Further investigation of 3D dose verification in proton therapy utilizing acoustic signal, wavelet decomposition and machine learning
BIOMEDICAL PHYSICS & ENGINEERING EXPRESS(2022)
摘要
Online dose verification in proton therapy is a critical task for quality assurance. We further studied the feasibility of using a wavelet-based machine learning framework to accomplishing that goal in three dimensions, built upon our previous work in 1D. The wavelet decomposition was utilized to extract features of acoustic signals and a bidirectional long-short-term memory (Bi-LSTM) recurrent neural network (RNN) was used. The 3D dose distributions of mono-energetic proton beams (multiple beam energies) inside a 3D CT phantom, were generated using Monte-Carlo simulation. The 3D propagation of acoustic signal was modeled using the k-Wave toolbox. Three different beamlets (i.e. acoustic pathways) were tested, one with its own model. The performance was quantitatively evaluated in terms of mean relative error (MRE) of dose distribution and positioning error of Bragg peak (Delta(BP)), for two signal-to-noise ratios (SNRs). Due to the lack of experimental data for the time being, two SNR conditions were modeled (SNR = 1 and 5). The model is found to yield good accuracy and noise immunity for all three beamlets. The results exhibit an MRE below 0.6% (without noise) and 1.2% (SNR = 5), and Delta(BP) below 1.2 mm (without noise) and 1.3 mm (SNR = 5). For the worst-case scenario (SNR = 1), the MRE and Delta(BP) are below 2.3% and 1.9 mm, respectively. It is encouraging to find out that our model is able to identify the correlation between acoustic waveforms and dose distributions in 3D heterogeneous tissues, as in the 1D case. The work lays a good foundation for us to advance the study and fully validate the feasibility with experimental results.
更多查看译文
关键词
proton therapy,dose verification,acoustic signal,machine learning,wavelet
AI 理解论文
溯源树
样例
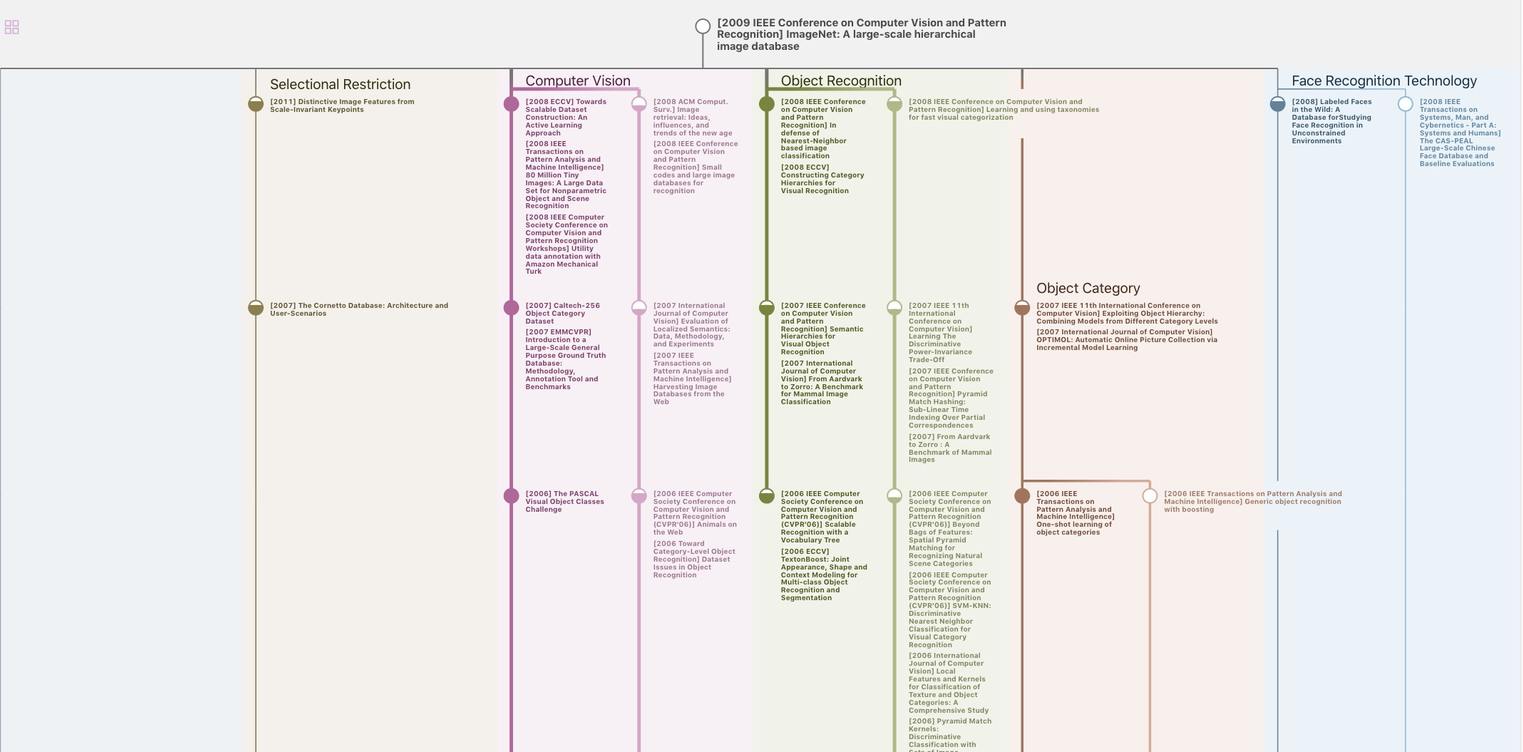
生成溯源树,研究论文发展脉络
Chat Paper
正在生成论文摘要