Weighted composite asymmetric Huber estimation for partial functional linear models
AIMS MATHEMATICS(2022)
摘要
In this paper, we first investigate a new asymmetric Huber regression (AHR) estimation procedure to analyze skewed data with partial functional linear models. To automatically reflect distributional features as well as bound the influence of outliers effectively, we further propose a weighted composite asymmetric Huber regression (WCAHR) estimation procedure by combining the strength across multiple asymmetric Huber loss functions. The slope function and constant coefficients are estimated through minimizing the combined loss function and approximating the slope function with principal component analysis. The asymptotic properties of the proposed estimators are derived. To realize the WCAHR estimation, we also develop a practical algorithm based on pseudo data. Numerical results show that the proposed WCAHR estimators can well adapt to the different error distributions, and thus are more useful in practice. Two real data examples are presented to illustrate the applications of the proposed methods.
更多查看译文
关键词
functional principal component analysis, partial functional linear model, asymmetric Huber regression, weighted composite asymmetric Huber regression, functional data analysis
AI 理解论文
溯源树
样例
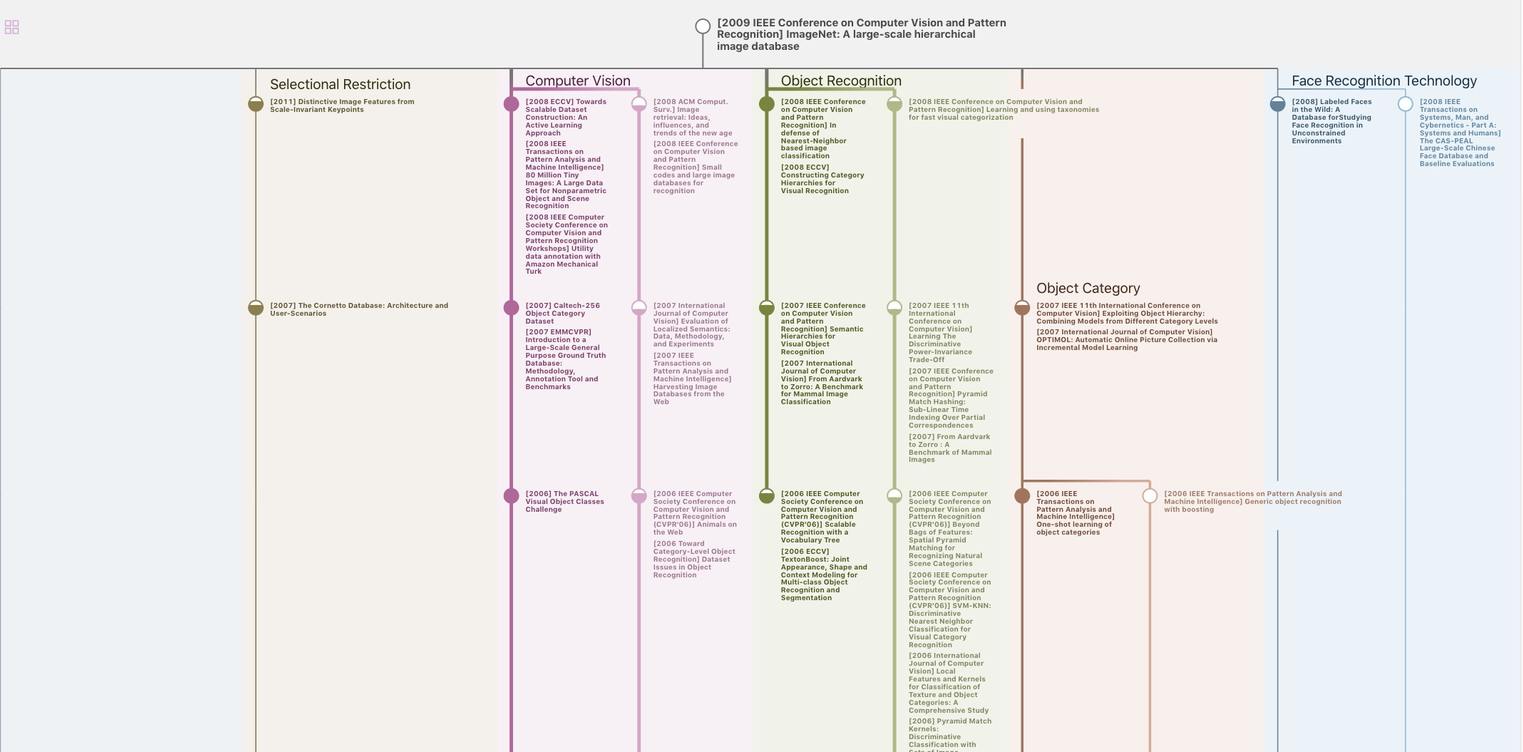
生成溯源树,研究论文发展脉络
Chat Paper
正在生成论文摘要