection was proposed. In addition, we introduce the geometric knowledge of Riemannian manifolds to further explain how features are transformed in the tangent space. Finally, we conduct experiments on several 3-D skeleton data sets with different structures, successfully verifying the effectiveness and advancement of the proposed method.
MoreTranslated text
Key words
Manifolds,Skeleton,Feature extraction,Convolutional neural networks,Geometry,Data models,Videos,Action recognition,hyperbolic manifold,Poincare model,Riemannian geometry,spatio-temporal features
AI Read Science
Must-Reading Tree
Example
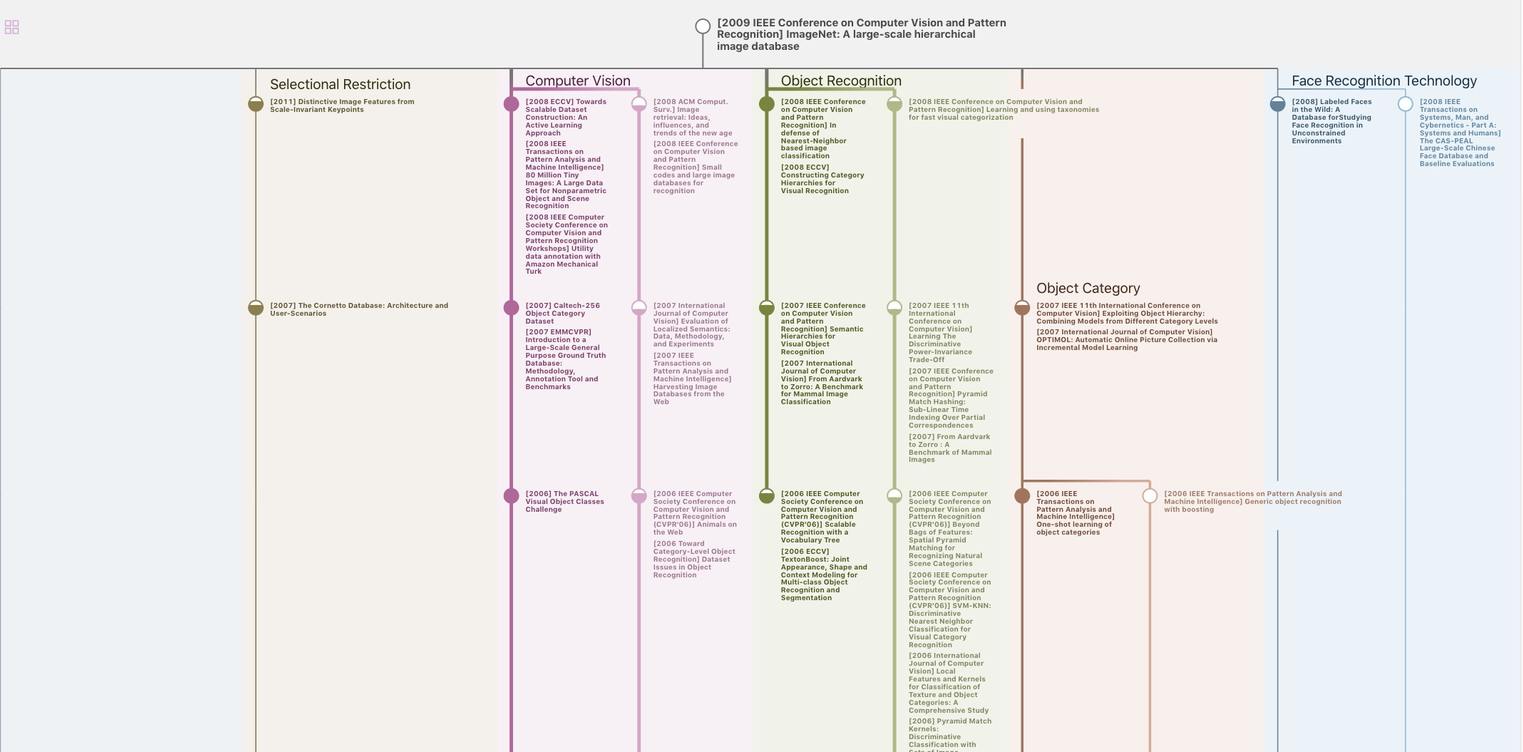
Generate MRT to find the research sequence of this paper
Chat Paper
Summary is being generated by the instructions you defined