A Blockchain-Reinforced Federated Intrusion Detection Architecture for IIoT
IEEE Internet of Things Journal(2024)
Abstract
Federated Learning (FL) in Industrial IoT (IIoT) facilitates collaborative model training across distributed edge devices, ensuring data privacy and localized insights without centralized data aggregation. However, the networked parameter sharing mechanism in FL renders it vulnerable to exploitation by Man-in-the-Middle (MITM) attackers, potentially disrupting the model training process. To mitigate this threat, this paper presents a novel blockchain-reinforced federated learning architecture aimed at enabling cooperative intrusion detection. Initially, federated learning is leveraged to aggregate all learned information from edge servers, thereby disseminating extracted attack characteristics to all participants through gradient sharing. Subsequently, a blockchain-based parameter verification scheme is introduced to safeguard against tampered local parameters affecting the global model. Clients record model parameters in smart contracts deployed on a private chain, and parameter servers verify parameter confidentiality before aggregation, ensuring only valid parameters are considered. Finally, extensive experiments are conducted using an edge IIoT cybersecurity dataset comprising 61 features spanning 10 protocol layers and five attacks targeting IIoT connectivity protocols. Simulation results demonstrate that the proposed scheme significantly enhances intrusion detection accuracy, achieving a threefold improvement when two-thirds of federated nodes are subjected to MITM attacks.
MoreTranslated text
Key words
IIoT,IIoT security,federated learning,blockchain,smart contract
AI Read Science
Must-Reading Tree
Example
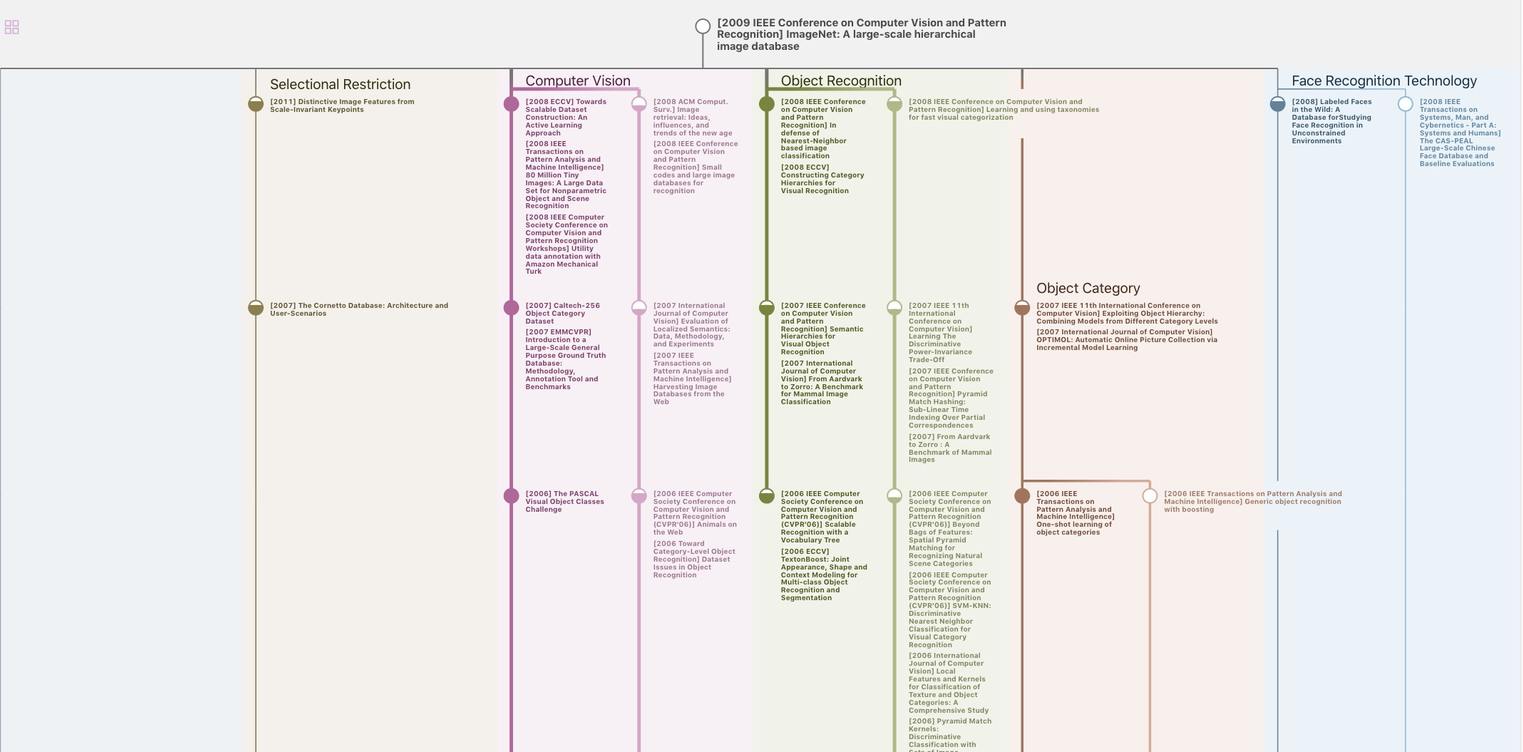
Generate MRT to find the research sequence of this paper
Chat Paper
Summary is being generated by the instructions you defined