Quality estimation method for gear hobbing based on attention and adversarial transfer learning
Measurement(2022)
Abstract
Because the gear inspection process is time-consuming and the equipment wears easily, gear machining and inspection data collection are costly; therefore, it is difficult to collect sufficient gear machining data for deep learning training. To solve this problem, this study proposes an attention and adversarial transfer learning method based on ResNet with adaptive Coral loss (A(2)ResNet-aCoral) for training small-sample datasets. The method integrates sample-spatial attention and dynamic-channel attention to enhance the feature extraction ability. To realize the transfer ability, an adversarial domain structure is constructed to induce the network to extract the homogeneous features of the source and target domains. Moreover, an adaptive correlation alignment loss is proposed to shorten the distance between the source and target domains. The results of a case study indicate that A(2)ResNet-aCoral has good transfer ability on small sample gear hobbing signals, and the accuracy can reach 92.71%.
MoreTranslated text
Key words
Transfer learning,Gear hobbing,Quality estimate,Attention mechanism,Domain adversarial
AI Read Science
Must-Reading Tree
Example
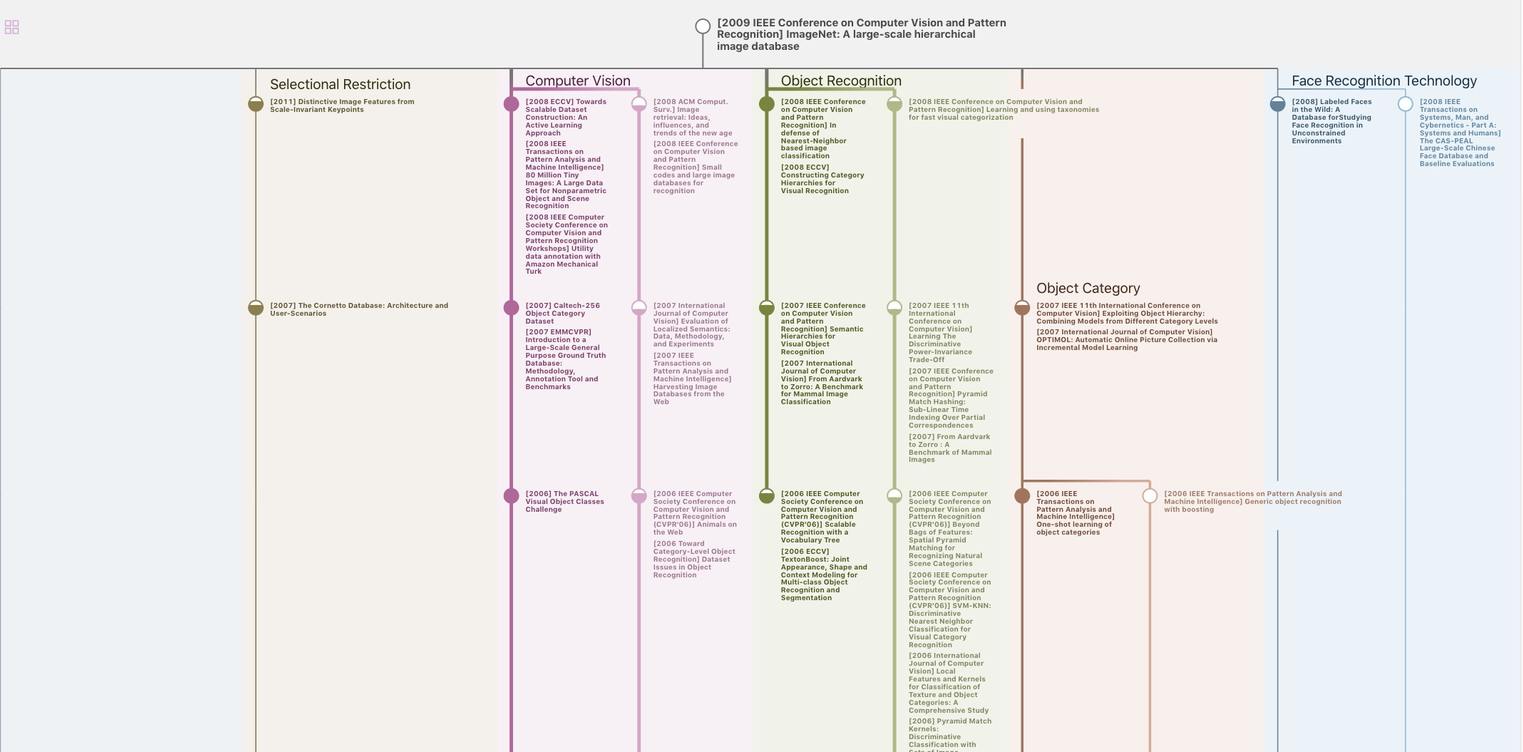
Generate MRT to find the research sequence of this paper
Chat Paper
Summary is being generated by the instructions you defined