Adaptive regularization minimization algorithms with nonsmooth norms
IMA JOURNAL OF NUMERICAL ANALYSIS(2023)
摘要
An adaptive regularization algorithm (AR1pGN) for unconstrained nonlinear minimization is considered, which uses a model consisting of a Taylor expansion of arbitrary degree and regularization term involving a possibly nonsmooth norm. It is shown that the nonsmoothness of the norm does not affect the O(epsilon(-(p+1)/p)(1)) upper bound on evaluation complexity for finding first-order epsilon(1)-approximate minimizers using p derivatives, and that this result does not hinge on the equivalence of norms in Rn. It is also shown that, if p = 2, the bound of O(epsilon(-3)(2)) evaluations for finding second-order epsilon(2)-approximate minimizers still holds for a variant of AR1pGN named AR2GN, despite the possibly nonsmooth nature of the regularization term. Moreover, the adaptation of the existing theory for handling the nonsmoothness results is an interesting modification of the subproblem termination rules, leading to an even more compact complexity analysis. In particular, it is shown when the Newton's step is acceptable for an adaptive regularization method. The approximate minimization of quadratic polynomials regularized with nonsmooth norms is then discussed, and a new approximate second-order necessary optimality condition is derived for this case. A specialized algorithm is then proposed to enforce first- and second-order conditions that are strong enough to ensure the existence of a suitable step in AR1pGN (when p = 2) and in AR2GN, and its iteration complexity is analyzed. A final section discusses how practical approximate curvature measures may lead to weaker second-order optimality guarantees.
更多查看译文
关键词
nonlinear optimization,adaptive regularization,evaluation complexity,nonsmooth norms,second-order minimizers
AI 理解论文
溯源树
样例
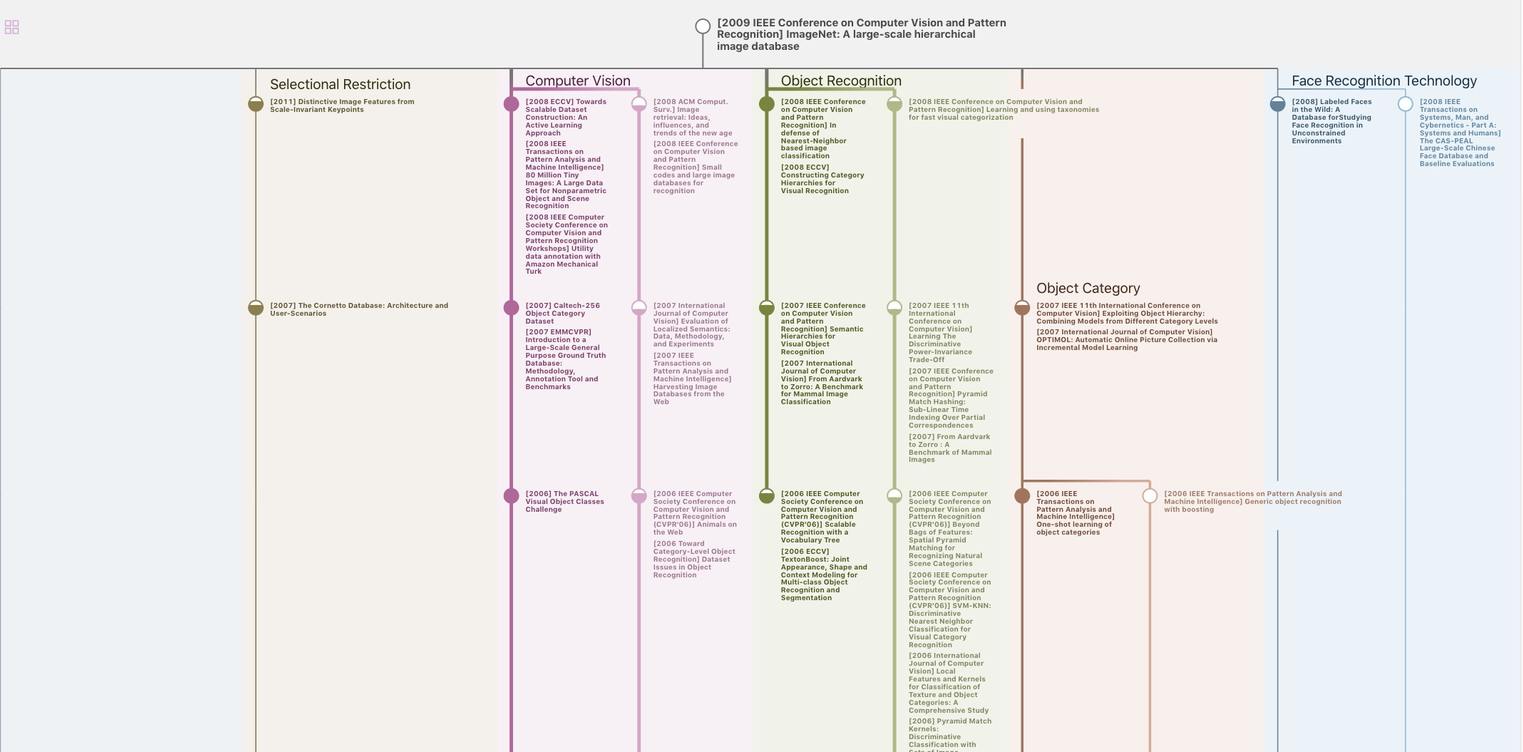
生成溯源树,研究论文发展脉络
Chat Paper
正在生成论文摘要