Probabilistic Spatio-Temporal Fusion of Affordances for Grasping and Manipulation
IEEE ROBOTICS AND AUTOMATION LETTERS(2022)
摘要
Robust vision-based grasping and manipulation of unknown objects in unstructured scenes requires the extraction of action candidates based on visual information while taking into account noise and occlusions in such scenes. We address this problem by combining the concept of affordances and Bayesian Recursive State Estimation. We propose to extract affordances using heuristics on the averaged local surface information of supervoxels in a point cloud. Based on a local, geometry-aware coordinate frame, we define a uniform state for different affordances. Using Bayesian statistics, this state is fused across multiple observations of the scene to improve the estimates for the pose and existence certainty of actions. This facilitates the extraction of robust grasping and manipulation actions independent of the segmentation of a scene. The proposed approach is evaluated in grasping experiments with more than 900 grasp executions using the humanoid robot ARMAR-6 in an unstructured scene with a variable number of unknown objects. The experimental results show that the grasping success rate is improved by over 10% compared to a state-of-the-art approach.
更多查看译文
关键词
Perception for grasping and manipulation, probabilistic inference, semantic scene understanding
AI 理解论文
溯源树
样例
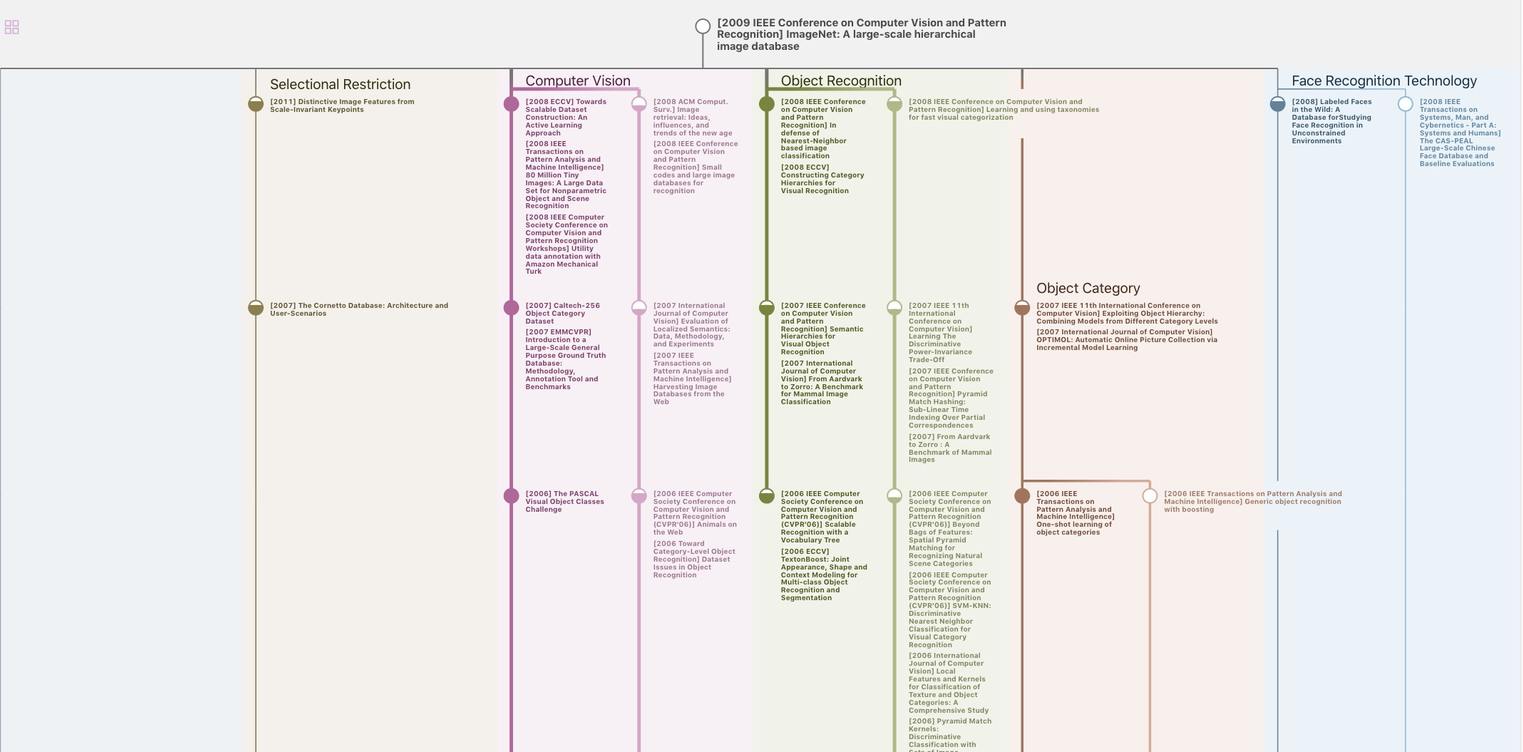
生成溯源树,研究论文发展脉络
Chat Paper
正在生成论文摘要