Contact State Estimation for Peg-in-Hole Assembly Using Gaussian Mixture Model
IEEE ROBOTICS AND AUTOMATION LETTERS(2022)
摘要
Recently, the robotic assembly has been expanded into an unstructured environment. This environment includes uncertainties that may cause unexpected situations such as a failure of the assembly.Such problems can be prevented or monitored by a robust contact state (CS) estimation method. In that sense, the paper suggests a CS estimation method that contains a torque indicator, a position/velocity' indicator, and a CS discriminator. Using joint torque of manipulators and position/velocity of the end-effector, a Gaussian Mixture Model (GMM) builds each indicator by reflecting on two properties of measured data, i.e., non-stationary behavior and correlation among the data. The indicators play a role to indicate the corresponding sensor state. The discriminator is defined by rules which combine the results of the indicators, allowing a robust CS estimation to he achieved. In this respect, the proposed method has a distinct advantage over existing distance-based clustering methods which ignore probabilistic properties or correlation among measured data. The performance of the estimation is demonstrated through experiments with torque-controlled manipulators and commercial prefabricated furniture.
更多查看译文
关键词
Assembly, contact modeling, perception for grasping and manipulation
AI 理解论文
溯源树
样例
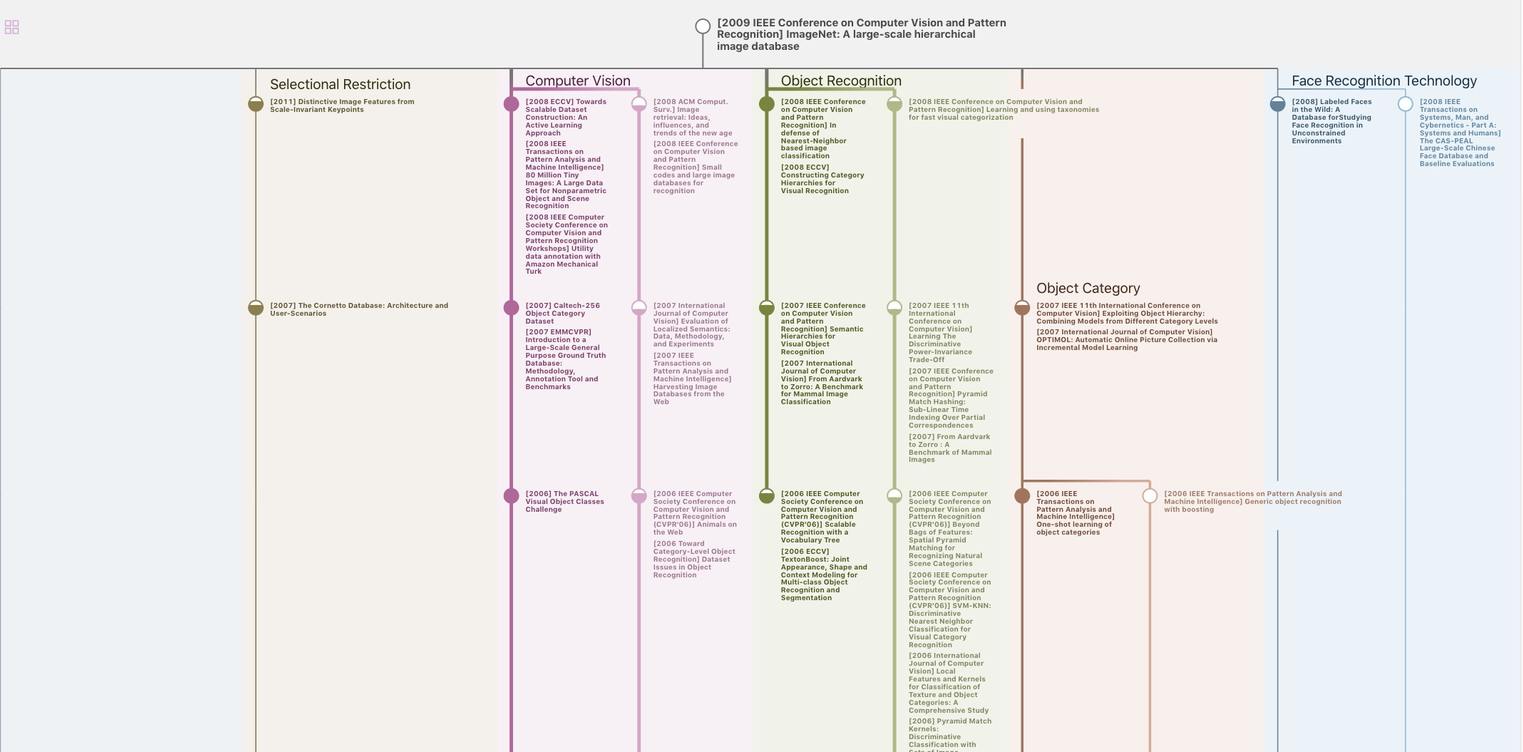
生成溯源树,研究论文发展脉络
Chat Paper
正在生成论文摘要